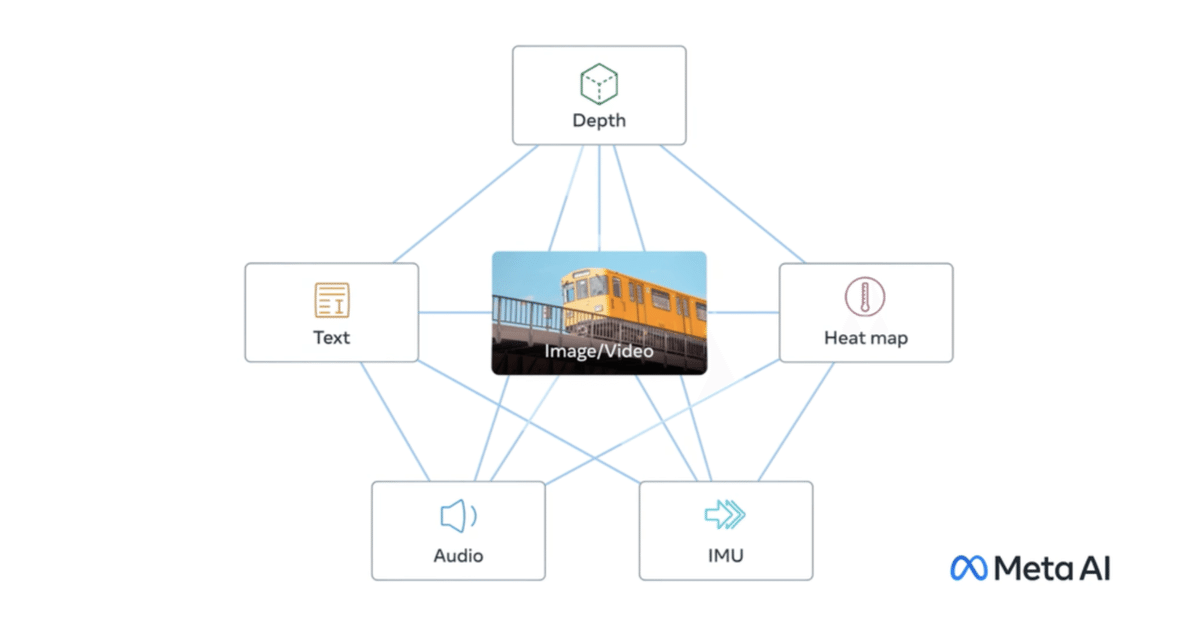
Google Colab で ImageBind を試す
「Google Colab」で「ImageBind」を試したので、まとめました。
1. ImageBind
「ImageBind」は、「画像」「テキスト」「音声」「深度(3D)」「熱」「IMU(慣性測定ユニット)」といった6つの異なるモダリティにまたがる共同埋め込みを学習します。これにより、クロスモーダル検索、演算によるモダリティの合成、クロスモーダル検出や生成など、すぐに使える新しい創発的アプリケーションを実現します。
2. Colabでの実行
Colabでの実行手順は、次のとおりです。
(1) メニュー「編集→ノートブックの設定」で、「ハードウェアアクセラレータ」で「GPU」を選択。
(2) パッケージのインストール。
# パッケージのインストール
!git clone https://github.com/facebookresearch/ImageBind
%cd ImageBind
!pip install -r requirements.txt
(3) デバイスの準備。
GPUとCPUをどちらを使うかの情報です。
import torch
# デバイスの準備
device = "cuda:0" if torch.cuda.is_available() else "cpu"
(4) モダリティ (テキスト・画像・音声) の準備。
import data
from models.imagebind_model import ModalityType
# モダリティ (テキスト・画像・音声) の準備
text_list=["A dog.", "A car", "A bird"]
image_paths=[".assets/dog_image.jpg", ".assets/car_image.jpg", ".assets/bird_image.jpg"]
audio_paths=[".assets/dog_audio.wav", ".assets/car_audio.wav", ".assets/bird_audio.wav"]
inputs = {
ModalityType.TEXT: data.load_and_transform_text(text_list, device),
ModalityType.VISION: data.load_and_transform_vision_data(image_paths, device),
ModalityType.AUDIO: data.load_and_transform_audio_data(audio_paths, device),
}
以下のメソッドでデータを読み込みます。
・data.load_and_transform_text()
・data.load_and_transform_vision_data()
