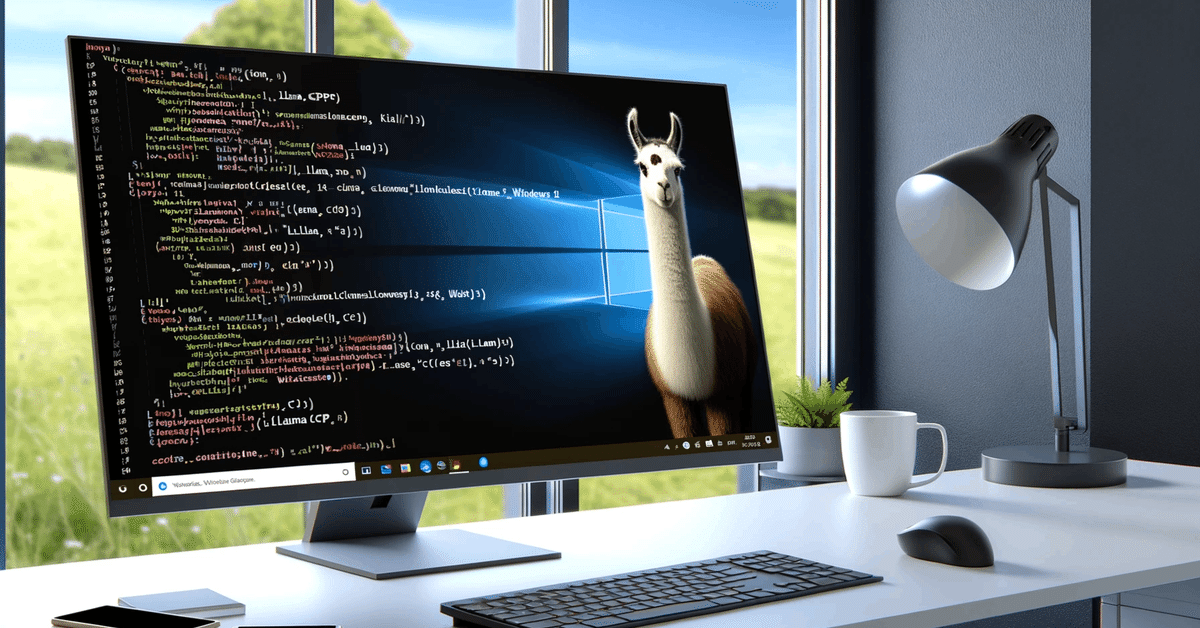
【ローカルLLM】Windows10のllama.cppでGPUを使う
はじめに
前回、ローカルLLMを使う環境構築として、Windows 10でllama.cppを使えるようにしました。
私のPCはGeForce RTX3060を積んでいるのですが、素直にビルドしただけではCPUを使った生成しかできないようなので、GPUを使えるようにして高速化を図ります。
以下環境です。
OS:Windows10 64bit
CPU:AMD Ryzen 5 3600
GPU:GeForce RTX 3060
llama.cppのGitHubを見ると、BLAS Buildの項目にそれらしい記載がありました。
CUDA、BLAS、cuBLAS
恥ずかしながら、「CUDA」がGPUに関する言葉であることぐらいしかわからなかったので、ChatGPTさんに聞いてみました。
まずはCUDAとは何か。
CUDA(Compute Unified Device Architecture)は、NVIDIAによって開発された、GPU(Graphics Processing Unit)を使用して並列計算を行うためのプログラミングモデルおよびコンピューティングプラットフォームです。CUDAを使用することで、デベロッパーはC、C++、Fortranなどの言語で書かれたプログラムを通じて、グラフィックス処理よりも幅広い種類の計算タスクを高速に実行できるようになります。
