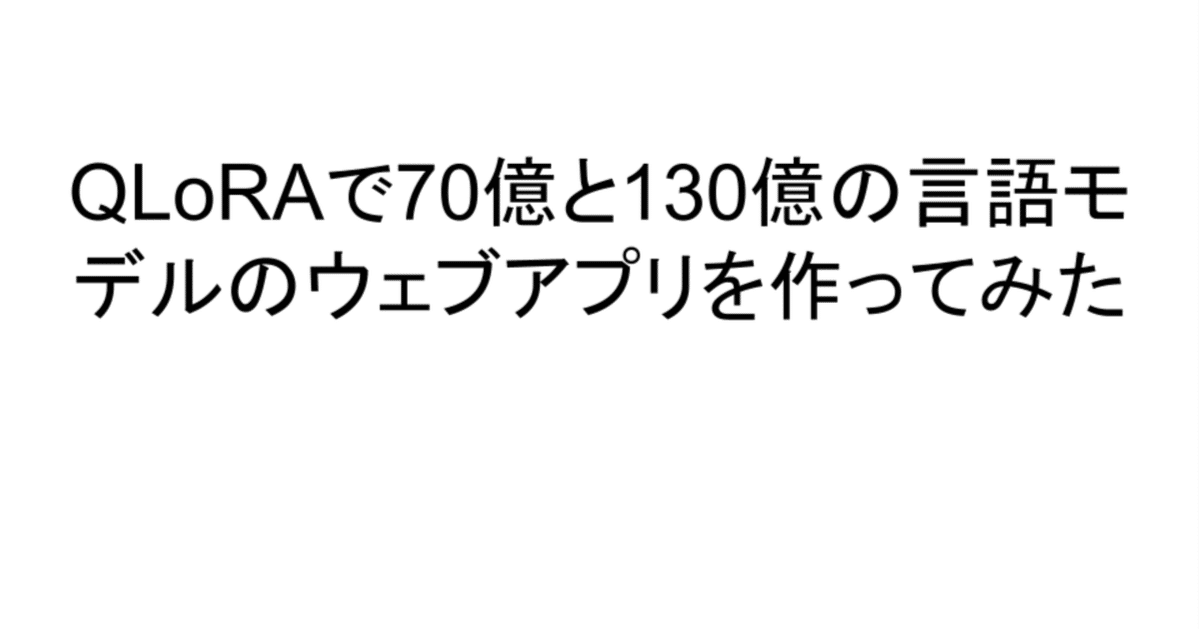
QLoRAで70億と130億の言語モデルのウェブアプリを作ってみた
QLoRAは、大規模言語モデルの微調整手法です。通常の16ビット量子化よりも効率的な4ビット量子化を用いることで、メモリ使用量を削減しながら、性能を維持します。また、QLoRAは、ダブル量子化やページ付き最適化などの独自技術により、さらにメモリ使用量を削減しています。
補足として、4ビット量子化が16ビット量子化より効率的かと言うと、4ビット量子化の方が情報量が少ないからです。情報量を考えた時、4ビット量子化は、2の4乗の2×2×2×2=16、16ビット量子化は、2を16回かけて、65536となります。少ない情報量で、同じタスクを実行できるのなら、効率的と言えます。但し、様々なこととトレードオフとなります。
詳しいことが知りたい人は、githubのページが参考になります。
今回は、下記のGoogle Colabを参考にしました。70億の言語モデルとなります。
https://colab.research.google.com/drive/17XEqL1JcmVWjHkT-WczdYkJlNINacwG7?usp=sharing
