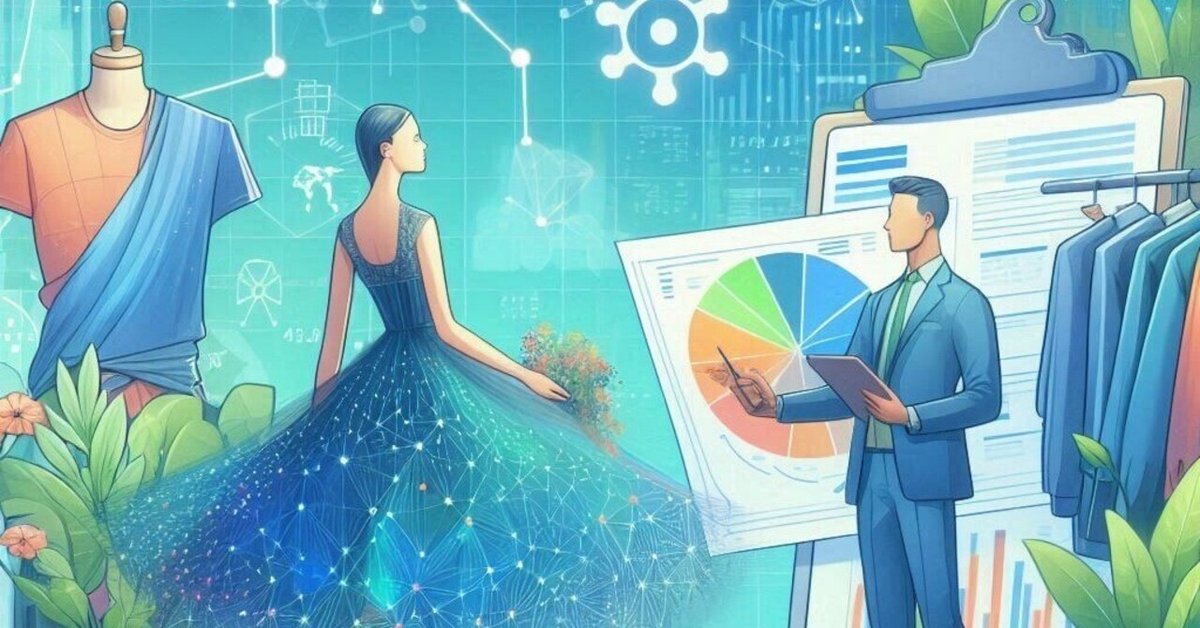
持続可能なファッションとデータサイエンス:産業分類別分析と月別統計
持続可能なファッションとデータサイエンス:産業分類別分析と月別統計
#SustainableFashion #DataScience #サプライチェーン最適化 #消費者行動分析 #持続可能性追跡 #素材革新 #ライフサイクル評価 #中古市場分析 #透明性 #リアルタイムフィードバック #産業分析
データサイエンスと持続可能なファッションの関係
データサイエンスは、持続可能なファッションを実現する上で不可欠なツールです。膨大なデータを活用することで、ファッション業界は環境に優しく、社会的に責任あるアプローチを取ることが可能になります。以下に、その主な関係性をまとめました。
サプライチェーンの最適化: データサイエンスは、サプライチェーン全体を効率化し、資源の無駄を削減します。これにより、環境への負荷が軽減されます。#サプライチェーン最適化
消費者行動分析: 消費者の購買パターンをデータ分析で理解し、過剰生産を防ぎます。これにより、適切な量の製品が市場に供給されます。#消費者行動分析
持続可能性追跡: ブランドが環境への影響を追跡し、持続可能な選択を行うための指標を提供します。#持続可能性追跡
素材革新: データサイエンスは、持続可能な素材の開発と普及を促進します。#素材革新
ライフサイクル評価: 製品のライフサイクル全体を評価し、環境への影響を最小限にするための改善点を特定します。#ライフサイクル評価
パーソナライズとオンデマンド生産: データに基づくパーソナライズにより、大量生産の必要性を減らし、資源の無駄を抑えます。#パーソナライズ #オンデマンド生産
中古市場分析: 中古市場のトレンドを分析し、衣服の再利用とリサイクルを促進します。#中古市場分析
透明性と追跡可能性: サプライチェーンの透明性を高め、倫理的な調達を確保します。#透明性 #追跡可能性
リアルタイムフィードバック: リアルタイムのデータ分析で迅速に持続可能な実践に対応します。#リアルタイムフィードバック
産業分類ごとの分析と協力の可能性
衣食住関連の事業者の売上、消費行動、企業の決算書などを分析することは、持続可能な方法を探る上で非常に重要です。以下のステップで分析を進めます。
データ収集と分析: 市場調査データや企業の財務データ、消費者アンケートを収集し分析します。#データ分析
データの統合: 売上や成長率、収益構造などを比較し、関連性のある情報を統合します。#データ統合
地域事業者の特定: 地元の事業者を特定し、協力の可能性を探ります。#地域事業者
協力のポテンシャルの評価: 大企業と地域事業者の協力の可能性を評価し、サプライチェーンの効率化や地元生産の促進を図ります。#協力ポテンシャル
パイロットプロジェクトの開始: 小規模なプロジェクトで協力の実現可能性と利点を検証します。#パイロットプロジェクト
持続可能性の視点: 環境への影響を考慮し、持続可能な協力プロジェクトを設計します。#持続可能性
地域コミュニティとの連携: 地域のニーズを理解し、協力を推進するためのコミュニケーションを確立します。#地域コミュニティ
成果のモニタリング: プロジェクトの進捗と成果をモニタリングし、必要に応じて改善を行います。#成果モニタリング
月別および季節ごとの統計データの収集
市場トレンドを理解し、戦略を立てるためには、月別および季節ごとの統計データが不可欠です。
データソースの特定: 政府機関や業界団体など、信頼性のあるデータソースを特定します。#データソース
データ収集: 月別および季節ごとの売上、消費行動、生産量などのデータを収集します。#データ収集
データの整理: 不正確なデータをクリーニングし、分析用のデータセットを準備します。#データ整理
データ分析: 統計手法やデータ可視化ツールを使って、月別および季節ごとのトレンドを特定します。#データ分析
トレンドの理解: 分析結果から、月別および季節ごとの変動要因を理解します。#トレンド理解
戦略の策定: データを活用して、プロモーションやキャンペーンの戦略を計画し実行します。#戦略策定
モニタリングと調整: データを定期的にモニタリングし、戦略を適切に調整します。#モニタリング
持続可能なファッションにおけるデータサイエンスの活用は、多くの可能性を秘めています。統計データの分析や産業分類ごとの協力は、持続可能な未来を実現するための重要なステップです。
Data Science and Sustainable Fashion: Industry Classification Analysis and Monthly Statistics
#SustainableFashion #DataScience #SupplyChainOptimization #ConsumerBehaviorAnalysis #SustainabilityTracking #MaterialInnovation #LifeCycleAssessment #SecondhandMarketAnalysis #Transparency #RealTimeFeedback #IndustryAnalysis
The Relationship Between Data Science and Sustainable Fashion