CUDAは特に科学計算、工学的なシミュレーション、データ分析、機械学習などの分野で有効であり、GPUの計算能力を活用して、これらの計算集約的なタスクをCPUだけで実行する場合と比べて大幅に高速化することができます。CUDAアーキテクチャを利用することで、開発者は並列処理のための直感的なアプローチを取り、複雑な並列アルゴリズムを簡単に実装できるようになります。
本来画像処理を行うためのGPUを、普通の計算もできるようにするアーキテクチャ、と言うことですね。
次に、BLASです。
BLAS(Basic Linear Algebra Subprograms)は、基本的な線形代数演算を高速に行うためのライブラリとAPIの集合です。ベクトルや行列の加算、スカラー乗算、ベクトル乗算、行列乗算など、線形代数の基本的な演算を効率的に実行するためのルーチンが含まれています。BLASは数値計算の分野で広く利用されており、科学技術計算、工学、統計解析、機械学習などのアプリケーションの基盤となっています。
BLASの実装は多数存在し、それぞれ異なるハードウェアアーキテクチャに最適化されています。例えば、IntelのMath Kernel Library (MKL) やAMDのCore Math Library (ACML)、NVIDIAのcuBLAS(CUDAを用いたBLASの実装)などがあります。これらの最適化された実装により、高性能計算環境での計算効率が大幅に向上します。
BLAS自体は線形代数演算のためのライブラリであって、GPUに直接関係するわけではないようです。
BLASをCUDAのアーキテクチャ向けに最適化し、計算タスクを(NVIDIAの)GPU上で高速に動かせるようにしたものが、cuBLASと言う事のようです。
