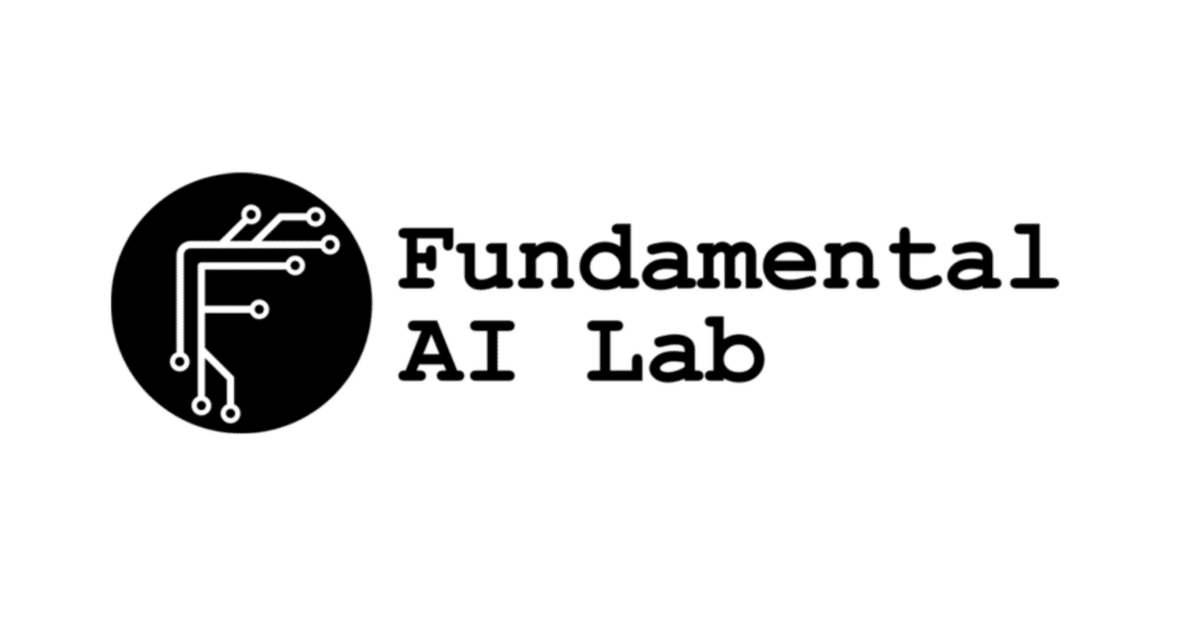
Towards trust and depth / 信頼と深みを目指して(by Yuki M. Asano)
English version / ※日本語訳は後半にあります
"ehhhm, I don't think so".
Those were my first thoughts when I got asked to write a blog entry for my dear collaborators from AIST on the topic of "Expanding Research Communities".
The reason was that the word "expanding" had this immediate connection to a development in our research field that I am finding quite annoying: the pressure to constantly expand. We constantly need to publish more papers and do more marketing on social media platforms – which, in turn, is made more difficult by the increasing number of papers that are coming out.
But of course I've expanded my research community. I was N=1 when I started my PhD, it grew to N=3 with my advisors Christian Rupprecht and Andrea Vedaldi from VGG, Oxford. It then grew to N=5 and more by working closely with my then fellow PhD comrade, Mandela Patrick. Eventually, it quickly grew to N>10 once I started supervising interdisciplinary AI projects in the OxAI society and supervising MSc students from Germany and Switzerland. Eventually, I became an assistant professor at Cees Snoek's large VISLab, which together with my own PhD students brought further collaboration opportunities and amazing connections. Now I work with colleagues from the Netherlands, France, Japan, US, UK and Italy.
Breadth vs Depth.
But the thing is: I have actually never counted the size of "my" community. Instead I was always focussed on the quality. I remember at one of my first conferences (after COVID it took a while for them to properly start), I met Kostas Derpanis and Andrei Bursuc, in-person. We've known each other online, but had never seen each other. We not only talked about research, the mountains, but also about living in multiple cultures. In short: we had a good chat as friends. Only much later did I start collaborating with Andrei on some research. I think there's something fundamental about first being friends and then doing research, and it has to do with trust.
Why Trust.
I believe that good research teams are much like good flight crews. While flying might, or might not, be more complicated than doing state-of-the-art AI research, they have figured out that everyone has the obligation to speak up when they have something important to say, e.g. when some safety critical thing happens -- no matter at which hierarchy level they are. This rule, which I generally try to introduce in new research teams by stating that "we're all researchers here, there's no titles, and your (the student's) opinion is as valid as any others".