Data science is an indispensable tool in achieving sustainable fashion. By leveraging vast amounts of data, the fashion industry can adopt approaches that are more environmentally friendly and socially responsible. Here's an overview of the key relationships:
Supply Chain Optimization: Data science optimizes the entire supply chain, reducing waste and minimizing environmental impact. #SupplyChainOptimization
Consumer Behavior Analysis: By analyzing consumer purchasing patterns, data analysis helps prevent overproduction, ensuring an appropriate amount of products are supplied to the market. #ConsumerBehaviorAnalysis
Sustainability Tracking: Provides indicators to track environmental impact, assisting brands in making sustainable choices. #SustainabilityTracking
Material Innovation: Promotes the development and use of sustainable materials through data science. #MaterialInnovation
Life Cycle Assessment: Evaluates the entire product life cycle to identify areas for improvement and minimize environmental impact. #LifeCycleAssessment
Personalization and On-Demand Production: Reduces the need for mass production by offering personalized products based on data insights, thus minimizing resource waste. #Personalization #OnDemandProduction
Secondhand Market Analysis: Analyzes trends in the secondhand market, promoting the reuse and recycling of clothing. #SecondhandMarketAnalysis
Transparency and Traceability: Enhances supply chain transparency, ensuring ethical sourcing. #Transparency #Traceability
Real-Time Feedback: Allows for quick adaptation to sustainable practices through real-time data analysis. #RealTimeFeedback
Industry Classification Analysis and Potential Cooperation
Analyzing sales, consumer behavior, and financial statements of businesses related to food, clothing, and shelter is crucial in exploring sustainable methods. The following steps outline the analysis process:
Data Collection and Analysis: Collect and analyze market research data, corporate financial data, and consumer surveys. #DataAnalysis
Data Integration: Compare and integrate related information such as sales, growth rates, and revenue structures. #DataIntegration
Identification of Local Businesses: Identify local businesses and explore cooperation opportunities. #LocalBusinesses
Evaluation of Cooperation Potential: Assess the potential for cooperation between large corporations and local businesses to streamline supply chains and promote local production. #CooperationPotential
