成人2型糖尿病: SGLT2i治療開始は治療前HbA1c値と関連なく、修正MACE・HHFのリスク減少するも、少ないながら性器感染症とDKAのリスクが増加、ただAKIのリスク減少 (vs DPP4i)
市販後の大規模な心血管アウトカム無作為化試験(CVOT)において、ナトリウム・グルコース共輸送体2阻害薬(SGLT2i)は、T2Dおよび心血管または腎臓の疾患が確立した患者において心腎保護効果を実証。。これらの効果は、臨床現場で見られるような幅広い心血管リスクを有する患者集団を対象としたリアルワールド・エビデンス試験で再現。高血糖の程度が異なる患者がSGLT2iの使用により同様に利益を得られるかどうかはまだ明らかではなかった。T2D患者は血糖コントロールにかかわらずSGLT2iの使用が有益であり、DPP-4iと比較しても期待通りの副作用プロファイルで、HbA1c値が高い患者における副作用リスクの追加もない。
Comparing Effectiveness and Safety of SGLT2 Inhibitors vs DPP-4 Inhibitors in Patients With Type 2 Diabetes and Varying Baseline HbA1c Levels
Elvira D’Andrea, et al.
JAMA Intern Med. Published online February 6, 2023. doi:10.1001/jamainternmed.2022.6664
https://jamanetwork.com/journals/jamainternalmedicine/fullarticle/2800884
SGLT2iの副事象をいかにコントロールするか。副事象も治療前のHbA1値と関連してないことを考えるとベネフィットにくらべ少ないながら以下にこれらの副事象対応するかが臨床の課題となるのだろう
性器感染症:IR/1000 PY 1847 vs 780 (SGLT2i vs DPP-4i)
ケトアシドーシス: IR/1000 PY 66 vs 36 (SGLT2i vs DPP-4i)
キーポイント
【疑問点】 2型糖尿病患者全体およびベースラインのヘモグロビンA1c(HbA1c)値が異なる場合、ナトリウム・グルコース共輸送体2阻害薬(SGLT2i)の有効性および安全性はジペプチジルペプチダーゼ4阻害薬(DPP-4i)のそれと異なるか。
【結果】propensity-scored match化された成人2型糖尿病患者87 274例を含むこの大規模な新規使用者の有効性と安全性の比較研究において、SGLT2i治療開始者はDPP-4i治療開始者と比較して、ベースラインのHbA1c値にかかわらず、主要心血管イベント、心不全、急性腎障害のリスクが低下し、性器感染症、糖尿病性ケトアシドーシスのリスクが増加した。
【意義】 HbA1c値が高い状態でのSGLT2iの使用は過剰なリスクを引き起こすという懸念があるものの、本試験の結果から、T2D患者は血糖コントロールにかかわらずSGLT2iの使用が有益であり、DPP-4iと比較しても期待通りの副作用プロファイルで、HbA1c値が高い患者における副作用リスクの追加もないことが示唆された。
Translated with DeepL
【意義】 Sodium-glucose cotransporter 2 inhibitor(SGLT2i)療法は,心血管に有益であり,有害事象は少ない。しかし,ベースラインのヘモグロビンA1c(HbA1c)値の違いにより,比較有効性と安全性プロファイルが異なるかどうかは不明である。
【目的】 成人2型糖尿病(T2D)患者において、SGLT2iとジペプチジルペプチダーゼ4阻害薬(DPP-4i)治療の心血管効果および安全性を、(1)全体および(2)ベースラインのHbA1c値の違いにより比較すること。