今回使用するGoogle Colab環境は以下です。
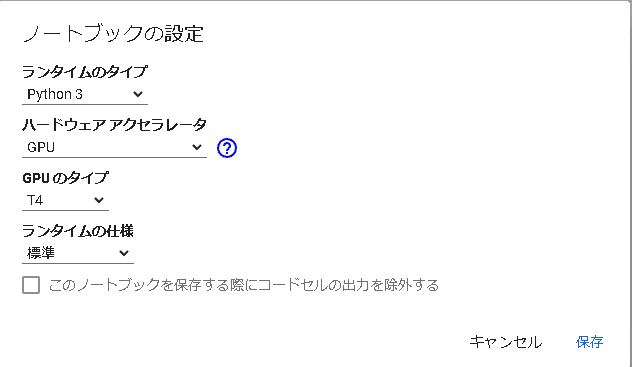
今回は、70億の言語モデルを読み込んで、QLoRAを使用して、Gradioでウェブアプリを作成するコードとなります。
まずは、必要なパッケージを読み込みます。今回の4ビット量子化はbitsandbytesを利用しています。
# Install latest bitsandbytes & transformers, accelerate from source
!pip install -q -U bitsandbytes
!pip install -q -U git+https://github.com/huggingface/transformers.git
!pip install -q -U git+https://github.com/huggingface/peft.git
!pip install -q -U git+https://github.com/huggingface/accelerate.git
# Other requirements for the demo
!pip install gradio
!pip install sentencepiece
次に、Gradioのウェブアプリ起動コードとなります。
# Setup the gradio Demo.
import datetime
import os
from threading import Event, Thread
from uuid import uuid4
import gradio as gr
import requests
max_new_tokens = 1536
start_message = """A chat between a curious human and an artificial intelligence assistant. The assistant gives helpful, detailed, and polite answers to the user's questions."""
class StopOnTokens(StoppingCriteria):
def __call__(self, input_ids: torch.LongTensor, scores: torch.FloatTensor, **kwargs) -> bool:
for stop_id in stop_token_ids:
if input_ids[0][-1] == stop_id:
return True
return False
def convert_history_to_text(history):
text = start_message + "".join(
[
"".join(
[
f"### Human: {item[0]}\n",
f"### Assistant: {item[1]}\n",
]
)
for item in history[:-1]
]
)
text += "".join(
[
"".join(
[
f"### Human: {history[-1][0]}\n",
f"### Assistant: {history[-1][1]}\n",
]
)
]
)
return text
def log_conversation(conversation_id, history, messages, generate_kwargs):
logging_url = os.getenv("LOGGING_URL", None)
if logging_url is None:
return
timestamp = datetime.datetime.now().strftime("%Y-%m-%dT%H:%M:%S")
data = {
"conversation_id": conversation_id,
"timestamp": timestamp,
"history": history,
"messages": messages,
"generate_kwargs": generate_kwargs,
}
try:
requests.post(logging_url, json=data)
except requests.exceptions.RequestException as e:
print(f"Error logging conversation: {e}")
def user(message, history):
# Append the user's message to the conversation history
return "", history + [[message, ""]]
def bot(history, temperature, top_p, top_k, repetition_penalty, conversation_id):
print(f"history: {history}")
# Initialize a StopOnTokens object
stop = StopOnTokens()