Pilot Project Launch: Test the feasibility and benefits of cooperation with small-scale pilot projects. #PilotProject
Sustainability Perspective: Design sustainable cooperation projects, considering environmental impact. #Sustainability
Collaboration with Local Communities: Understand local needs and establish communication to promote cooperation. #LocalCommunity
Monitoring of Outcomes: Monitor project progress and outcomes, making improvements as necessary. #OutcomeMonitoring
Collection of Monthly and Seasonal Statistical Data
Understanding market trends and developing strategies require monthly and seasonal statistical data.
Identification of Data Sources: Identify reliable data sources such as government agencies and industry organizations. #DataSources
Data Collection: Collect monthly and seasonal data on sales, consumer behavior, and production volume. #DataCollection
Data Cleaning: Clean inaccurate data and prepare datasets for analysis. #DataCleaning
Data Analysis: Use statistical methods and data visualization tools to identify monthly and seasonal trends. #DataAnalysis
Understanding Trends: Understand the factors influencing monthly and seasonal fluctuations from the analysis results. #TrendUnderstanding
Strategy Formulation: Utilize the data to plan and execute strategies for promotions and campaigns. #StrategyFormulation
Monitoring and Adjustment: Regularly monitor the data and adjust strategies accordingly. #Monitoring
The application of data science in sustainable fashion holds vast potential. Analyzing statistical data and fostering cooperation in industry classifications are crucial steps toward realizing a sustainable future.
*************************************************************:
世界地図
聖書
それぞれの自分のイエス
みんなのイエス
福音 十字架
憲法
取材の自由
知る権利
生活保護法
読み替え規定
社会福祉関係法
自然科学論文
演繹法 帰納法
デ-タ改竄
ブルムバ-グ
日本製鉄 イタリアとアメリカで企業買収
中国 住宅市場低迷
EC 好材料なし
World Map
Bible
Each person's Jesus
Everyone's Jesus
Gospel, Cross
Constitution
Freedom of the press
Right to know
Public Assistance Act
Reinterpretation provisions
Social welfare-related laws
Natural Science Papers
Deductive method, Inductive method
Data falsification
Bloomberg
Nippon Steel acquires companies in Italy and the United States
China, Housing market downturn
EU, No good news
#Bible #Gospel #FreedomOfThePress #RightToKnow #PublicAssistanceAct #SocialWelfare #NaturalScience #DeductiveMethod #InductiveMethod #DataFalsification #NipponSteel #ChinaHousingMarket #EU #Bloomberg