という事でGPUを使うために必要なのは、cuBLASに対応できるようにllama.cppをビルドしなおすことのようです。
Githubの記述を追って、作業を進めます。
CUDA Toolkitの確認
まずはCUDA Toolkitがインストールされているか確認します。
下記コマンドを実行してます。
nvcc --version
コマンドが認識されなければ、インストールされていないか、環境変数が設定されていないということらしいです。インストールをした記憶はないので、もちろんコマンドも認識されず。ということで、CUDA Toolkitをインストールしていきます。
下記にアクセスします。
自分の環境に合うものを選んで行って、インストーラをダウンロードします。
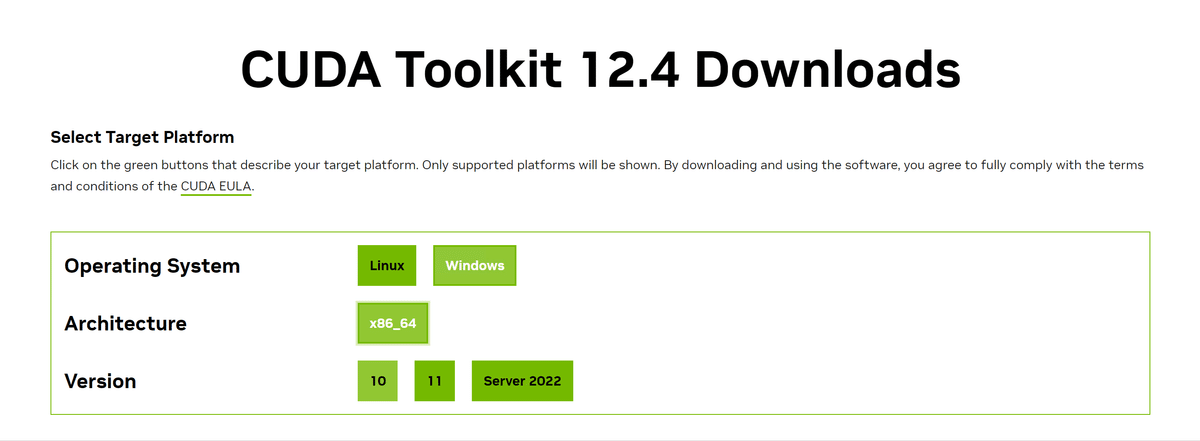
ダウンロードできたらファイルを実行し、インストールを進めます。
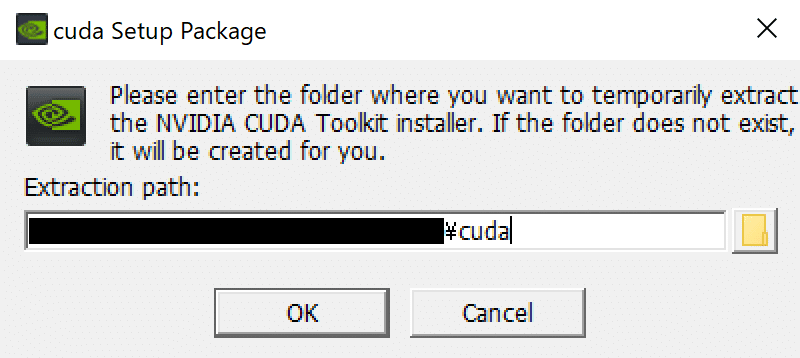
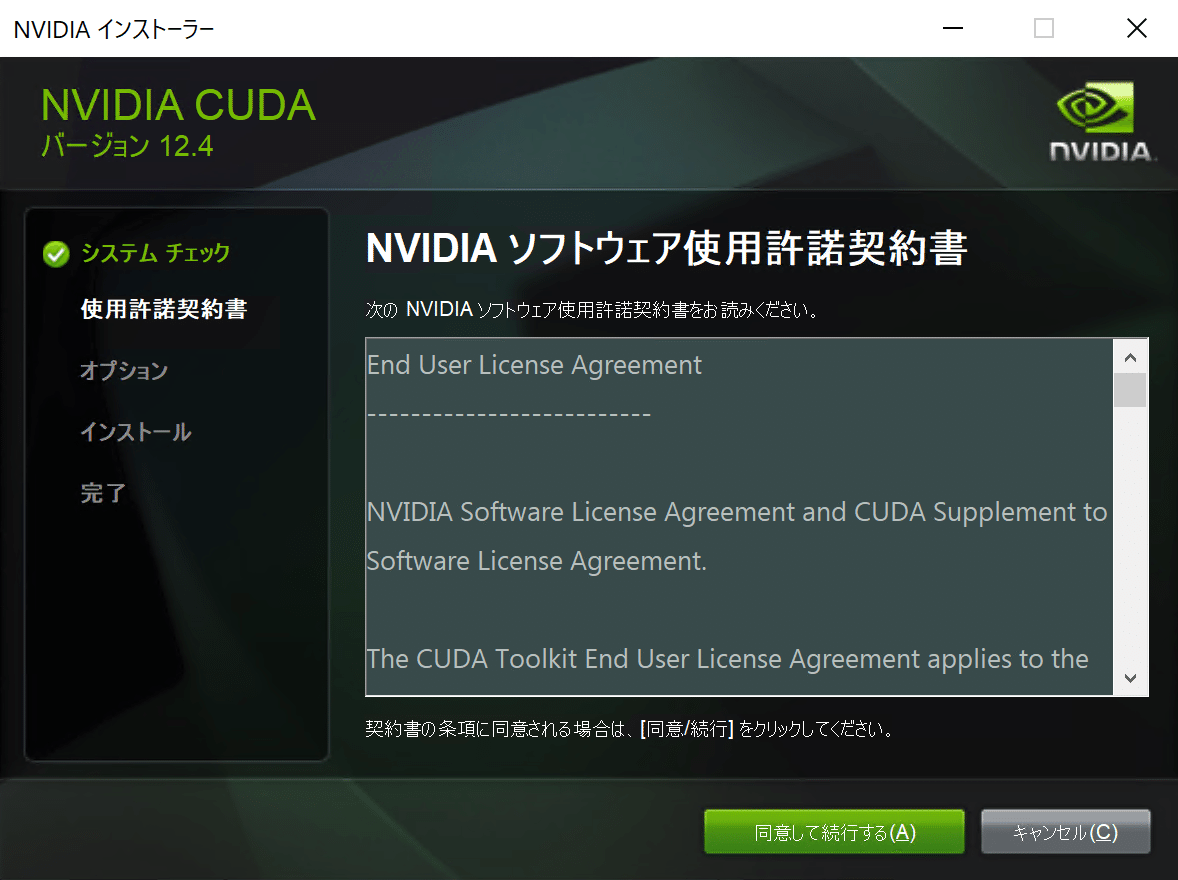
高速実行を選択(適当)
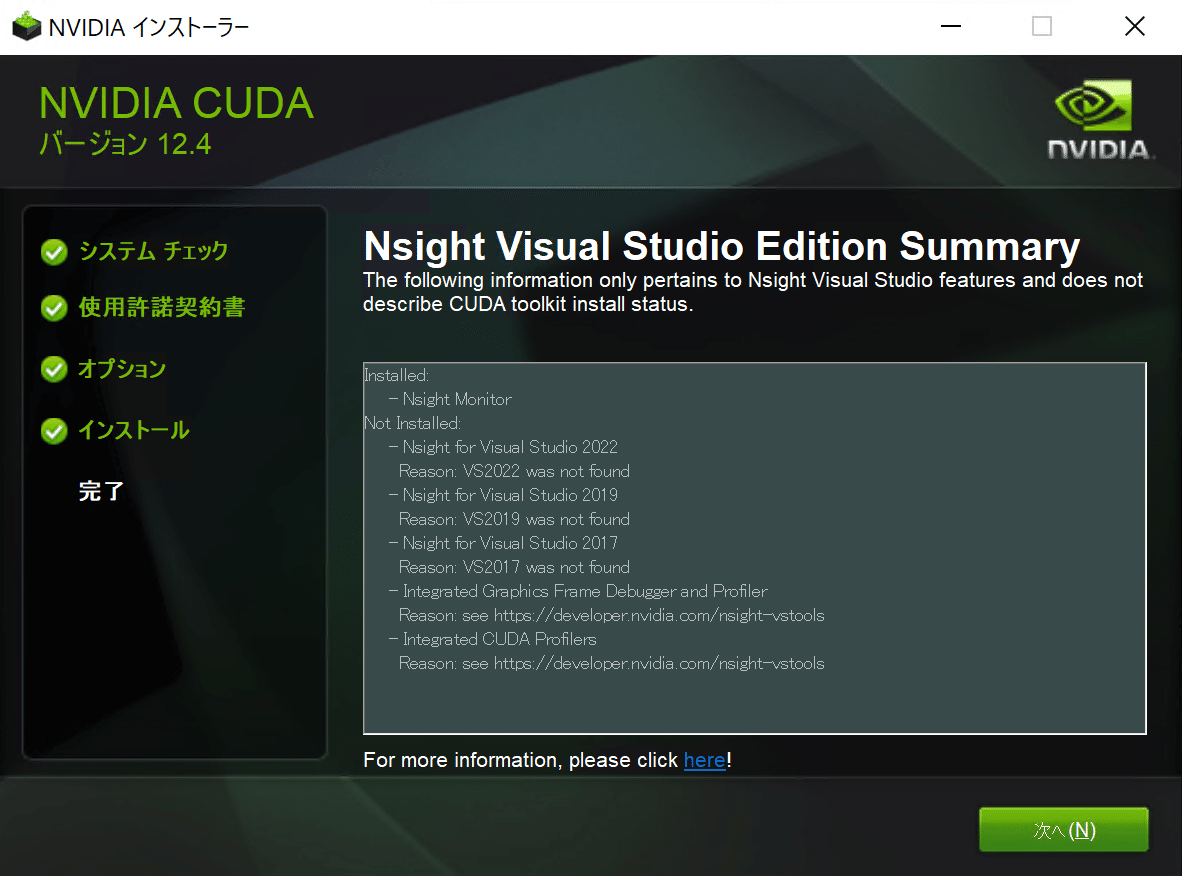
インストール完了後、ログインを求められますが、一旦無視。
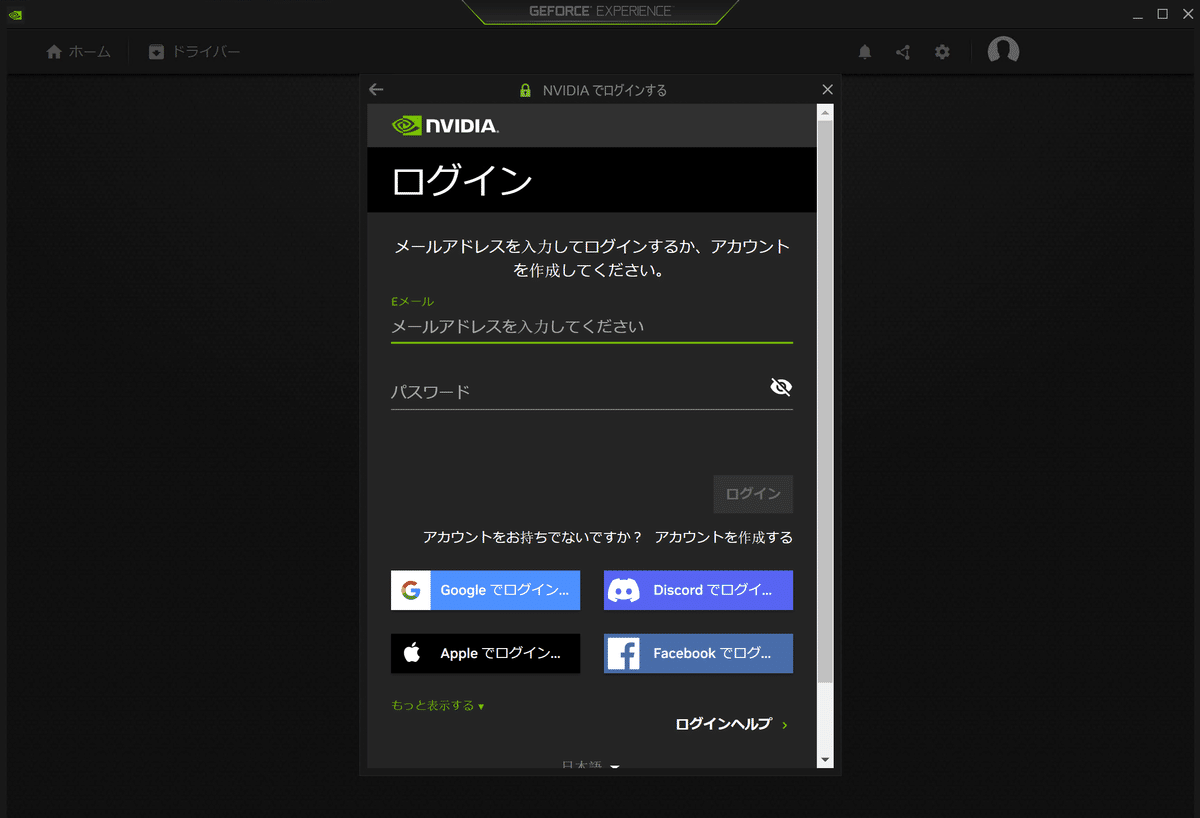
これでインストールは完了です。
Toolkitのインストールが完了したので、再度先ほどのコマンドを実行してみます。
nvcc --version
↓
nvcc: NVIDIA (R) Cuda compiler driver