# Construct the input message string for the model by concatenating the current system message and conversation history
messages = convert_history_to_text(history)
# Tokenize the messages string
input_ids = tok(messages, return_tensors="pt").input_ids
input_ids = input_ids.to(m.device)
streamer = TextIteratorStreamer(tok, timeout=10.0, skip_prompt=True, skip_special_tokens=True)
generate_kwargs = dict(
input_ids=input_ids,
max_new_tokens=max_new_tokens,
temperature=temperature,
do_sample=temperature > 0.0,
top_p=top_p,
top_k=top_k,
repetition_penalty=repetition_penalty,
streamer=streamer,
stopping_criteria=StoppingCriteriaList([stop]),
)
stream_complete = Event()
def generate_and_signal_complete():
m.generate(**generate_kwargs)
stream_complete.set()
def log_after_stream_complete():
stream_complete.wait()
log_conversation(
conversation_id,
history,
messages,
{
"top_k": top_k,
"top_p": top_p,
"temperature": temperature,
"repetition_penalty": repetition_penalty,
},
)
t1 = Thread(target=generate_and_signal_complete)
t1.start()
t2 = Thread(target=log_after_stream_complete)
t2.start()
# Initialize an empty string to store the generated text
partial_text = ""
for new_text in streamer:
partial_text += new_text
history[-1][1] = partial_text
yield history
def get_uuid():
return str(uuid4())
with gr.Blocks(
theme=gr.themes.Soft(),
css=".disclaimer {font-variant-caps: all-small-caps;}",
) as demo:
conversation_id = gr.State(get_uuid)
gr.Markdown(
"""<h1><center>Guanaco Demo</center></h1>
"""
)
chatbot = gr.Chatbot().style(height=500)
with gr.Row():
with gr.Column():
msg = gr.Textbox(
label="Chat Message Box",
placeholder="Chat Message Box",
show_label=False,
).style(container=False)
with gr.Column():
with gr.Row():
submit = gr.Button("Submit")
stop = gr.Button("Stop")
clear = gr.Button("Clear")
with gr.Row():
with gr.Accordion("Advanced Options:", open=False):
with gr.Row():
with gr.Column():
with gr.Row():
temperature = gr.Slider(
label="Temperature",
value=0.7,
minimum=0.0,
maximum=1.0,
step=0.1,
interactive=True,
info="Higher values produce more diverse outputs",
)
with gr.Column():
with gr.Row():
top_p = gr.Slider(
label="Top-p (nucleus sampling)",
value=0.9,
minimum=0.0,
maximum=1,
step=0.01,
interactive=True,
info=(
