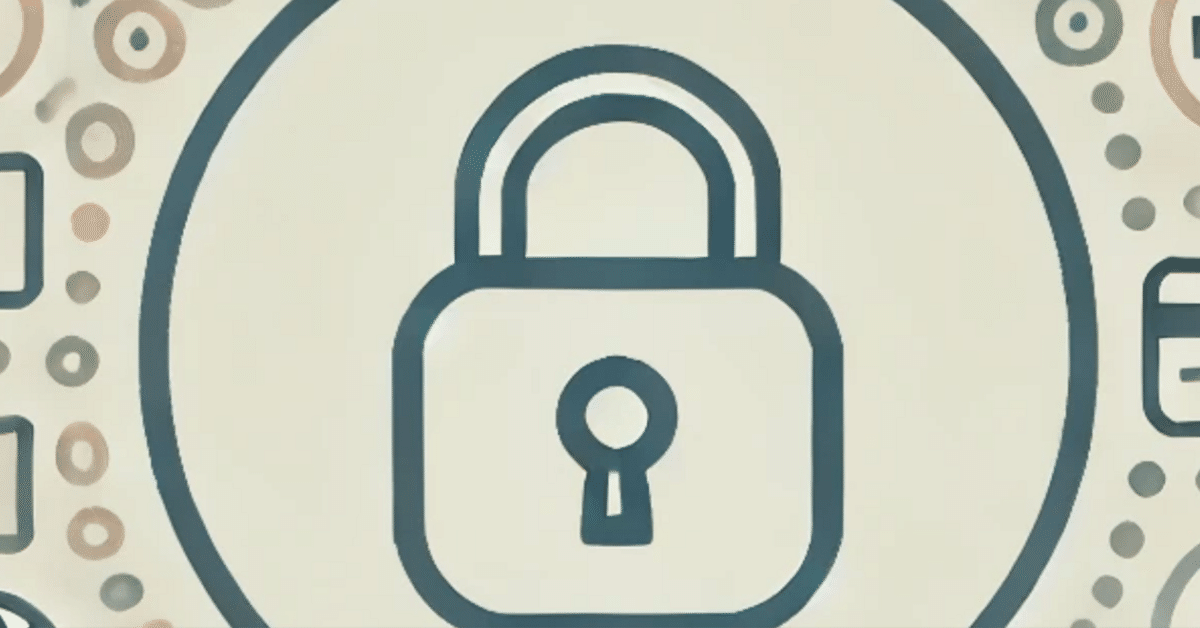
AIガードレール:PII(個人情報)の検出を理解する
個人情報(PII)は、数多くのデジタルサービスやプラットフォームの中心的な存在となっています。データが国境を越えて自由に行き交う中、PIIのための明確なガードレールを確立することがこれまで以上に重要になっています。これらのガードレールは、法的要件、倫理的配慮に基づいて構築され、氏名、メールアドレス、電話番号、金融情報、健康記録などの機密データを保護する役割を果たします。
以下では、PIIの基本を掘り下げ、それを保護するために設計・実装されたガードレールを探ります。ガードレールの実装を確認したい場合は、こちらのColabをご覧ください。
PIIとは何か?