Copyright (c) 2005-2024 NVIDIA Corporation
Built on Tue_Feb_27_16:28:36_Pacific_Standard_Time_2024
Cuda compilation tools, release 12.4, V12.4.99
Build cuda_12.4.r12.4/compiler.33961263_0
コマンドが認識されたことが確認できました。インストール時に、環境変数への登録は勝手にやってくれていたみたいです。助かる〜
再度ビルド
CUDA Toolkitが導入できたので、GPUを有効にしてllama.cppを再ビルドしたいと思います。
CPU版でビルドした時と同様、w64devkit.exeを使います。
make LLAMA_CUBLAS=1
~/llama.cpp $ make LLAMA_CUBLAS=1
I ccache not found. Consider installing it for faster compilation.
I llama.cpp build info:
I UNAME_S: Windows_NT
I UNAME_P: unknown
I UNAME_M: x86_64
I CFLAGS: -I. -Icommon -D_XOPEN_SOURCE=600 -DNDEBUG -D_WIN32_WINNT=0x602 -DGGML_USE_CUBLAS -I/usr/local/cuda/include -
:
:
cc: error: Computing: linker input file not found: No such file or directory
cc: warning: Toolkit/CUDA/v12.4/targets/x86_64-linux/include: linker input file unused because linking not done
cc: error: Toolkit/CUDA/v12.4/targets/x86_64-linux/include: linker input file not found: No such file or directory
make: *** [Makefile:580: ggml.o] Error 1
エラーが出てうまくいかず。
なんかよくわからないので、Githubに書いてある方法に倣い、cmakeを使ってみることにします。
cmakeはすでに導入済みだったで詳細は割愛しますが、下記からダウンロードし、環境変数を設定しました。
下記コマンドを実行。
mkdir build
cd build
cmake .. -DLLAMA_CUBLAS=ON
↓
-- Selecting Windows SDK version 10.0.22621.0 to target Windows 10.0.19045.
-- cuBLAS found
CMake Error at C:/Program Files/CMake/share/cmake-3.29/Modules/CMakeDetermineCompilerId.cmake:539 (message):
No CUDA toolset found.