However, there is something behind this principle that you need to make it work. And that's having established a level of trust. You need trust to have people speak up without fearing repercussions or feeling dumb. You need trust to have people propose (seemingly crazy) ideas*. You need trust in your co-authors to progress in your experiments and establish reproducibility. Trust fosters psychological safety, which in turn enables people to bring their full selves to the table. I believe that that's when the best work happens.
*On crazy ideas: Maybe I’ll write another extended article on this point in a while, but here’s some thoughts. I think as scientists we are obliged to use our minds towards thinking the yet-unthought. Yet in publications we need to measure against benchmarks and prior works. So I often get asked how to balance these two factors. I don’t have a perfect answer. But I do think that trying to go for research ideas that (when they work) lead to big surprises is a good intuition to follow: 1) Either make something work in a way that no one has ever done before (e.g. learning deep representations from a single image or video, or object counting without any annotations at all), 2) Or do something much much better than prior works with clear and elegant solutions (e.g. reduce number of parameters by further 100x compared to LoRA). A good check is to tell a colleague or friend your idea and see what they think. If they’re not too surprised, chances are that it’s an obvious next step and maybe someone else is already working on it.
Building Trust.
I recently read** that trust can be established in four dimensions: Competence, Care, Character and Consistency.
Competency is the sort of trust you have in a person when you value their opinions and they have prior experiences and work that they bring to the table. I believe this sort of trust is the one we're most familiar in research. But the other three are also, or even more, important.
Character: It's when the person you're working with has a strong moral compass and shares values with you. Values are what guides you when you need to make decisions that don't lend themselves to easy answers.
Consistency: It's when a person shows up when they say they will. In short: "to walk the talk".
Care: When we are putting our emotions into someone’s hands, we need to know they care and that they have our best interests in mind. We're not only researchers but actual humans!
** Jay Shetty. Think like a Monk. 2020
Conclusion.
Don't think about explicitly expanding your research community. Focus on depth rather than breadth whenever you can. Breadth will follow. Be aware that trust is required for great research, and work towards building it. Focus on finding people with whom you click. There's zero need to work with people where you feel uncomfortable.
About FunAI Lab.
I lead the Fundamental AI Lab (FunAI Lab) at the University of Technology Nuremberg (UTN). We collaborate widely and are always keen for new connections!
In terms of topics: we work on developing core technologies of current Foundation Models:
Self-supervised learning
Vision-language and multimodal learning
LLM reasoning and planning