PIIは、名前やメールアドレスから財務情報に至るまで、特定の個人を識別するデータのことを指します。PIIを保護するためのガードレール(法的、倫理的、技術的な仕組み)は、銀行業界、医療、教育などの分野で機密情報が安全に保たれることを保証します。
PIIに関する制限は、個人情報データを収集、保存、処理する際の安全性とプライバシーを保護するための倫理的・組織的な指針の枠組みです。これらの制限は地域や業界によって異なりますが、一般的には、PIIの利用を正当な目的に限定し、不正なアクセス、悪用、または漏洩を防止することを目的としています。
例えば、EUの 一般データ保護規則(GDPR) の下では、PIIの取り扱いは厳格に規制されています。GDPRでは、データは特定の明確かつ正当な目的のためにのみ収集および処理されるべきであり、データ収集前に個人の明示的な同意を得ることが義務付けられています。また、特に国際的なデータ移転において、第三者へのデータ共有や転送に厳しい制限を課しています。さらに、組織には、仮名化や暗号化などの手法を用いて機密データを保護すること、ならびに個人が自分のデータにアクセスし、修正し、削除を要求する権利を提供することが求められています。
一方、米国では、PIIの制約は文脈や情報の種類に依存します。例えば、医療保険の携行性と責任に関する法律(HIPAA) は、医療関連のPII(保護対象医療情報:PHI)の取り扱いを医療提供者に制限し、不正な開示から情報を保護することを求めています。同様に、児童オンラインプライバシー保護法(COPPA) では、13歳未満の子供から収集したデータの取り扱いについて厳しい規制を設けており、親の同意を重視し、データ保持を制限しています。
また、多くの業界が独自の基準を採用してPII制限を強化しています。例えば、金融業界では PCI DSS(Payment Card Industry Data Security Standard) に準拠し、支払いおよび口座情報を保護しています。米国の教育機関では、家族教育権とプライバシー法(FERPA) に従い、学生のデータを保護しています。これらの枠組みは、通常、組織に対して堅牢なアクセス制御の実施、データ運用の定期的な監査、データプライバシーに関する従業員への教育を求めています。
PIIガードレールが重要な理由
必要最低限のデータ保持、ユーザーの同意、厳格なセキュリティ対策を求めることで、PIIガードレールはデータ漏洩や不正な開示のリスクを軽減します。また、個人に自身のデータへのアクセス・修正・削除の権利を保証することで、信頼を確保します。
PIIの重要性
PIIの重要性は、プライバシーとセキュリティに密接に結びついています。PIIが適切に扱われることで、ユーザーがパーソナライズされたサービスを利用でき、組織がより効率的に運営することを可能にします。しかし、PIIの誤用や不適切な取り扱いは、プライバシー侵害、金銭的損失、精神的な苦痛など、深刻な結果を招く可能性があります。サイバー犯罪者は、アイデンティティ盗用や詐欺を目的にPIIを標的にし、システムの脆弱性やデータ漏洩を利用して機密データに不正にアクセスします。
世界各地の法的枠組み、たとえばヨーロッパの一般データ保護規則(GDPR) や米国のカリフォルニア州消費者プライバシー法(CCPA) は、組織がPIIを責任を持って収集、保存、処理することを確保するために制定されています。医療分野においては、米国の 医療保険の携行性と責任に関する法律(HIPAA) が、保護対象医療情報(PHI)と呼ばれる健康関連のPIIを保護するための厳格な基準を定めています。HIPAAは、医療提供者、保険会社、およびそのビジネスパートナーに対し、医療記録やその他の健康情報を保護するための措置を求め、これらの機密性、完全性、および可用性を確保します。HIPAAの違反は、厳しい罰則を招くだけでなく、患者の信頼を損なう恐れがあります。
社会がデジタルプラットフォームにますます依存する中で、PIIを保護することは単なる規制上の要件ではなく、倫理的な責任でもあります。PIIの価値と脆弱性を認識することで、組織はこの機密情報を保護するために積極的な対策を講じることができ、データ駆動型の世界における安全性を高めることができます。
PII検出ガードレールチュートリアル
PIIを検出し保護することは、コンプライアンスの維持、ユーザーのプライバシー保護、そして責任あるデータ運用を確保するための重要なステップとなっています。このチュートリアルでは、単純な正規表現ベースの検出から高度なAIを活用した手法に至るまで、さまざまなPII検出ガードレールを設定し、テキスト内のPIIを自動的に特定および処理する方法を紹介します。
まず最初に、以下のコマンドを使用してsafeguardsライブラリをインストールしてください:
git clone https://github.com/soumik12345/safeguards.git && cd safeguards && pip install -e .