・data.load_and_transform_audio_data()
画像と音声は、.assetsフォルダにあります。
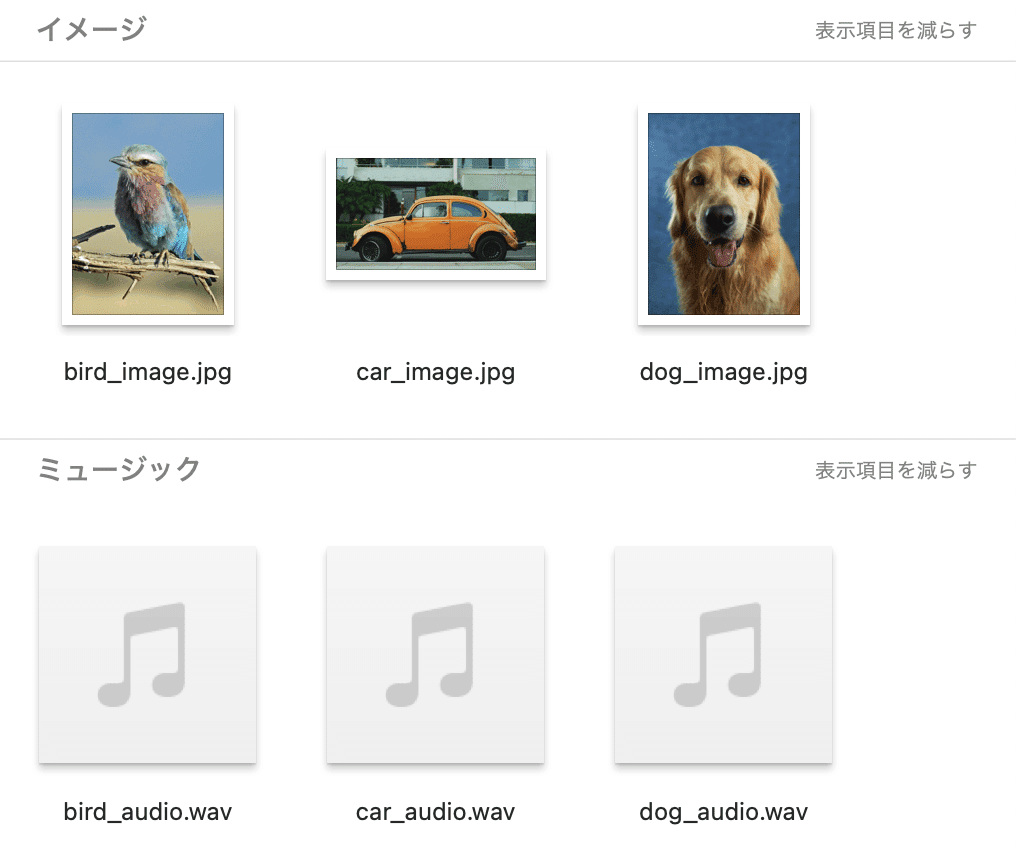
(5) モデルの準備。
from models import imagebind_model
# モデルの準備
model = imagebind_model.imagebind_huge(pretrained=True)
model.eval()
model.to(device)
以下のメソッドでモデルを読み込みます。
・imagebind_model.imagebind_huge()
(6) 推論の実行。
# 推論の実行
with torch.no_grad():
embeddings = model(inputs)
# 結果の確認
print(
"Vision x Text: ",
torch.softmax(embeddings[ModalityType.VISION] @ embeddings[ModalityType.TEXT].T, dim=-1),
)
print(
"Audio x Text: ",
torch.softmax(embeddings[ModalityType.AUDIO] @ embeddings[ModalityType.TEXT].T, dim=-1),
)
print(
"Vision x Audio: ",
torch.softmax(embeddings[ModalityType.VISION] @ embeddings[ModalityType.AUDIO].T, dim=-1),
)
Vision x Text: tensor([
[9.9684e-01, 3.1310e-03, 2.5929e-05],
[5.4495e-05, 9.9993e-01, 2.0353e-05],
[4.4847e-05, 1.3246e-02, 9.8671e-01]], device='cuda:0')
Audio x Text: tensor([
[1., 0., 0.],
[0., 1., 0.],
[0., 0., 1.]], device='cuda:0')
Vision x Audio: tensor([
[0.8064, 0.1051, 0.0885],
[0.1284, 0.7205, 0.1511],
[0.0016, 0.0022, 0.9962]], device='cuda:0')
「画像 x テキスト」「音声 x テキスト」「画像 x 音声」のモダリティの距離が出力されます。1に近いほど類似してることを示します。「dog, car, bird」同士を比較してるため、対角線上に1に近い値が並んでいます。
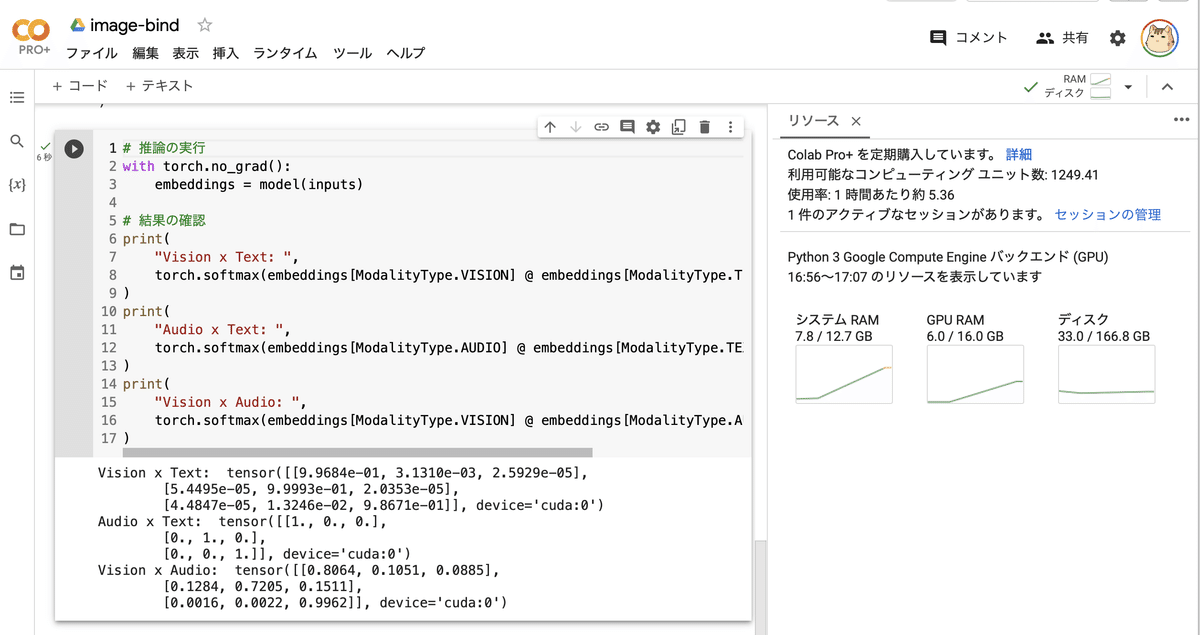