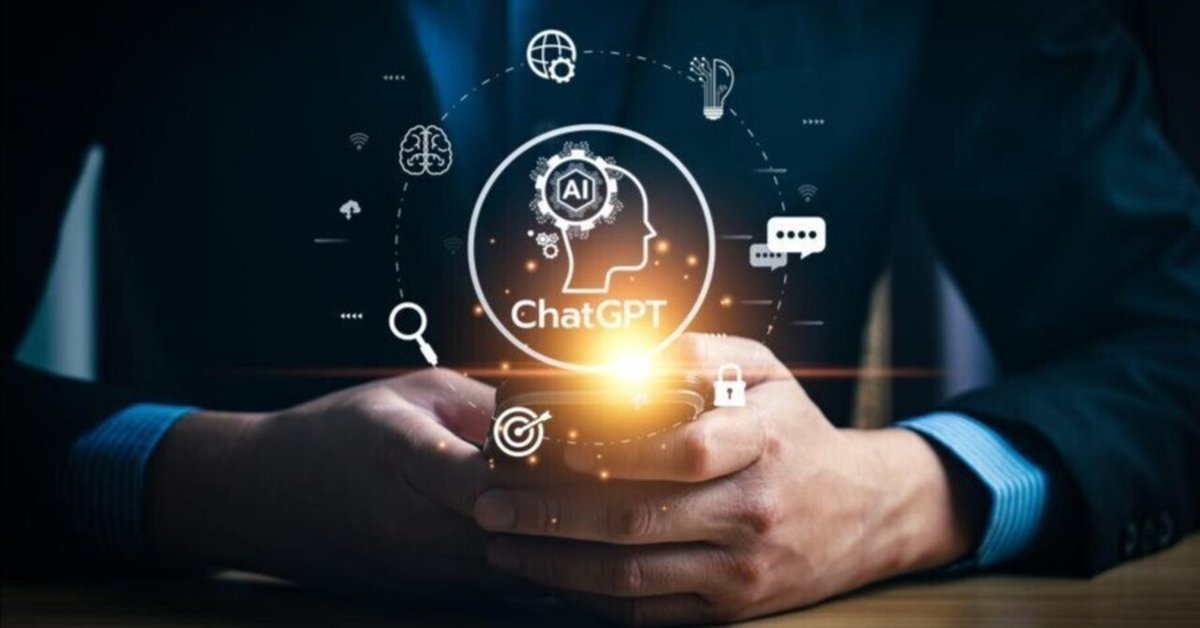
【English Composition Practice Book】What does ChatGPT do and why does it work?
OpenAI's interactive AI, ChatGPT, can answer questions from humans in a very natural way.
An overview of what happens inside ChatGPT - how it generates natural sentences and why it works so well - is given by theoretical physicist Stephen Wolfram, CEO of software company Wolfram Research.
Wolfram first explains that ‘what ChatGPT is always and fundamentally trying to do is to create a “rational continuation” of the text we have got so far’.
Here, ‘reasonable continuation’ means what people expect when they read a text, and how they expect someone else to write what they write next.
By scanning billions of texts on the web, ChatGPT predicts the probability that ‘when a sentence is written, what sentence will be written next’.
For example, given the sentence ‘The best thing about AI is its ability to’, ChatGPT searches the scanned text for sentences that are related to this sentence and ranks those that match the meaning according to their ‘probability’.
The ‘ranking of the next word’ is then used to rank the words that match the meaning of the sentence.
This ‘ranking of subsequent words’ is repeated to produce a sentence, but according to Wolfram, the highest-ranked word is not always selected.
This is because always selecting the highest-ranked words results in flat sentences with no creativity, so lower-ranked words are sometimes selected as a ‘daring breakdown’.
Therefore, even if the same prompt is used multiple times, it is more likely that different answers will be returned each time.
A similar ChatGPT mechanism was also noted by David Smardon, Assistant Professor of Economics at the University of Queensland and a chess grandmaster.
According to Mr Smardon, ChatGPT works broadly by ‘predicting the most likely word to come next from the start of a sentence’, so that when asked a factual question, people often make up a non-existent bullshit answer by focusing only on likely word combinations.
Often, the system is said to be able to predict the most likely words that will come next.
Wolfram continues by explaining how ChatGPT generates sentences by ‘ranking the words that follow’.
To obtain a probability table for ranking, ChatGPT first acquires an underlying language model neural network.
It then applies the acquired network model to the text and finds the top five most probable words that follow next according to the model.
This is repeated to add words with high probability to the text.
By varying the degree of randomness in word selection, different texts are output instead of always selecting the top words.
Wolfram gives a more detailed example of how the system ‘selects the next most likely word’.
If you take a sample of the English used within a Wikipedia article on ‘cat’ and ‘dog’, you can calculate the frequency of occurrence of the letters.
Generating a series of letters according to the extracted frequencies and separating them word by word with a specific probability will result in a somewhat presentable form, but it will not be something that can be read as a real word if you just select letters at random.
Add here the probability of a ‘pair of letters’ in a typical English text.
Here, for example, we see that when the letter ‘q’ arrives, the probability of a pair of letters is zero, except for the letter ‘u’.
Generating words in this way by looking at two letters at a time allows a sentence that was completely unreadable to contain a word that actually exists.