正規表現ベースのPII検出ガードレール
RegexEntityRecognitionGuardrailは、事前定義されたパターンを使用してテキスト内のPIIを識別します。この単純なガードレールは、電話番号やメールアドレスなどの構造化されたデータに対して効果的に機能し、高い解釈性と簡単な設定が可能です。しかし、その固定的な性質のため、テキスト内の特殊なケースやバリエーションに対応するのは難しい場合があります。
Weaveを使用すると、検出されたエンティティをログに記録し、視覚化することでガードレールのパフォーマンスをより深く理解できます。通常、追跡したい関数の上に@Weave.opデコレータを使用する必要がありますが、safeguardsライブラリにはネイティブの統合機能があるため、Weaveをインポートして初期化するだけで、結果が自動的に追跡されます。
from safeguards.guardrails.entity_recognition import RegexEntityRecognitionGuardrail
import weave; weave.init("guardrails-pii")
# Define hardcoded sample data
test_cases = [
{
"input_text": "Contact me at john.doe@example.com or call me at (123) 456-7890.",
"expected_entities": {
"EMAIL": ["john.doe@example.com"],
"TELEPHONENUM": ["(123) 456-7890"],
},
},
{
"input_text": "My SSN is 123-45-6789, and my credit card is 4111-1111-1111-1111.",
"expected_entities": {
"SOCIALNUM": ["123-45-6789"],
"CREDITCARDNUMBER": ["4111-1111-1111-1111"],
},
},
]
# Initialize the regex-based guardrail
regex_guardrail = RegexEntityRecognitionGuardrail(should_anonymize=True)
# Process each test case
for i, case in enumerate(test_cases, 1):
try:
# Use the `guard` method for PII detection
result = regex_guardrail.guard(case["input_text"])
print(f"Test Case {i}")
print(f"Input: {case['input_text']}")
print(f"Expected Entities: {case['expected_entities']}")
print(f"Detected Entities: {result}\n")
except AttributeError as e:
print(f"Error processing Test Case {i}: {e}")
RegexEntityRecognitionGuardrailは、メールアドレスや電話番号などの典型的なPIIを含むテキストサンプルに対して初期化され、適用されます。guardメソッドは、正規表現パターンに基づいて検出されたエンティティを返し、それらはWeaveに記録されます。これにより、ガードレールが期待通りに機能した箇所と、エンティティの検出に失敗した箇所を簡単に確認することが可能です。この手法は固定されたフォーマットに依存しているため、複雑なテキストに対する適応性に限界があります。
PresidioベースのPII検出ガードレール
PresidioEntityRecognitionGuardrailは、MicrosoftのPresidioフレームワークを基盤として構築されており、正規表現ルールと文脈認識型の検出機能を組み合わせています。このPIIガードレールは、正規表現のみの場合よりも適応性が高く、フォーマットが多少異なる場合でもエンティティを認識できます。以下にコードを示します:
from safeguards.guardrails.entity_recognition import PresidioEntityRecognitionGuardrail
import weave; weave.init("guardrails-pii")
# Define hardcoded sample data
test_cases = [
{
"input_text": "Jane's email is jane.doe@gmail.com, and her phone is +1-800-555-1234.",
"expected_entities": {
"EMAIL_ADDRESS": ["jane.doe@gmail.com"],
"PHONE_NUMBER": ["+1-800-555-1234"],
},
},
{
"input_text": "My passport number is A12345678, and I live in New York.",
"expected_entities": {
"US_PASSPORT": ["A12345678"],
"LOCATION": ["New York"],
},
},
]