【デザイン、設定、被験者】 SGLT2iまたはDPP-4iによる治療を開始し、ベースラインでT2D診断が記録され、治療開始前3ヶ月以内に少なくとも1回のHbA1c検査結果が記録されている、商業保険の成人144 614人を対象に新規ユーザーの有効性および安全性に関する比較研究試験を実施した。
【介入】 SGLT2iまたはDPP-4iによる治療の開始が介入とされた。
【主要評価項目】 主要評価項目は、心筋梗塞、脳卒中、全死亡(修正主要有害心血管イベント[MACE])および心不全による入院(HHF)の複合であった。安全性のアウトカムは、低ボレミア、骨折、転倒、生殖器感染症、糖尿病性ケトアシドーシス(DKA)、急性腎障害(AKI)、下肢切断とした。1000人年当たりの発生率(IR),ハザード比(HR),発生率差(RD)とその95%CIを128の共変量で制御して推定した。
【結果】 SGLT2i(n=60523)またはDPP-4i(n=84091)による治療を開始したT2Dの成人適格者144 614人(平均[SD]年齢、62[12.4]歳、54%男性参加)を特定した。HbA1cベースライン値は、44099が7.5%未満、52 986が7.5%から9%の間、47 529が9%を超えていた。全体として、87人の適格な患者が1:1のpropensityスコアでマッチされた:HbA1cが7.5%未満の24 052人、HbA1cが7.5%から9%の32 290人、HbA1cが9%を超える30 932人(総ヘモグロビンの割合に変換するには、0.01倍してください)。
開始薬剤SGLT2i vs DPP-4iは、平均フォローアップ期間8ヶ月において、修正MACEリスク低下 (IR per 1000 person-years 17.13 vs 20.18, respectively; HR, 0.85; 95% CI, 0.75-0.95; RD, −3.02; 95% CI, −5.23 to –0.80) と関連、同様、HHF (IR per 1000 person-years 3.68 vs 8.08, respectively; HR, 0.46; 95% CI, 0.35 to 0.57; RD −4.37; 95% CI, −5.62 to −3.12) とも関連し、HbA1c値間の治療効果heterogeneityは認めなかった。
SGLT2iによる治療では、DPP-4iと比較して、性器感染症およびDKAのリスクが増加し、AKIリスクは減少した。HbA1c値によって結果は一貫していたが、HbA1c値7.5%~9%ではSGLT2iによる性器感染症のリスクがより顕著であった(1000人年あたりのIR 68.5 vs 22.8; HR, 3.10; 95% CI, 2.68-3.58; RD, 46.22; 95% CI, 40.54-51.90 )。
【結論と関連性】 この成人T2D患者における有効性と安全性の比較研究において、SGLT2i治療開始者はDPP-4iの治療開始者と比べベースラインのHbA1cにかかわらず、修正MACEとHHFのリスクが減少し、性器感染症とDKAのリスクが増加し、AKIのリスクが減少していた。
Translated with DeepL
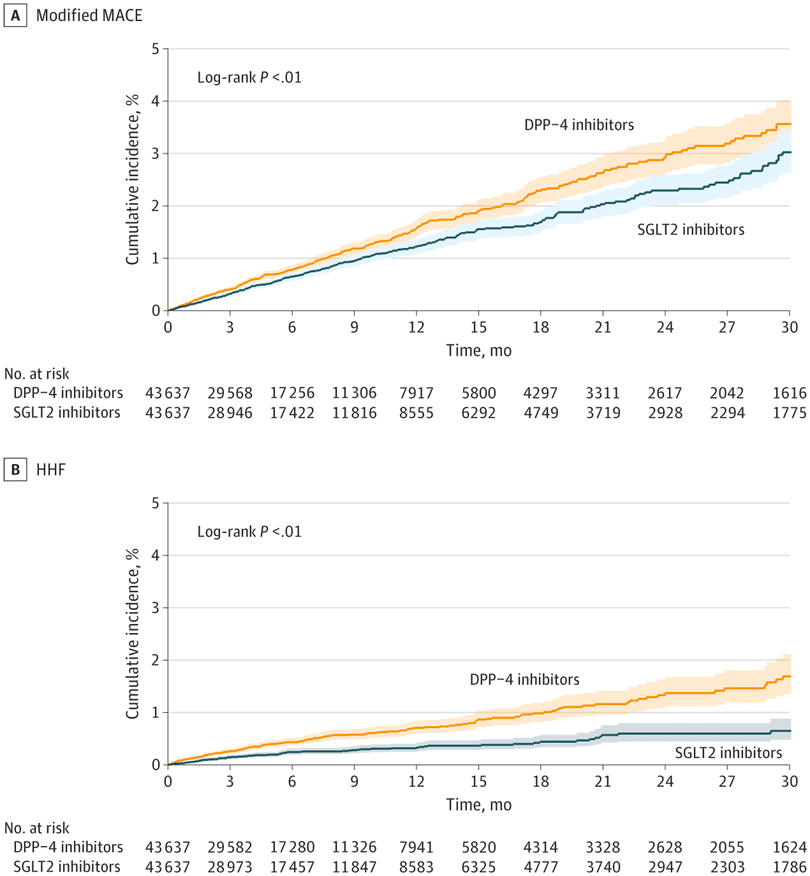
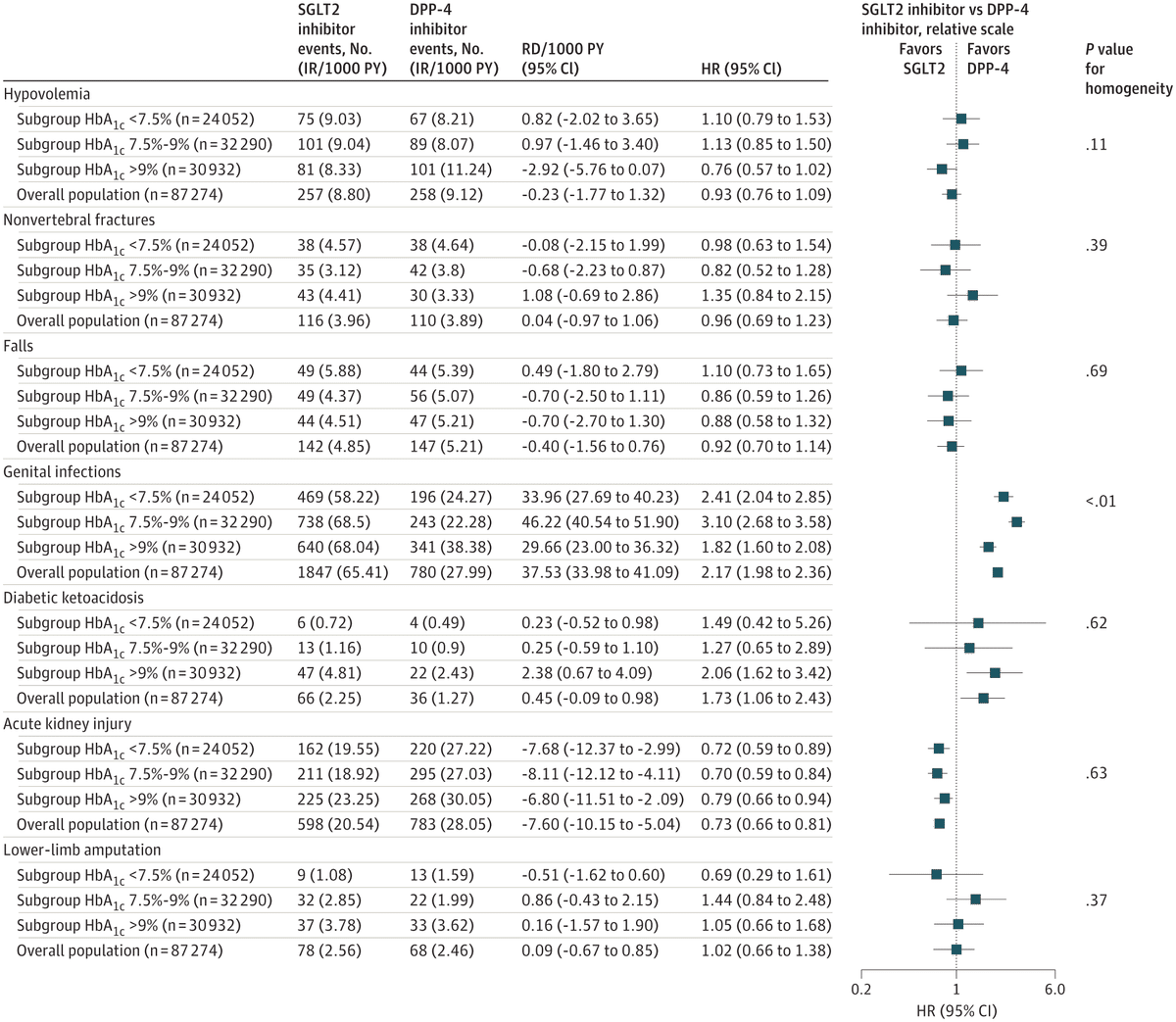
上の論文とは全く関係ないが・・・
Propensity matchingについてのpythonでの使い方教えて下さい
ChatGPT:
Here's an example of how you could use propensity matching in Python:
import pandas as pd
from sklearn.neighbors import NearestNeighbors
# Load the data into a pandas DataFrame
df = pd.read_csv("data.csv")