Furthermore, in the same way, estimates can be obtained for the ‘combination probability’ for long stretches of letters as well as ‘pairs’, provided there is a sufficient amount of text.
The text then becomes more realistic, even when random words are generated.
Similarly, ChatGPT uses whole words, rather than characters, to estimate ‘how commonly a word is used’ from a large textual data set, and generates sentences where each word is individually selected at random.
However, here, as with word generation from characters, probability alone does not generate sentences that make sense.
Therefore, here too, the probability of a ‘word pair’ or combination of words is taken into account, in the same way as for letters, in order to get closer to a plausible sentence.
Thus, Wolfram explains ‘what ChatGPT does’, but states that it is difficult to explain ‘how it works’.
For example, he said that while it is understandable how difficult it is for a neural network to attempt to recognise an image of a cat, there is no concrete way to explain the actual process taking place within the network, as it also involves a computational black box.
According to Wolfram, ChatGPT is a massive neural network with 175 billion weights, and its main feature is Google's Transformer neural network architecture, which excels at language understanding tasks.
Transformer was developed as a translation model, but it can map parameters such as images in a process that translates them in the same way as language, allowing it to ‘modularise’ things by introducing concepts such as ‘paying more attention’ to some parts of a sequence than others The.
You can read more about how Transformer has created breakthroughs in machine learning in the following article.
Based on the above, Wolfram describes how ChatGPT works in practice in three stages.
First, it takes a series of tokens corresponding to conventional text and finds the rules corresponding to these as an array of numbers.
Next, the rules are manipulated in the ‘standard neural net way’ and the values ‘spill over’ through successive layers in the network, generating new rules.
This rule is then taken and an array of about 50,000 values is generated from it.
This array becomes a probability of the various token possibilities, so the probability of combining words is derived.
According to Wolfram, all of these mechanisms are implemented by neural networks, and since everything is only learnt from training data, nothing is explicitly designed, except the entire architecture.
However, the design of the entire architecture reflects all kinds of experience and knowledge of neural networks.
The way the architecture works is that it first converts the myriad of input tokens into ‘embedded vectors’, and the ‘pay attention’ function, which is a key feature of Transformer, allows it to ‘look back with attention’ at a series of texts to understand word combinations and to establish overall unity.
After passing through these attentional processes, the Transformer converts a series of tokens into a final collection, so ChatGPT takes the collection, decodes it and creates it as a probability list of words that come next.
This is how ChatGPT works in outline, with Wolfram explaining that ‘it may seem complex, but in reality it is made up of simple elements - just a neural network taking a collection of numerical inputs and combining them with certain weights to make a list He explains that ‘it's a very simple thing to do’.
Finally, Wolfram says: ‘What is finally remarkable is that all these operations can somehow work together to perform the excellent and human-like task of generating text. It needs to be reiterated that, at least as far as we know, there is no ‘ultimate theoretical reason’ for this stuff to work’, he added: ’And this can be seen as a scientific discovery: in a neural network like ChatGPT, the human brain can may be able to capture the essence of what the human brain does to produce language’.
【参考図書】
「日本人のための英作文練習帳」酒井文秀(著)GregoryAlanDick(英文監修)
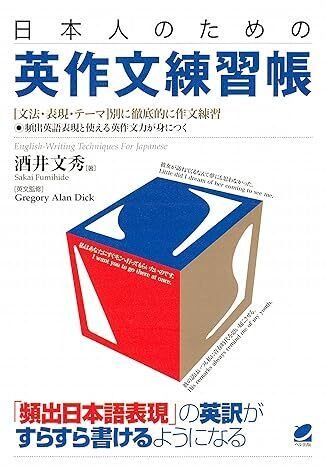
「[必修編] 英作文のトレーニング」Z会編集部(編)
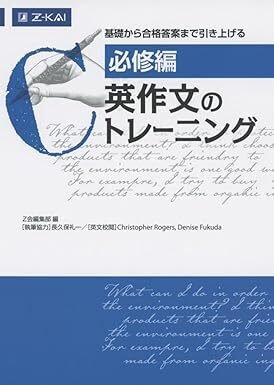
「自由英作文編 英作文のトレーニング 改訂版」成田あゆみ(著)
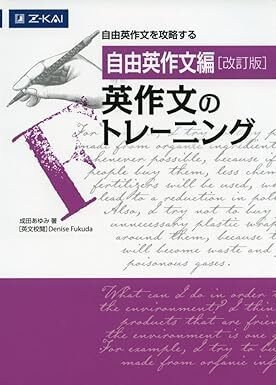
「実戦編 英作文のトレーニング 改訂版」Z会編集部(編)
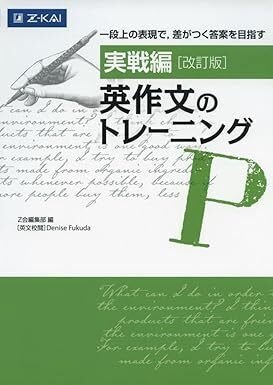
「[はじめる編] 英作文のトレーニング 新装版」渡辺寿郎(著)
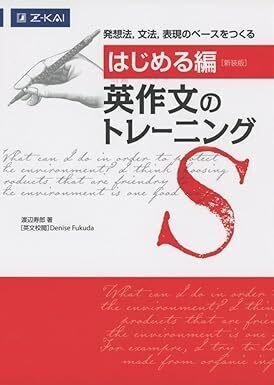