# Initialize the Presidio-based guardrail
presidio_guardrail = PresidioEntityRecognitionGuardrail(should_anonymize=True)
# Process each test case
for i, case in enumerate(test_cases, 1):
try:
# Use the `guard` method for PII detection
result = presidio_guardrail.guard(case["input_text"])
print(f"Test Case {i}")
print(f"Input: {case['input_text']}")
print(f"Expected Entities: {case['expected_entities']}")
print(f"Detected Entities: {result}\n")
except AttributeError as e:
print(f"Error processing Test Case {i}: {e}")
PresidioEntityRecognitionGuardrailは、メールアドレスやパスポート番号などのPIIを特定するためにテキストサンプルに適用されます。このガードレールは、エンティティのフォーマットにおけるバリエーションを効果的に処理し、検出されたエンティティのセットを返すと同時に、Weaveにログとして記録されます。正規表現に比べて柔軟性が向上していることが分かります。
TransformerベースのPII検出
TransformersEntityRecognitionGuardrailは、Transformerのような機械学習モデルを使用してテキスト内のPIIを識別します。このガードレールは文脈を理解し、非構造化データに適応する能力を持ち、複雑または微妙な状況において特に強力です。この手法では事前定義されたルールは必要なく、代わりに事前学習済みの言語モデルの能力に依存します。その出力をWeaveに記録することで、他の手法と比較しながら詳細なパフォーマンス分析が可能になります。
from safeguards.guardrails.entity_recognition import TransformersEntityRecognitionGuardrail
import weave; weave.init("guardrails-pii")
# Define hardcoded sample data
test_cases = [
{
"input_text": "My name is Brett Johnson, and my phone number is +1 987-654-3210.",
"expected_entities": {
"PERSON": ["Alice Johnson"],
"TELEPHONENUM": ["987-654-3210"],
},
},
{
"input_text": "The acc. # is 1234532289, and the credit card is 5555-5555-5555-5555.",
"expected_entities": {
"IP_ADDRESS": ["192.168.1.1"],
"CREDITCARDNUMBER": ["5555-5555-5555-5555"],
},
},
]
# Initialize the transformer-based guardrail
transformer_guardrail = TransformersEntityRecognitionGuardrail(should_anonymize=True)
# Process each test case
for i, case in enumerate(test_cases, 1):
try:
# Use the `guard` method for PII detection
result = transformer_guardrail.guard(case["input_text"])
print(f"Test Case {i}")
print(f"Input: {case['input_text']}")
print(f"Expected Entities: {case['expected_entities']}")
print(f"Detected Entities: {result}\n")
except AttributeError as e:
print(f"Error processing Test Case {i}: {e}")
TransformersEntityRecognitionGuardrailは、テキストサンプルを処理し、難しい文脈においても高い精度でPIIを検出します。たとえば、長い文の中に埋め込まれたエンティティや曖昧な構造を持つエンティティを正確に識別しました。検出されたエンティティはWeaveにログとして記録され、そのパフォーマンスを他のガードレールと比較するのが容易になります。以下は、スクリプトを実行した後のWeaveでの表示例です:
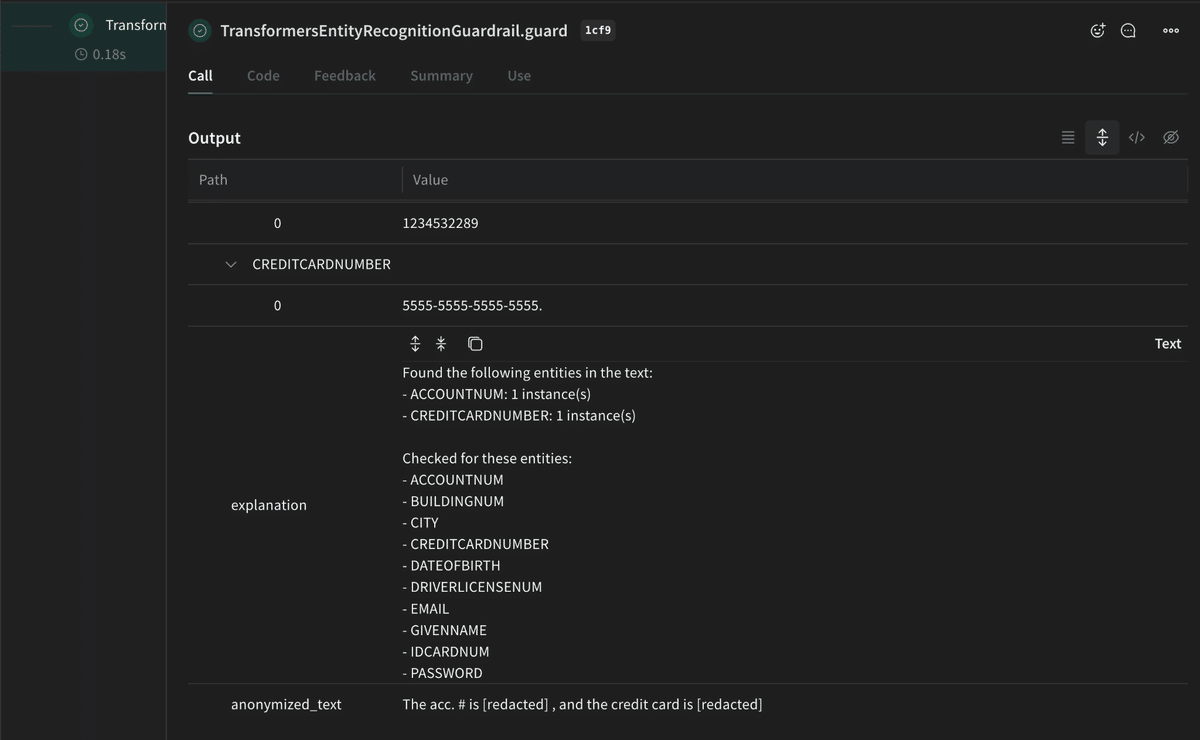
Weaveを用いたPIIガードレールの評価
各ガードレールでPIIを検出した後、そのパフォーマンスを体系的に評価することが重要です。Weaveの評価フレームワークを使用すると、検出されたエンティティや精度、再現率、F1スコアといった関連メトリクスを記録し、比較することでそれぞれの有効性を把握できます。これにより、異なる種類のデータに対する各ガードレールのパフォーマンスに関する具体的な洞察が得られ、特定のユースケースに最適な手法を特定するのに役立ちます。
