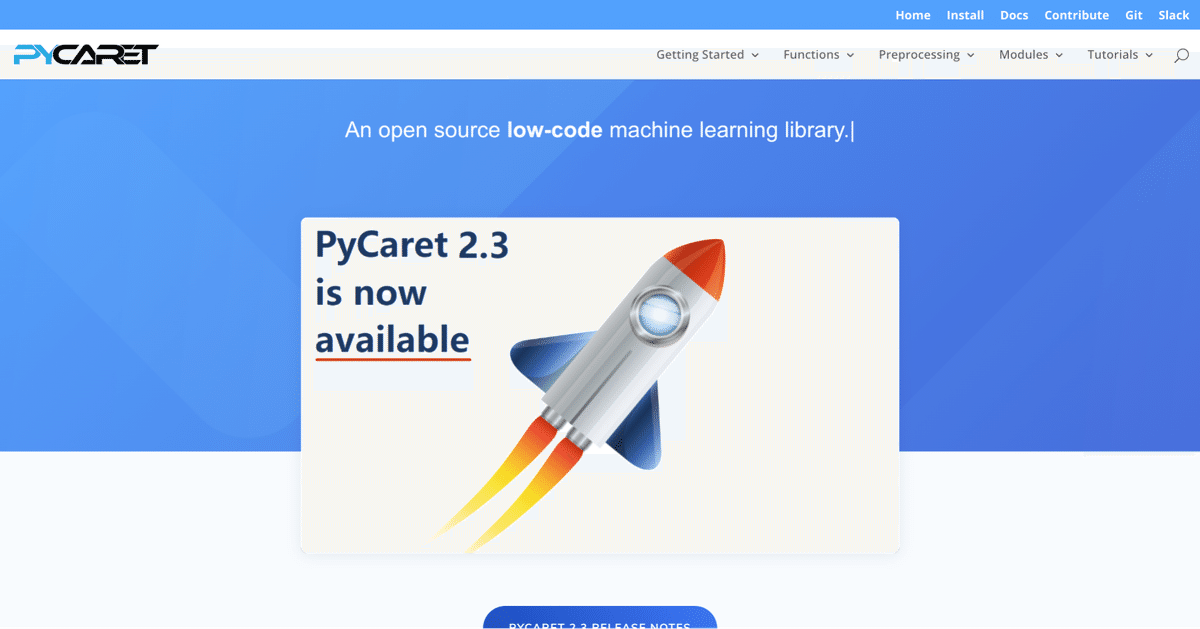
超簡単Pythonで株価予測(PyCaret 利用)自動機械学習(AutoML)-アンサンブル-
PythonでPyCaretを利用して翌日の株価の上下予測を超簡単に自動機械学習(AutoML)アンサンブル(Voting・バギング・スタッキング)
1. ツールインストール
$ pip install pycaret yfinance
2. ファイル作成(バギング)
bagging.py
import yfinance as yf
from sklearn.model_selection import train_test_split
from pycaret.classification import *
from sklearn.metrics import accuracy_score
df = yf.download("AAPL", start="2010-11-01", end="2020-11-01")
df["Diff"] = df.Close.diff()
df["SMA_2"] = df.Close.rolling(2).mean()
df["Force_Index"] = df["Close"] * df["Volume"]
df["y"] = df["Diff"].apply(lambda x: 1 if x > 0 else 0).shift(-1)
df = df.drop(
["Open", "High", "Low", "Close", "Volume", "Diff", "Adj Close"],
axis=1,
).dropna()
# print(df)
train, test = train_test_split(
df,
test_size=0.2,
shuffle=False,
)
setup(data = train, target = "y", silent = True) # 前処理
best_model = compare_models() # 複数の機械学習モデルからベストなモデルを選択
tuned_model = tune_model(best_model) # ハイパーパラメータ自動調整
bagged_model = ensemble_model(tuned_model, method = "Bagging") # バギング
pred = predict_model(bagged_model, data = test) # 予測
print(accuracy_score(pred.y.values, pred.Label.astype(float).values))
3. 実行(バギング)
$ python bagging.py
0.5496031746031746
4. ファイル作成(Voting)
voting.py
import yfinance as yf
from sklearn.model_selection import train_test_split
from pycaret.classification import *
from sklearn.metrics import accuracy_score
df = yf.download("AAPL", start="2010-11-01", end="2020-11-01")
df["Diff"] = df.Close.diff()
df["SMA_2"] = df.Close.rolling(2).mean()
df["Force_Index"] = df["Close"] * df["Volume"]
df["y"] = df["Diff"].apply(lambda x: 1 if x > 0 else 0).shift(-1)
df = df.drop(
["Open", "High", "Low", "Close", "Volume", "Diff", "Adj Close"],
axis=1,
).dropna()
# print(df)
train, test = train_test_split(
df,
test_size=0.2,
shuffle=False,
)
setup(data = train, target = "y", silent = True) # 前処理
top3 = compare_models(n_select = 3) # 複数の機械学習モデルからトップ3モデルを選択
tuned_top3 = [tune_model(i) for i in top3] # ハイパーパラメータ自動調整
voted_model = blend_models(tuned_top3) # Voting
pred = predict_model(voted_model, data = test) # 予測
print(accuracy_score(pred.y.values, pred.Label.astype(float).values))
5. 実行(Voting)
$ python voting.py
0.5535714285714286
6. ファイル作成(スタッキング)
stacking.py
import yfinance as yf
from sklearn.model_selection import train_test_split
from pycaret.classification import *
from sklearn.metrics import accuracy_score
df = yf.download("AAPL", start="2010-11-01", end="2020-11-01")
df["Diff"] = df.Close.diff()
df["SMA_2"] = df.Close.rolling(2).mean()
df["Force_Index"] = df["Close"] * df["Volume"]
df["y"] = df["Diff"].apply(lambda x: 1 if x > 0 else 0).shift(-1)
df = df.drop(
["Open", "High", "Low", "Close", "Volume", "Diff", "Adj Close"],
axis=1,
).dropna()
# print(df)
train, test = train_test_split(
df,
test_size=0.2,
shuffle=False,
)
setup(data = train, target = "y", silent = True) # 前処理
top3 = compare_models(n_select = 3) # 複数の機械学習モデルからトップ3モデルを選択
tuned_top3 = [tune_model(i) for i in top3] # ハイパーパラメータ自動調整
stacked_model = stack_models(tuned_top3) # スタッキング
pred = predict_model(stacked_model, data = test) # 予測
print(accuracy_score(pred.y.values, pred.Label.astype(float).values))
7. 実行(スタッキング)
$ python stacking.py
0.5496031746031746
以上、超簡単!
8. 結果
同じデータ、特徴量で、計算した結果、PyCaret・PyCaret(bagging)・PyCaret(voting)・PyCaret(stacking)・TPOT・TPOT(NN)・Auto-sklearn・AutoGluon・AutoKeras・FLAMLのうちTPOTが最も良いという事に
PyCaret 0.5178571428571429
PyCaret(bagging) 0.5496031746031746
PyCaret(voting) 0.5535714285714286
PyCaret(stacking) 0.5496031746031746
TPOT 0.5555555555555556
TPOT(NN) 0.503968253968254
Auto-sklearn 0.5198412698412699
AutoGluon 0.5496031746031746
AutoKeras 0.4861111111111111
FLAML 0.5277777777777778