# Estimate the propensity score using logistic regression
from sklearn.linear_model import LogisticRegression
X = df.drop("outcome", axis=1)
y = df["outcome"]
propensity_model = LogisticRegression().fit(X, y)
propensity_scores = propensity_model.predict_proba(X)[:, 1]
# Find nearest neighbors with matching propensity scores
neigh = NearestNeighbors(n_neighbors=1)
neigh.fit(propensity_scores.reshape(-1, 1))
control_indices = neigh.kneighbors(propensity_scores.reshape(-1, 1), return_distance=False)
# Use the nearest neighbors to create the matched samples
control_df = df.iloc[control_indices.flatten()].drop("outcome", axis=1)
treated_df = df[df["outcome"] == 1].drop("outcome", axis=1)
# Compare the outcomes between the treated and control groups
# (you could use any statistical test or visualization here)
In this example, we first fit a logistic regression model to predict the outcome based on the other variables in the data (X). We then use the model to estimate the propensity score for each individual in the data, which represents their probability of being treated (propensity_scores).
Next, we use the NearestNeighbors class from scikit-learn to find the nearest neighbor for each individual with the same propensity score. We then create two separate DataFrames, one for the treated group (treated_df) and one for the control group (control_df). Finally, we can perform statistical comparisons or visualizations to compare the outcomes between the two groups.
Note that this is just one example of how you could use propensity matching in Python, and there are many other ways to implement it, including using other algorithms or packages.
Regenerate response