今回評価するのは以下の3つのPII検出ガードレールです:
RegexEntityRecognitionGuardrail
PresidioEntityRecognitionGuardrail
TransformersEntityRecognitionGuardrail
目標は、これらのガードレールがデータセット全体でPIIエンティティをどの程度正確に識別できるかを評価することで、精度(Precision)、再現率(Recall)、F1スコアといったメトリクスを使用してパフォーマンスを測定します。以下は評価を実行するコードです:
import asyncio
import json
import random
from pathlib import Path
from typing import Dict, List, Optional
import weave
from datasets import load_dataset
from weave import Evaluation
from weave.scorers import Scorer
from safeguards.guardrails.entity_recognition import (
RegexEntityRecognitionGuardrail,
PresidioEntityRecognitionGuardrail,
TransformersEntityRecognitionGuardrail
)
# Add this mapping dictionary near the top of the file
PRESIDIO_TO_TRANSFORMER_MAPPING = {
"EMAIL_ADDRESS": "EMAIL",
"PHONE_NUMBER": "TELEPHONENUM",
"US_SSN": "SOCIALNUM",
"CREDIT_CARD": "CREDITCARDNUMBER",
"IP_ADDRESS": "IDCARDNUM",
"DATE_TIME": "DATEOFBIRTH",
"US_PASSPORT": "IDCARDNUM",
"US_DRIVER_LICENSE": "DRIVERLICENSENUM",
"US_BANK_NUMBER": "ACCOUNTNUM",
"LOCATION": "CITY",
"URL": "USERNAME", # URLs often contain usernames
"IN_PAN": "TAXNUM", # Indian Permanent Account Number
"UK_NHS": "IDCARDNUM",
"SG_NRIC_FIN": "IDCARDNUM",
"AU_ABN": "TAXNUM", # Australian Business Number
"AU_ACN": "TAXNUM", # Australian Company Number
"AU_TFN": "TAXNUM", # Australian Tax File Number
"AU_MEDICARE": "IDCARDNUM",
"IN_AADHAAR": "IDCARDNUM", # Indian national ID
"IN_VOTER": "IDCARDNUM",
"IN_PASSPORT": "IDCARDNUM",
"CRYPTO": "ACCOUNTNUM", # Cryptocurrency addresses
"IBAN_CODE": "ACCOUNTNUM",
"MEDICAL_LICENSE": "IDCARDNUM",
"IN_VEHICLE_REGISTRATION": "IDCARDNUM",
}
class EntityRecognitionScorer(Scorer):
"""Scorer for evaluating entity recognition performance"""
@weave.op()
async def score(
self, model_output: Optional[dict], input_text: str, expected_entities: Dict
) -> Dict:
"""Score entity recognition results"""
if not model_output:
return {"f1": 0.0}
# Convert Pydantic model to dict if necessary
if hasattr(model_output, "model_dump"):
model_output = model_output.model_dump()
elif hasattr(model_output, "dict"):
model_output = model_output.dict()
detected = model_output.get("detected_entities", {})
# Map Presidio entities if needed
if model_output.get("model_type") == "presidio":
mapped_detected = {}
for entity_type, values in detected.items():
mapped_type = PRESIDIO_TO_TRANSFORMER_MAPPING.get(entity_type)
