アセットマネージャーのためのファイナンス機械学習:ポートフォリオの構築 練習問題 共分散行列のサイズによるNCOの性能
共分散行列のサイズを、10、100としてNCOを使った最少分散ポートフォリオと最大シャープレシオポートフォリオを計算する。
サイズ10の共分散行列
nBlocks, bSize, bCorr = 2, 5, .5
np.random.seed(0)
mu0, cov0 = MP.formTrueMatrix(nBlocks, bSize, bCorr)
minVarPortf=True
w1, w1_d=PortExp(mu0=mu0,cov0=cov0,minVarPortf = minVarPortf)
w0 = MP.optPort(cov0, None if minVarPortf else mu0)
w0 = np.repeat(w0.T, w1.shape[0], axis=0)
print('minVar Markowitz RMSE: ',
round(np.mean((w1-w0).values.flatten()**2)**.5,4))
print('minVar NCO RMSE:',
round(np.mean((w1_d-w0).values.flatten()**2)**.5,4))
minVarPortf=False
w1, w1_d=PortExp(mu0=mu0,cov0=cov0,minVarPortf = minVarPortf)
w0 = MP.optPort(cov0, None if minVarPortf else mu0)
w0 = np.repeat(w0.T, w1.shape[0], axis=0)
print('maxSR Markowitz RMSE: ',
round(np.mean((w1-w0).values.flatten()**2)**.5,4))
print('maxSRt NCO RMSE:',
round(np.mean((w1_d-w0).values.flatten()**2)**.5,4))
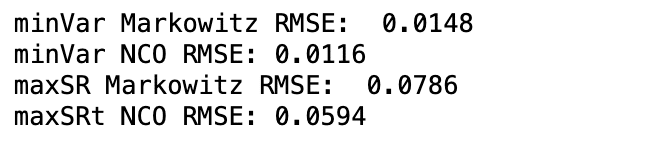
サイズ100の共分散行列
nBlocks, bSize, bCorr = 20, 5, .5
np.random.seed(0)
mu0, cov0 = MP.formTrueMatrix(nBlocks, bSize, bCorr)
minVarPortf=True
w1, w1_d=PortExp(mu0=mu0,cov0=cov0,minVarPortf = minVarPortf)
w0 = MP.optPort(cov0, None if minVarPortf else mu0)
w0 = np.repeat(w0.T, w1.shape[0], axis=0)
print('minVar Markowitz RMSE: ',
round(np.mean((w1-w0).values.flatten()**2)**.5,4))
print('minVar NCO RMSE:',
round(np.mean((w1_d-w0).values.flatten()**2)**.5,4))
minVarPortf=False
w1, w1_d=PortExp(mu0=mu0,cov0=cov0,minVarPortf = minVarPortf)
w0 = MP.optPort(cov0, None if minVarPortf else mu0)
w0 = np.repeat(w0.T, w1.shape[0], axis=0)
print('maxSR Markowitz RMSE: ',
round(np.mean((w1-w0).values.flatten()**2)**.5,4))
print('maxSRt NCO RMSE:',
round(np.mean((w1_d-w0).values.flatten()**2)**.5,4))
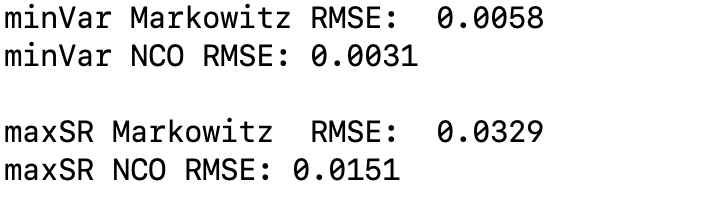
サイズに関係なく、NCOを使った方が二乗誤差が小さい。