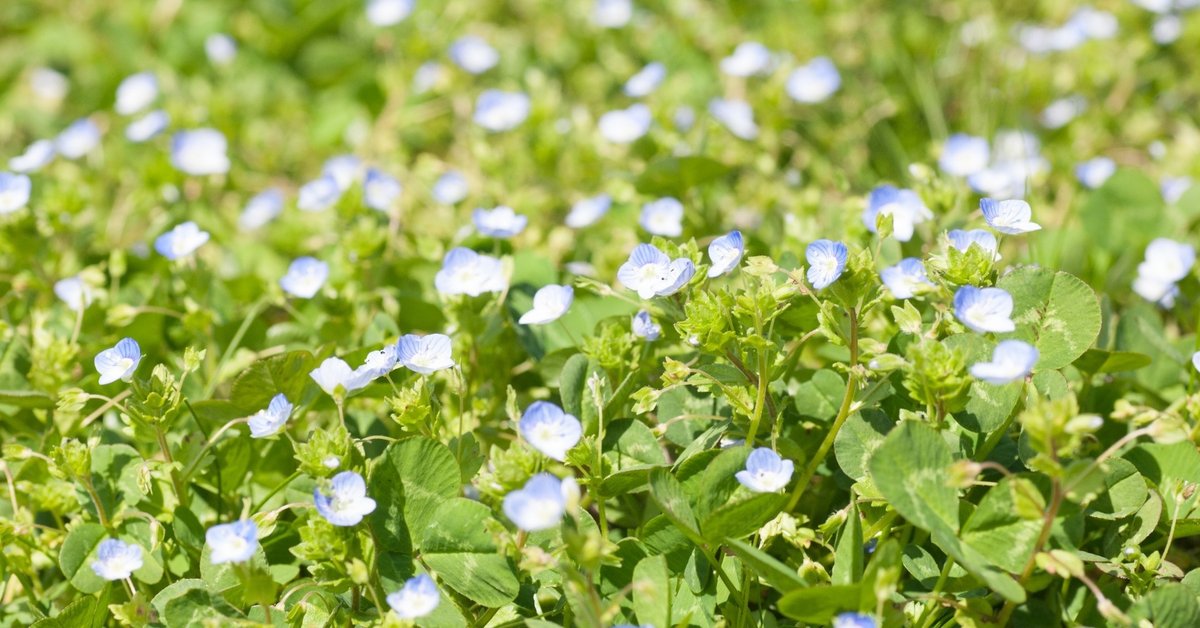
ML.netでModel Builderを使ってみる その5
前回の続きです。
ソースコードを見ながら、思ってしまいました。
ModelBuilder.csは全く使っていないのではと。
試しに全部コメントアウトして実行してみますが、やはり実行されていません。
ModelBuilder.csを削除しますが、もっと考えてコピーするべきだったかなと思っています。
余計なソースコードが消えたので、とても使いやすいソースコードになりました。
その上、もし画像判定の学習内容を変更したい場合、プログラムと同一のフォルダにあるMLModel.zipを交換するだけで良いのです。
こういうメンテナンスの事を考えて環境が出来上がっているのは、本当に助かりますね。
残る作業は、C#のクラスライブラリーからVB.net側へと結果を返す部分です。
この部分、実際のプログラムの運用を考えながら設計する必要があります。
まず、受渡した画像ファイルを判定した結果を返す必要があります。
これが出来ないと、判定した意味がありません。
もう一つ、重要な事があります。
判定結果の確率です。
正解率が90%の画像と、正解率55%の画像では処理を変える必要が出てきそうです。
例えば、コンクリートのひびなのか怪しい場合、人間に判断を求めるなど、運用時に必要になってきそうです。
とりあえず、2つの戻り値をC#からVB.netへ戻さなければなりません。
片方は簡単ですね、文字列の受け渡しだけですから。
もう片方の確率をどう処理すればよいのか、正直悩みましたが良い方法がありました。
一番数値の大きい割合と判定されているので、単純にpredictionResult.Score[]で一番大きな数値を受け渡せばよいのです。
例によってソースコードを記載します。
このコード、以下のWebアドレスに掲載されているものを基本にしています。
https://docs.microsoft.com/ja-jp/dotnet/machine-learning/
印刷して学びたい場合もPDFファイルがあるので参考になります。
https://docs.microsoft.com/ja-jp/dotnet/opbuildpdf/machine-learning/toc.pdf?branch=live
#学習 #勉強 #AI #機械学習 #プログラミング #Visual #CSharp #BASIC #ソースコード #Windows #自動判定
#画像 #判定 #検査
VB.net側のソースコード
Imports System.Reflection
Imports System.Windows.Forms
Imports System.IO
Imports System.Text
Imports Microsoft.VisualBasic.FileIO
Imports System.Globalization
Public Class Form1
Private Sub Form1_Load(sender As Object, e As EventArgs) Handles MyBase.Load
'クラスライブラリーを使う宣言
Dim ClassLibraryObject As Object = New ClassLibrary1.Class1
'写真を入力するフォルダの指定
'このフォルダ指定は自分の環境にあったものにしてください。
Dim SpecifyFileToInputPhoto As String = "C:\MLNET\data\assets\CD\7001-115.jpg"
'判別結果の格納
Dim JudgmentResult As String = ""
Dim Probability As Single = 0
'C#のクラスライブラリーで画像の判定を行わせる
Dim BooleanReturn As Boolean = ClassLibraryObject.SampleMachineLearning(SpecifyFileToInputPhoto, JudgmentResult, Probability)
End Sub
End Class
C#側のクラスライブラリーのコードです。
using System;
using System.Collections.Generic;
using System.Linq;
using System.IO;
using Microsoft.ML;
using static Microsoft.ML.DataOperationsCatalog;
using Microsoft.ML.Vision;
namespace ClassLibrary1
{
public class Class1
{
public void SampleMachineLearning(string SpecifyFileToInputPhoto,ref string JudgmentResult, ref float Probability)
{
// Create single instance of sample data from first line of dataset for model input
MlTestML.Model.ModelInput sampleData = new MlTestML.Model.ModelInput()
{
ImageSource = SpecifyFileToInputPhoto,
};
// Make a single prediction on the sample data and print results
var predictionResult = MlTestML.Model.ConsumeModel.Predict(sampleData);
Console.WriteLine("Using model to make single prediction -- Comparing actual Label with predicted Label from sample data...\n\n");
Console.WriteLine($"ImageSource: {sampleData.ImageSource}");
Console.WriteLine($"\n\nPredicted Label value {predictionResult.Prediction} \nPredicted Label scores: [{String.Join(",", predictionResult.Score)}]\n\n");
Console.WriteLine("=============== End of process, hit any key to finish ===============");
//Console.ReadKey();
JudgmentResult = predictionResult.Prediction;
Probability = 0;
for (int i = 0; i < predictionResult.Score.Length; i++)
{
if (Probability < predictionResult.Score[i])
{
Probability = predictionResult.Score[i];
}
}
}
}