if mapped_type:
if mapped_type not in mapped_detected:
mapped_detected[mapped_type] = []
mapped_detected[mapped_type].extend(values)
detected = mapped_detected
# Track entity-level metrics
all_entity_types = set(list(detected.keys()) + list(expected_entities.keys()))
entity_metrics = {}
for entity_type in all_entity_types:
detected_set = set(detected.get(entity_type, []))
expected_set = set(expected_entities.get(entity_type, []))
# Calculate metrics
true_positives = len(detected_set & expected_set)
false_positives = len(detected_set - expected_set)
false_negatives = len(expected_set - detected_set)
if entity_type not in entity_metrics:
entity_metrics[entity_type] = {
"total_true_positives": 0,
"total_false_positives": 0,
"total_false_negatives": 0,
}
entity_metrics[entity_type]["total_true_positives"] += true_positives
entity_metrics[entity_type]["total_false_positives"] += false_positives
entity_metrics[entity_type]["total_false_negatives"] += false_negatives
# Calculate per-entity metrics
precision = (
true_positives / (true_positives + false_positives)
if (true_positives + false_positives) > 0
else 0
)
recall = (
true_positives / (true_positives + false_negatives)
if (true_positives + false_negatives) > 0
else 0
)
f1 = (
2 * (precision * recall) / (precision + recall)
if (precision + recall) > 0
else 0
)
entity_metrics[entity_type].update(
{"precision": precision, "recall": recall, "f1": f1}
)
# Calculate overall metrics
total_tp = sum(
metrics["total_true_positives"] for metrics in entity_metrics.values()
)
total_fp = sum(
metrics["total_false_positives"] for metrics in entity_metrics.values()
)
total_fn = sum(
metrics["total_false_negatives"] for metrics in entity_metrics.values()
)
overall_precision = (
total_tp / (total_tp + total_fp) if (total_tp + total_fp) > 0 else 0
)
overall_recall = (
total_tp / (total_tp + total_fn) if (total_tp + total_fn) > 0 else 0
)
overall_f1 = (
2
* (overall_precision * overall_recall)
/ (overall_precision + overall_recall)
if (overall_precision + overall_recall) > 0
else 0
)
entity_metrics["overall"] = {
"precision": overall_precision,
"recall": overall_recall,
"f1": overall_f1,
"total_true_positives": total_tp,
"total_false_positives": total_fp,
"total_false_negatives": total_fn,
}
return entity_metrics["overall"]
def load_ai4privacy_dataset(
num_samples: int = 100, split: str = "validation"
) -> List[Dict]:
"""
Load and prepare samples from the ai4privacy dataset.