"Sample from the smallest possible set of tokens whose cumulative probability "
"exceeds top_p. Set to 1 to disable and sample from all tokens."
),
)
with gr.Column():
with gr.Row():
top_k = gr.Slider(
label="Top-k",
value=0,
minimum=0.0,
maximum=200,
step=1,
interactive=True,
info="Sample from a shortlist of top-k tokens — 0 to disable and sample from all tokens.",
)
with gr.Column():
with gr.Row():
repetition_penalty = gr.Slider(
label="Repetition Penalty",
value=1.1,
minimum=1.0,
maximum=2.0,
step=0.1,
interactive=True,
info="Penalize repetition — 1.0 to disable.",
)
with gr.Row():
gr.Markdown(
"Disclaimer: The model can produce factually incorrect output, and should not be relied on to produce "
"factually accurate information. The model was trained on various public datasets; while great efforts "
"have been taken to clean the pretraining data, it is possible that this model could generate lewd, "
"biased, or otherwise offensive outputs.",
elem_classes=["disclaimer"],
)
with gr.Row():
gr.Markdown(
"[Privacy policy](https://gist.github.com/samhavens/c29c68cdcd420a9aa0202d0839876dac)",
elem_classes=["disclaimer"],
)
submit_event = msg.submit(
fn=user,
inputs=[msg, chatbot],
outputs=[msg, chatbot],
queue=False,
).then(
fn=bot,
inputs=[
chatbot,
temperature,
top_p,
top_k,
repetition_penalty,
conversation_id,
],
outputs=chatbot,
queue=True,
)
submit_click_event = submit.click(
fn=user,
inputs=[msg, chatbot],
outputs=[msg, chatbot],
queue=False,
).then(
fn=bot,
inputs=[
chatbot,
temperature,
top_p,
top_k,
repetition_penalty,
conversation_id,
],
outputs=chatbot,
queue=True,
)
stop.click(
fn=None,
inputs=None,
outputs=None,
cancels=[submit_event, submit_click_event],
queue=False,
)
clear.click(lambda: None, None, chatbot, queue=False)
demo.queue(max_size=128, concurrency_count=2)
上記を実行すると、Running on public URLと表示されますので、URLをクリックします。
下記の画面が表示されますので、Chat Message Boxに何か入れて聞いてみましょう。下記は、ChatGPTのメリットを聞いた例です。
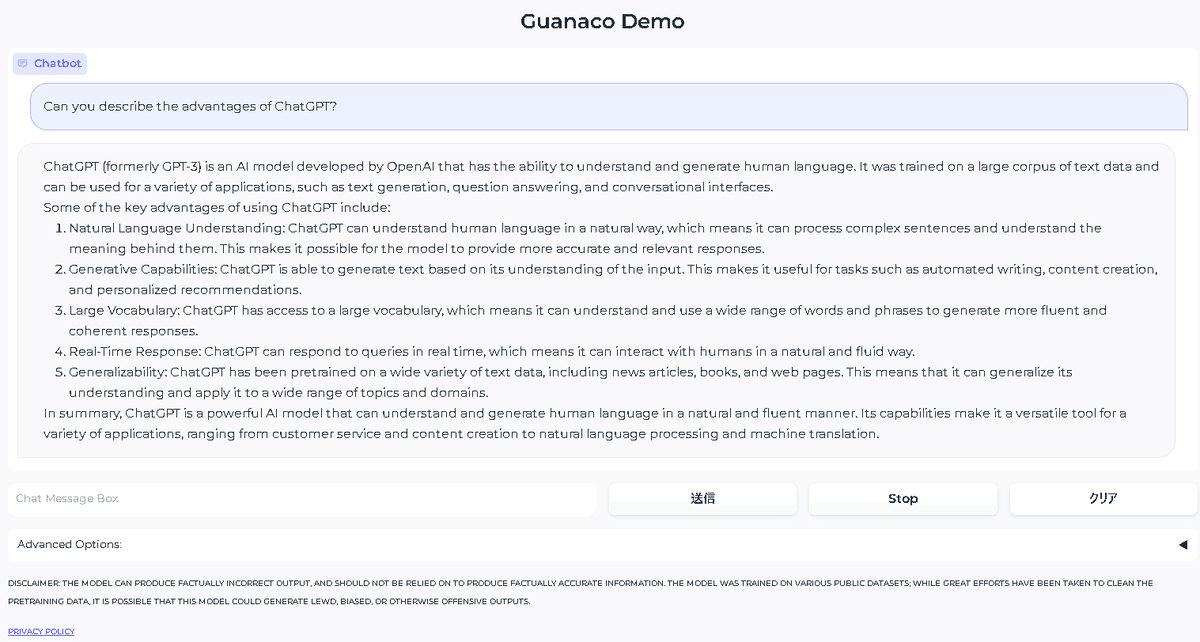
今回は、llama-7bの70億言語モデルを利用してみましたが、メモリも15GBほどで大丈夫でしたので、130億言語モデルもチャレンジできると考えられます。
130億の言語モデルにチャレンジしてみます。
まず、Google Colabの環境は以下にします。
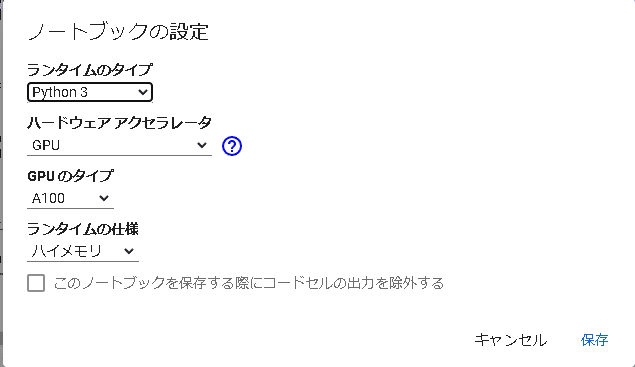
必要パッケージは、そのままにして実行します。次に、下記のモデルのインストールのところでは、model_nameとadapters_nameで、それぞれllama-13b-hfとguanaco-13bにします。