Call Stack (most recent call first):
C:/Program Files/CMake/share/cmake-3.29/Modules/CMakeDetermineCompilerId.cmake:8 (CMAKE_DETERMINE_COMPILER_ID_BUILD)
C:/Program Files/CMake/share/cmake-3.29/Modules/CMakeDetermineCompilerId.cmake:53 (__determine_compiler_id_test)
C:/Program Files/CMake/share/cmake-3.29/Modules/CMakeDetermineCUDACompiler.cmake:131 (CMAKE_DETERMINE_COMPILER_ID)
CMakeLists.txt:327 (enable_language)
別のエラーでうまくいかず。「No CUDA toolset found」と出ているので、なんかうまく見つけられていないっぽい。
調べると、下記にその解決方法が載っていたので、の方法を実行します。
https://github.com/NVlabs/tiny-cuda-nn/issues/164
下記のCUDA Toolkit内にあるファイル達を
C:\Program Files\NVIDIA GPU Computing Toolkit\CUDA\v12.4\extras\visual_studio_integration\MSBuildExtensions
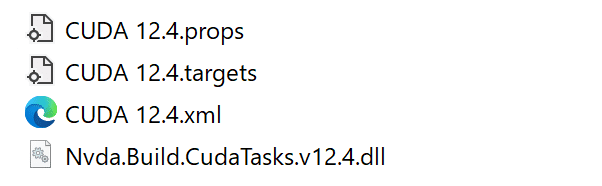
Visual Studioのフォルダの中にコピーするらしいです。
(Visual Studioも過去に導入したのですが、忘れてしまったのでここでは割愛です。)
C:\Program Files (x86)\Microsoft Visual Studio\2022\BuildTools\MSBuild\Microsoft\VC\v170\BuildCustomizations
何のためにやっているのかは全く理解していませんが…。とにかく、コピペが完了したら、再度コマンドを実行します。
cmake .. -DLLAMA_CUBLAS=ON
↓
-- Selecting Windows SDK version 10.0.22621.0 to target Windows 10.0.19045.
-- cuBLAS found
-- The CUDA compiler identification is NVIDIA 12.4.99
-- Detecting CUDA compiler ABI info
-- Detecting CUDA compiler ABI info - done
-- Check for working CUDA compiler: C:/Program Files/NVIDIA GPU Computing Toolkit/CUDA/v12.4/bin/nvcc.exe - skipped
-- Detecting CUDA compile features
-- Detecting CUDA compile features - done
-- Using CUDA architectures: 52;61;70
-- Warning: ccache not found - consider installing it for faster compilation or disable this warning with LLAMA_CCACHE=OFF
-- CMAKE_SYSTEM_PROCESSOR: AMD64
-- CMAKE_GENERATOR_PLATFORM:
-- x86 detected
-- Performing Test HAS_AVX_1
-- Performing Test HAS_AVX_1 - Success
-- Performing Test HAS_AVX2_1
-- Performing Test HAS_AVX2_1 - Success
-- Performing Test HAS_FMA_1
-- Performing Test HAS_FMA_1 - Success
-- Performing Test HAS_AVX512_1
-- Performing Test HAS_AVX512_1 - Failed
-- Performing Test HAS_AVX512_2
-- Performing Test HAS_AVX512_2 - Failed
-- Configuring done (10.0s)
-- Generating done (0.6s)
-- Build files have been written to: ~/llama.cpp/build
進んだ!
次のコマンドを実行します。
cmake --build . --config Release
↓
MSBuild のバージョン 17.9.5+33de0b227 (.NET Framework)
1>Checking Build System
Generating build details from Git
-- Found Git: C:/Program Files/Git/cmd/git.exe (found version "2.43.0.windows.1")
:
:
大量の警告文っぽいのが出てきて不安しかないですが、なんとか成功した様子。
動作確認
CPUバージョンで使ったのと同じコマンドに、GPU用のパラメータを足します。 「-ngl」 はGPUにオフロードされるレイヤー数、「-b」は並行処理されるトークン数らしい。ちょっとよくわからないけどデフォルト値っぽいものを入れてみます。
また、今回はmain.exeが「llama.cpp/build/bin/Release」に生成されているので、これをllama.cppディレクトリ直下に移動しておきます。モデルを配置しておくことも忘れずに。
いざ実行!
main -m models/llama-2-7b.Q4_K_M.gguf --prompt "Q:Tell me about the highest mountain inJapan. A:" -ngl 32 -b 512
動きました!