Args:
num_samples: Number of samples to evaluate
split: Dataset split to use ("train" or "validation")
Returns:
List of prepared test cases
"""
# Load the dataset
dataset = load_dataset("ai4privacy/pii-masking-400k")
# Get the specified split
data_split = dataset[split]
# Randomly sample entries if num_samples is less than total
if num_samples < len(data_split):
indices = random.sample(range(len(data_split)), num_samples)
samples = [data_split[i] for i in indices]
else:
samples = data_split
# Convert to test case format
test_cases = []
for sample in samples:
# Extract entities from privacy_mask
entities: Dict[str, List[str]] = {}
for entity in sample["privacy_mask"]:
label = entity["label"]
value = entity["value"]
if label not in entities:
entities[label] = []
entities[label].append(value)
test_case = {
"description": f"AI4Privacy Sample (ID: {sample['uid']})",
"input_text": sample["source_text"],
"expected_entities": entities,
"masked_text": sample["masked_text"],
"language": sample["language"],
"locale": sample["locale"],
}
test_cases.append(test_case)
return test_cases
def save_results(
weave_results: Dict, model_name: str, output_dir: str = "evaluation_results"
):
"""Save evaluation results to files"""
output_dir = Path(output_dir)
output_dir.mkdir(exist_ok=True)
# Extract and process results
scorer_results = weave_results.get("EntityRecognitionScorer", [])
if not scorer_results or all(r is None for r in scorer_results):
print(f"No valid results to save for {model_name}")
return
# Calculate summary metrics
total_samples = len(scorer_results)
passed = sum(1 for r in scorer_results if r is not None and not isinstance(r, str))
# Aggregate entity-level metrics
entity_metrics = {}
for result in scorer_results:
try:
if isinstance(result, str) or not result:
continue
for entity_type, metrics in result.items():
if entity_type not in entity_metrics:
entity_metrics[entity_type] = {
"precision": [],
"recall": [],
"f1": [],
}
entity_metrics[entity_type]["precision"].append(metrics["precision"])
entity_metrics[entity_type]["recall"].append(metrics["recall"])
entity_metrics[entity_type]["f1"].append(metrics["f1"])
except (AttributeError, TypeError, KeyError):
continue
# Calculate averages
summary_metrics = {
"total": total_samples,
"passed": passed,
"failed": total_samples - passed,
"success_rate": (passed / total_samples) if total_samples > 0 else 0,
"entity_metrics": {
entity_type: {
"precision": (
sum(metrics["precision"]) / len(metrics["precision"])
if metrics["precision"]
else 0
),
"recall": (
sum(metrics["recall"]) / len(metrics["recall"])
if metrics["recall"]
else 0
),
"f1": sum(metrics["f1"]) / len(metrics["f1"]) if metrics["f1"] else 0,
}
for entity_type, metrics in entity_metrics.items()
},
}
# Save files
with open(output_dir / f"{model_name}_metrics.json", "w") as f:
json.dump(summary_metrics, f, indent=2)
# Save detailed results, filtering out string results
detailed_results = [
r for r in scorer_results if not isinstance(r, str) and r is not None
]
with open(output_dir / f"{model_name}_detailed_results.json", "w") as f:
json.dump(detailed_results, f, indent=2)
def print_metrics_summary(weave_results: Dict):
"""Print a summary of the evaluation metrics"""
print("\nEvaluation Summary")
print("=" * 80)
# Extract results from Weave's evaluation format
scorer_results = weave_results.get("EntityRecognitionScorer", {})
if not scorer_results:
print("No valid results available")
return
# Calculate overall metrics
total_samples = int(weave_results.get("model_latency", {}).get("count", 0))
passed = total_samples # Since we have results, all samples passed
failed = 0
print(f"Total Samples: {total_samples}")
print(f"Passed: {passed}")
print(f"Failed: {failed}")
print(f"Success Rate: {(passed/total_samples)*100:.2f}%")
# Print overall metrics
if "overall" in scorer_results:
overall = scorer_results["overall"]
print("\nOverall Metrics:")
print("-" * 80)
print(f"{'Metric':<20} {'Value':>10}")
print("-" * 80)
print(f"{'Precision':<20} {overall['precision']['mean']:>10.2f}")
print(f"{'Recall':<20} {overall['recall']['mean']:>10.2f}")
print(f"{'F1':<20} {overall['f1']['mean']:>10.2f}")
# Print entity-level metrics
print("\nEntity-Level Metrics:")
print("-" * 80)
print(f"{'Entity Type':<20} {'Precision':>10} {'Recall':>10} {'F1':>10}")
print("-" * 80)
for entity_type, metrics in scorer_results.items():
if entity_type == "overall":
continue
precision = metrics.get("precision", {}).get("mean", 0)
recall = metrics.get("recall", {}).get("mean", 0)
f1 = metrics.get("f1", {}).get("mean", 0)
print(f"{entity_type:<20} {precision:>10.2f} {recall:>10.2f} {f1:>10.2f}")
def preprocess_model_input(example: Dict) -> Dict:
"""Preprocess dataset example to match model input format."""