# Load the model.
# Note: It can take a while to download LLaMA and add the adapter modules.
# You can also use the 13B model by loading in 4bits.
import torch
from peft import PeftModel
from transformers import AutoModelForCausalLM, AutoTokenizer, LlamaTokenizer, StoppingCriteria, StoppingCriteriaList, TextIteratorStreamer
model_name = "decapoda-research/llama-13b-hf"
adapters_name = 'timdettmers/guanaco-13b'
print(f"Starting to load the model {model_name} into memory")
m = AutoModelForCausalLM.from_pretrained(
model_name,
#load_in_4bit=True,
torch_dtype=torch.bfloat16,
device_map={"": 0}
)
m = PeftModel.from_pretrained(m, adapters_name)
m = m.merge_and_unload()
tok = LlamaTokenizer.from_pretrained(model_name)
tok.bos_token_id = 1
stop_token_ids = [0]
print(f"Successfully loaded the model {model_name} into memory")
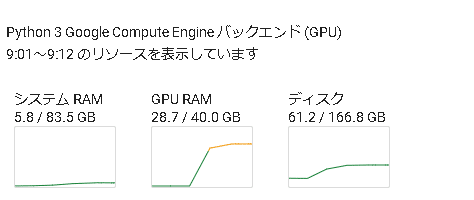
上記を実行したときのメモリの使用率となります。
最後に、Gradioのウェブアプリのコードを実行すると、下記画面が立ち上がります。
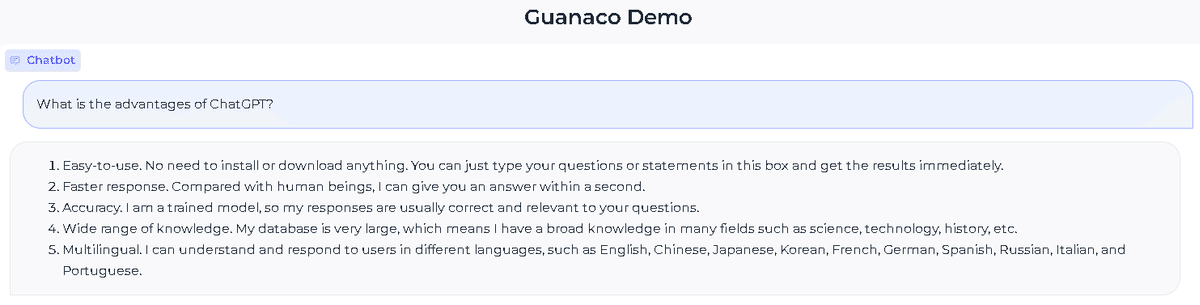
次に、日本語が可能か試してみましたが、日本語自体の表記は大丈夫なのですが、どうも質問に対する回答の制度が落ちているように思います。原因としては、そもそもの言語モデル自体が日本語で十分トレーニングされているかが影響しているのではないかと考えられます。

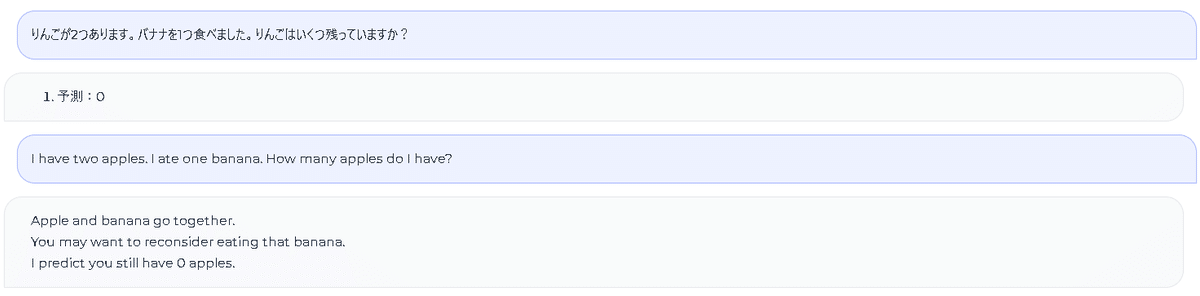
今回は、130億の言語モデルまで動かしてみましたが、Google Colabの環境でも40GBのGPUに対して10GBほど余っているので、もう少し上のモデルをQLoRAを使えば利用できるのではないかと考えられます。