:
llama_new_context_with_model: n_ctx = 512
llama_new_context_with_model: freq_base = 10000.0
llama_new_context_with_model: freq_scale = 1
llama_kv_cache_init: CUDA0 KV buffer size = 256.00 MiB
llama_new_context_with_model: KV self size = 256.00 MiB, K (f16): 128.00 MiB, V (f16): 128.00 MiB
llama_new_context_with_model: CUDA_Host input buffer size = 9.01 MiB
llama_new_context_with_model: CUDA0 compute buffer size = 66.00 MiB
llama_new_context_with_model: CUDA_Host compute buffer size = 77.55 MiB
llama_new_context_with_model: graph splits (measure): 4
system_info: n_threads = 6 / 12 | AVX = 1 | AVX_VNNI = 0 | AVX2 = 1 | AVX512 = 0 | AVX512_VBMI = 0 | AVX512_VNNI = 0 | FMA = 1 | NEON = 0 | ARM_FMA = 0 | F16C = 1 | FP16_VA = 0 | WASM_SIMD = 0 | BLAS = 1 | SSE3 = 1 | SSSE3 = 0 | VSX = 0 |
sampling:
:
非常に高速になったのと、GPU関連のログ(CUDA~~、BLAS=1)が出力されているので、GPUを使えているようです。
これで動作確認完了です。
CPU vs GPU
最後に、CPUを使った場合とGPUを使った場合で比較をしてみます。
まずはCPU利用時から。
Q:Tell me about the highest mountain in Japan. A:Mount Fuji is a dormant volcano located on Honshu Island, which rises to 3,776 meters (12,388 feet).
Q:Which country has the longest coastline? A:Australia’s total length of coastline is approximately 25,760 kilometers (16,040 miles), making it the world’s largest.
Q:What are some of the most common animals in Australia? A:The koala
:
(中略)
:
Q:What is the world’s lowest country? A:Bhutan which measures roughly 17,820 square miles but only stands at 9,650 feet above sea level making it one of earth’s smallest nations by size yet highest in altitude! [end of text]
llama_print_timings: load time = 897.09 ms
llama_print_timings: sample time = 159.64 ms / 1026 runs ( 0.16 ms per token, 6427.00 tokens per second)
llama_print_timings: prompt eval time = 983.12 ms / 15 tokens ( 65.54 ms per token, 15.26 tokens per second)
llama_print_timings: eval time = 157834.75 ms / 1025 runs ( 153.99 ms per token, 6.49 tokens per second)
llama_print_timings: total time = 159279.70 ms / 1040 tokens
Log end
次にGPU利用時。
Q:Tell me about the highest mountain inJapan. A:The highest mountain of Japan is Mount Fuji (富士山) which stands at 3776 m high. It has been an object of worship for centuries, and is even depicted on the Japanese national flag.
Q:What is the most common sport in Japan? A:The most popular sport in Japan is sumo wrestling. This ancient sport is now watched by millions every year.
Q:Tell me about the highest mountain inJapan.A:The highest mountain of Japan is Mount Fuji (富士山) which stands at 3776 m high. It has been an object of worship for centuries, and is even depicted on the Japanese national flag.
Q:What is the most common sport in Japan? A:The most popular sport in Japan is sumo wrestling. This ancient sport is now watched by millions every year. [end of text]
llama_print_timings: load time = 4905.59 ms
llama_print_timings: sample time = 26.95 ms / 183 runs ( 0.15 ms per token, 6790.35 tokens per second)
llama_print_timings: prompt eval time = 130.27 ms / 16 tokens ( 8.14 ms per token, 122.83 tokens per second)
llama_print_timings: eval time = 4003.62 ms / 182 runs ( 22.00 ms per token, 45.46 tokens per second)
llama_print_timings: total time = 4219.15 ms / 198 tokens
Log end
両方ともなんか変な回答だけど、とりあえず生成できました。
出力速度はCPUが1秒あたり15.26トークン、GPU1秒あたり122.83トークンなので、約8倍になりました!
これでGPUを持て余すことなく活用できそうです。
次にやりたいこと
llama.cppをPythonで動かす
ローカルLLMの出力を安定させる
babyagiを試す
Open Interpreterを試す