Post-pretraining and instruction tuning
We put the fun into fundamental research.
日本語版
「えぇっと、それはどうかな……」。
研究連携している産総研(AIST)の研究者から「拡大する研究コミュニティ」とのトピックでブログ記事を書いて欲しいと依頼されたときの私の正直なファーストインプレッションです。
…というのも「拡大」という言葉から、私の属する研究分野において進行するある現象「常に拡大を求められるプレッシャー」をすぐに連想したからです。私たちは常により多くの論文を投稿・発表し、SNSでの広報活動を強化する必要があります。一方、発表論文の数が単調増加しているため、SNSで広報したとしても目立つことがより困難になっています。
そうは言っても、実のところ私もこれまで身の回りの研究コミュニティを拡大してきました。博士課程を始めた当初は自分ひとり(N=1)でしたが、オックスフォード大学 VGG の指導教員 Christian RupprechtとAndrea Vedaldi が加わりN=3となりました。その後、博士課程友達のMandela Patrickと密にコラボして研究を拡大することで N=5 以上に増加しました。さらに、OxAI Societyにおける学際的なAIプロジェクトの中でのメンタリング、ドイツやスイスからの修士課程学生をメンタリングするようになったことで、一気にN>10へと拡大したのです。最終的にはアムステルダム大学のCees Snoekが率いる大規模ラボ VISLabで助教授(Assistant Professor)に着任、私が受け持つ博士課程の学生含め、なおも多くのコラボレーション・素晴らしい繋がりを持つことができたのです。今では、オランダ、フランス、日本、アメリカ、イギリス、イタリアの研究者仲間とコラボしています。
幅広さ vs 深み
ただこの点において、実のところ「私の」研究コミュニティの規模を繋がりとして数えたことがないです。それよりは常に「質」に注目します。コロナ禍もありしばらく対面で国際会議がなく、その後に物理的に開催されたのある国際会議でKostas DerpanisとAndrei Bursucに初めて直接会ったことを覚えています。オンラインで交流はあったものの、直接対面はその際が初めてでした。研究の話はもちろん、登山話や複数文化圏内において暮らすことなどについて議論し、詰まるところ友人として楽しく会話したのです。そしてAndrei とは後に共同研究を行なっています。まず友人としての関係があり、その後に研究連携をするというアプローチには根源的な意義があると感じています。それは「信頼」と大きく関わっていると感じます。
なぜ信頼が重要なのか
優れた研究チームというのは良いフライトクルー(flight crews)に似ています。フライトが最先端のAI研究よりも複雑かどうかは私にはわかりませんが、フライトクルーの世界では、例として安全上の重要事項が発生したとき、立場に関係なく遠慮せずコメントする責任がある、というルールが確立されています。私が新規に研究チームに参加する際によく「我々は皆研究者で、肩書きは関係ありません。学生の意見だとしても他の研究者と同じくらい尊重されます」と伝え、このルールの共有を試みています。
ただし、この原則をうまく機能させるため、その裏付けが必要です。それこそが「信頼」です。信頼があれば、人々は報復や「バカだと思われるのでは」という恐れを感じることなく発言できるようになります。信頼があれば、(一見突拍子もないアイデアでも; seemingly crazy ideas (※1))気軽に提案できるようになります。信頼があれば、共著者たちと協力して実験を進め、再現性を確立できます。信頼があるからこそ心理的安全性が生じ、メンバーが自分の能力を遺憾無く発揮できるのです。そういう環境でこそ最高の研究が生まれると確信しています。
(※1) Crazy ideas: この点、近いうちに詳細な記事を出そうと思っていますが、ここでひとまず考えを共有します。研究者としては、誰も思いついていない思考から研究を実施する義務があると考えます。一方、論文発表の際、先行研究やベンチマークと比較する必要があり、よく双方どうバランスさせるか質問されます。実際のところ完璧な答えを持っていません。それでも「成功時」に大きな驚きを与え得る研究アイデアを目指すというのは、良い直感です。具体例として、1) これまで誰もやったことのない方法で何かを実現(例:単一画像や動画から深層表現学習する、一切教師ラベルなしで物体数を当てる、など)、2)あるいは先行研究を遥かに上回る性能を、明確で洗練された解法により達成(例:LoRAと比較してパラメータ数をさらに100倍削減、など)。研究アイデアを同僚や仲間と議論して反応を見るのは良い確認方法でしょう。仮にあまり驚かれないなら、すでに誰かが取り組んでいるか、または研究の流れとして順当なステップの可能性が高いです。
信頼を築くために
最近読んだ本 (※2) から、信頼とは以下の4つの次元で構築されるとのことです。
能力(Competency): 相手の意見に価値を認め、過去の経験や実績を信頼できること。研究の場面では、私たちが最も馴染みのある信頼の形だと思いますが、他の3つも同様に重要と考えます。
品性(Character): 倫理観がしっかりしていて、価値観を共有できること。価値観は、容易に答えが出ない決断を迫られたときの羅針盤になります。
一貫性(Consistency): 言ったことをやり遂げる姿勢、いわゆる“言行一致”であること。
思いやり(Care): 自分の感情を預ける相手が、相手を本当に気遣い、我々にとって最善を考えていると信じられること。私たちは研究者である前に一人の人間でもあります。
(※2) Jay Shetty. Think like a Monk. 2020
結論
上記の通りで、研究コミュニティを「拡大」することをわざわざ意識しなくても大丈夫です。可能な限り、広がりよりも深みに集中してください。広がりは後から自然についてきます。そして、素晴らしい研究には信頼が欠かせないことを理解し、その構築に努めてください。自分が「この人となら気が合う」と感じられる人を見つけることに集中しましょう。一緒にいて居心地が良くない人とわざわざ研究する必要はありません。
FunAI Labについて
私は、ニュルンベルク工科大学(University of Technology Nuremberg; UTN)でFundamental AI Lab(FunAI Lab)をリードしています。私たちは幅広くコラボしており、新しいつながりを常に歓迎しています!
研究トピックについて:現在の基盤モデル(Foundation Models)に関わるコア技術の開発を主な目的として、以下のようなトピックを扱っています。
Self-supervised learning(自己教師あり学習)
視覚と言語やマルチモーダル学習(Vision-language and multimodal learning)
大規模言語モデルの推論と計画(LLM reasoning and planning)
Post-pretraining and Instruction tuning
我々は 基礎研究(Fundamental Research)に楽しさ(Fun)のエッセンスを加えます。