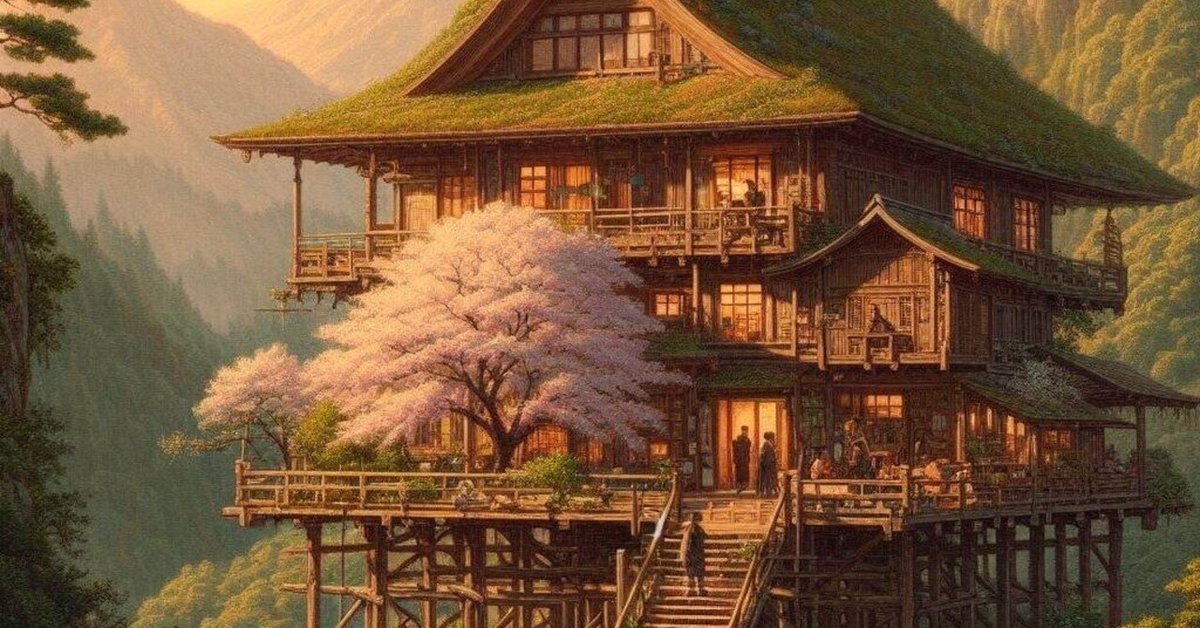
衣食住の季節ごとの特徴を分析する
次への道筋
四半期の有価証券報告書とビッグデータを活用して衣食住の季節ごとの特徴を分析することは、企業や地域経済に対する深い洞察を得るために非常に効果的です。特に、ビッグデータのリソースやその具体的な活用手法に関する詳細な理解が重要です。今回は、ビッグデータの具体的なリソースや、それらを活用するための根拠サイトやプラットフォームに焦点を当て、さらに詳しく説明します。
有価証券報告書のデータ抽出
衣食住関連企業の四半期有価証券報告書は、財務状況や市場動向を理解するための基盤的なデータソースです。財務データ、収益、在庫状況、経営者コメントなどが重要な指標となりますが、これらを補完するためにビッグデータを活用することで、より深い洞察が得られます。ビッグデータの収集元と根拠サイト
ビッグデータの収集には、複数のデータソースを活用し、それらを統合して分析します。具体的には、次のようなリソースと根拠サイトが考えられます:
a. ソーシャルメディアデータ
ソーシャルメディアは、消費者のリアルタイムの反応や季節ごとのトレンドを把握するための重要なデータソースです。以下のプラットフォームやAPIがデータ収集に活用されます:
Twitter API (developer.twitter.com):リアルタイムでトレンドやハッシュタグ、地域別の消費者の声を収集し、感情分析やトレンド分析に利用。
Instagram Graph API (developers.facebook.com):消費者のビジュアル投稿データやハッシュタグ分析により、ファッションや食品トレンドを視覚的に捉えることが可能。
Google Trends (trends.google.com):季節ごとの検索トレンドを把握し、特定の商品やテーマに対する消費者の関心がいつ高まるかを予測。
b. 購買データ
購買データは、消費者が実際にどのような商品をどのタイミングで購入しているかを反映します。オンラインショッピングプラットフォームやPOSシステムから取得可能です:
Amazon Web Services (AWS) Marketplace (aws.amazon.com/marketplace):購買履歴データや消費者行動に関するデータセットを提供しており、季節ごとの商品売上データを解析することができます。
Shopify API (shopify.dev):Eコマースデータを収集し、購買パターンやシーズンごとの売れ筋商品の分析に利用。
POSデータ分析(例:Square、Cloverなど):実店舗での購入履歴を収集し、リアルタイムの売上や商品カテゴリー別の消費動向を追跡。
c. 気候データ
気候データは、衣食住に密接に関わるため、季節ごとの需要予測に不可欠な要素です。次のようなプラットフォームから気候データを収集します:
気象庁データ(Japan Meteorological Agency) (jma.go.jp):日本国内の詳細な気象データを収集し、特定の地域や季節における気温や降水量のトレンドを分析。
OpenWeather API (openweathermap.org):世界中の気象データを取得し、季節ごとの天気パターンや気候に応じた消費者行動を予測可能。
NOAA (National Oceanic and Atmospheric Administration) (noaa.gov):アメリカの気候データを取得し、グローバルな気候変動に基づく衣食住の市場予測に役立てます。
d. モバイル位置情報データ
消費者の移動パターンを解析することで、特定の季節や地域ごとの行動変化を捉えることができます。次のようなプラットフォームからモバイル位置データを取得できます:
Foursquare API (developer.foursquare.com):消費者の訪問傾向やロケーションベースのデータを分析し、どの場所でどの季節に消費が活発かを把握します。
Google Maps API (developers.google.com/maps):消費者の移動経路や特定エリアでの活動データを活用して、消費行動の地域性や季節性を分析。
e. 小売業者からの売上データ
リアルタイムの売上データを提供するプラットフォームを活用して、製品のカテゴリーごとに季節的な売上動向を分析します。
Nielsen Retail Measurement (nielsen.com):小売業界全体の売上データを収集し、季節ごとの消費トレンドを分析します。
IRI(Information Resources, Inc.) (iriworldwide.com):食品や日用品を中心としたリアルタイムの販売データを収集し、季節ごとの売上の増減を把握します。
3. 季節ごとの特徴を特定する
ビッグデータを用いて、衣食住に関する企業パフォーマンスや消費者行動の季節ごとの特徴を明確に特定することができます。各データソースから得られた情報を組み合わせることで、各シーズンにおける消費トレンドや売上の変動を予測し、以下のように分析を深めます。
衣(ファッション業界):
ソーシャルメディアや購買データを組み合わせて、特定の季節におけるファッショントレンドや、消費者の関心がどのタイミングでピークに達するかを予測。
利用可能なデータソース:Instagram Graph API、Google Trends
食(食品業界):
気候データと購買データを統合して、季節性食材や外食産業の季節ごとの動向を予測。
利用可能なデータソース:OpenWeather API、Amazon Web Services Marketplace
住(住宅関連業界):
気候や移動データを活用して、季節に応じた住宅リフォームや引っ越しシーズンの需要を予測。
利用可能なデータソース:NOAA、Foursquare API
4. データ分析手法と活用方法
ビッグデータの豊富なリソースを活用した高度なデータ分析手法を用いることで、季節ごとの消費パターンをより正確に理解できます。これには、以下のような手法が含まれます:
時系列解析:AWS MarketplaceやGoogle Trendsのデータを用いて、消費動向を時系列で分析し、季節性を特定します。
機械学習モデル:Amazon Web ServicesやShopifyからの購買データを基に、季節ごとの需要予測モデルを構築し、次の四半期の需要を予測。
クラスター分析:Nielsen Retail Measurementのデータを使用し、消費者セグメントごとの購買傾向を分析し、シーズンごとの消費パターンをクラスター化。
データビジュアライゼーション:TableauやPower BIを使用して、データを視覚化し、季節ごとの消費動向を視覚的に把握できるようにします。
まとめ
ビッグデータのリソースと根拠サイトを活用することで、衣食住の季節ごとの消費パターンや企業のパフォーマンスを深く分析し、戦略的な意思決定をサポートします。ソーシャルメディアデータや購買データ、気候データ、モバイル位置情報データ、小売業者の売上データなど、多様なデータソースを統合することで、より精度の高い分析が可能です。
#ビッグデータ #衣食住 #有価証券報告書 #データ分析 #ソーシャルメディアデータ #購買データ #気候データ #時系列解析 #機械学習モデル #データビジュアライゼーション #地域経済Analyzing the seasonal characteristics of the clothing, food, and housing industries using quarterly securities reports and big data can be highly effective in gaining deep insights into companies and regional economies. It is particularly important to have a detailed understanding of big data resources and how to utilize them effectively. In this post, we will focus on specific big data resources, relevant platforms, and foundational sites for utilizing them, providing a detailed explanation.
Extracting Data from Securities Reports
Quarterly securities reports from clothing, food, and housing-related companies are foundational data sources for understanding financial conditions and market trends. Key indicators include financial data, revenues, inventory levels, and management comments. However, supplementing these with big data can provide even deeper insights.Sources and Foundational Sites for Big Data
Big data collection involves leveraging multiple data sources, integrating them, and conducting analyses. Specifically, the following resources and foundational sites are relevant:
a. Social Media Data
Social media is an important data source for understanding real-time consumer reactions and seasonal trends. The following platforms and APIs are useful for data collection:
Twitter API (developer.twitter.com): Collects real-time trends, hashtags, and consumer voices by region, enabling sentiment and trend analysis.
Instagram Graph API (developers.facebook.com): Analyzes visual posts and hashtags, allowing for a deeper understanding of fashion and food trends.
Google Trends (trends.google.com): Tracks seasonal search trends, predicting when consumer interest in specific products or themes peaks.
b. Purchase Data
Purchase data reflects the timing and types of products consumers are buying. This data is obtainable from online shopping platforms and POS systems:
Amazon Web Services (AWS) Marketplace (aws.amazon.com/marketplace): Provides datasets on purchasing history and consumer behavior, allowing for seasonal sales data analysis.
Shopify API (shopify.dev): Collects e-commerce data, analyzing purchase patterns and best-selling products by season.
POS Data Analysis (e.g., Square, Clover): Tracks in-store purchase history, real-time sales, and category-specific consumer trends.
c. Climate Data
Climate data is closely related to the clothing, food, and housing industries, making it indispensable for seasonal demand forecasting. The following platforms provide climate data:
Japan Meteorological Agency Data (jma.go.jp): Collects detailed climate data in Japan, analyzing trends in temperature and precipitation by region and season.
OpenWeather API (openweathermap.org): Acquires global weather data, predicting consumer behavior based on seasonal weather patterns.
NOAA (National Oceanic and Atmospheric Administration) (noaa.gov): Provides U.S. climate data, contributing to global market forecasting in the clothing, food, and housing sectors based on climate changes.
d. Mobile Location Data
By analyzing consumer movement patterns, mobile location data captures behavioral changes by season and region. The following platforms provide mobile location data:
Foursquare API (developer.foursquare.com): Analyzes consumer visit trends and location-based data to identify where and when consumption is most active.
Google Maps API (developers.google.com/maps): Analyzes consumer travel routes and activities in specific areas, revealing seasonal and regional consumption trends.
e. Retailer Sales Data
Real-time sales data platforms can be used to analyze seasonal sales trends by product category:
Nielsen Retail Measurement (nielsen.com): Collects comprehensive retail sales data, analyzing seasonal consumer trends.
IRI (Information Resources, Inc.) (iriworldwide.com): Provides real-time sales data, particularly for food and daily necessities, capturing seasonal sales fluctuations.
3. Identifying Seasonal Characteristics
Big data can help clearly identify seasonal characteristics in company performance and consumer behavior related to clothing, food, and housing. By integrating information from various data sources, it is possible to predict seasonal consumption trends and sales fluctuations, and further deepen the analysis as follows:
Clothing (Fashion Industry):
By combining social media and purchase data, it is possible to predict fashion trends for specific seasons and pinpoint when consumer interest peaks.
Available Data Sources: Instagram Graph API, Google Trends
Food (Food Industry):
By integrating climate and purchase data, it is possible to predict trends for seasonal ingredients and dining-out industries.
Available Data Sources: OpenWeather API, Amazon Web Services Marketplace
Housing (Housing Industry):
By utilizing climate and movement data, it is possible to forecast demand for home renovations or peak moving seasons based on seasonal changes.
Available Data Sources: NOAA, Foursquare API
4. Data Analysis Techniques and Applications
Utilizing rich big data resources enables a more accurate understanding of seasonal consumption patterns. The following techniques can be applied:
Time Series Analysis: Analyze time-series data from AWS Marketplace and Google Trends to identify seasonal trends in consumer behavior.
Machine Learning Models: Build seasonal demand forecasting models using purchase data from Amazon Web Services and Shopify, predicting demand for the next quarter.
Cluster Analysis: Use Nielsen Retail Measurement data to analyze purchasing trends by consumer segment, identifying seasonal consumption patterns by clustering consumers.
Data Visualization: Tools like Tableau and Power BI can be used to visually display data, making it easier to understand seasonal consumption trends.
Conclusion
Leveraging big data resources and foundational sites enables deep analysis of seasonal consumption patterns and corporate performance in the clothing, food, and housing sectors, supporting strategic decision-making. By integrating diverse data sources—social media data, purchase data, climate data, mobile location data, and retailer sales data—more accurate analysis becomes possible.
#BigData #ClothingFoodHousing #SecuritiesReports #DataAnalysis #SocialMediaData #PurchaseData #ClimateData #TimeSeriesAnalysis #MachineLearningModels #DataVisualization #RegionalEconomy