return {
"prompt": example["input_text"],
"model_type": example.get(
"model_type", "unknown"
), # Add model type for Presidio mapping
}
def main():
"""Main evaluation function"""
weave.init("guardrails-genie-pii-evaluation")
# Load test cases
test_cases = load_ai4privacy_dataset(num_samples=100)
# Add model type to test cases for Presidio mapping
models = {
"regex": RegexEntityRecognitionGuardrail(should_anonymize=True),
"presidio": PresidioEntityRecognitionGuardrail(should_anonymize=True),
"transformers": TransformersEntityRecognitionGuardrail(should_anonymize=True)
}
scorer = EntityRecognitionScorer()
# Evaluate each model
for model_name, guardrail in models.items():
print(f"\nEvaluating {model_name} model...")
# Add model type to test cases
model_test_cases = [{**case, "model_type": model_name} for case in test_cases]
evaluation = Evaluation(
dataset=model_test_cases,
scorers=[scorer],
preprocess_model_input=preprocess_model_input,
)
asyncio.run(evaluation.evaluate(guardrail))
if __name__ == "__main__":
main()
まず、PresidioとTransformerモデル間でエンティティタイプを正規化し、一貫性を確保するためのマッピング辞書を定義します。次に、エンティティレベルの比較を行い、評価メトリクスを計算するためのカスタムクラス EntityRecognitionScorer を実装します。このスコアラーは、各エンティティタイプにおける真陽性(True Positive)、偽陽性(False Positive)、偽陰性(False Negative)を考慮して評価を行います。
その後、load_ai4privacy_dataset 関数を使用してデータセットを準備し、評価用のテストケースを抽出・構造化します。各ガードレールをデータセットに適用し、検出されたエンティティを期待値と比較します。結果はWeaveの評価フレームワークを使用して記録および保存され、ガードレールのパフォーマンスを詳細に分析および視覚化できるようになります。
Weaveログは、各ガードレールのパフォーマンスを明確に視覚化し、全体的な検出精度だけでなく、個々のエンティティタイプごとの詳細なメトリクスも提供します。この詳細な記録により、異なる手法間の結果を簡単に比較でき、特定のPIIカテゴリに対して各ガードレールがどのように機能するかを明確にします。この評価により、シンプルさ、適応性、または複雑なデータの処理における堅牢性など、ユースケースの特定の要件に基づいて最適なガードレールを選択するのに役立ちます。
以下に、いくつかのパフォーマンスメトリクスの表を共有します:
モデルパフォーマンス
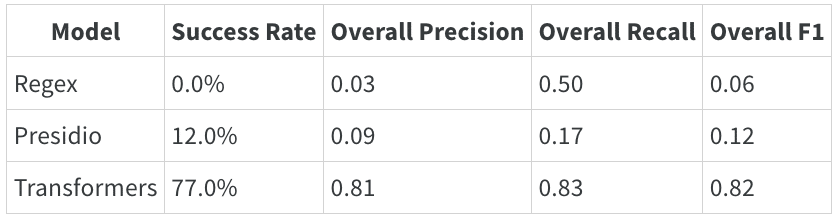
エンティティレベルの F1 スコア
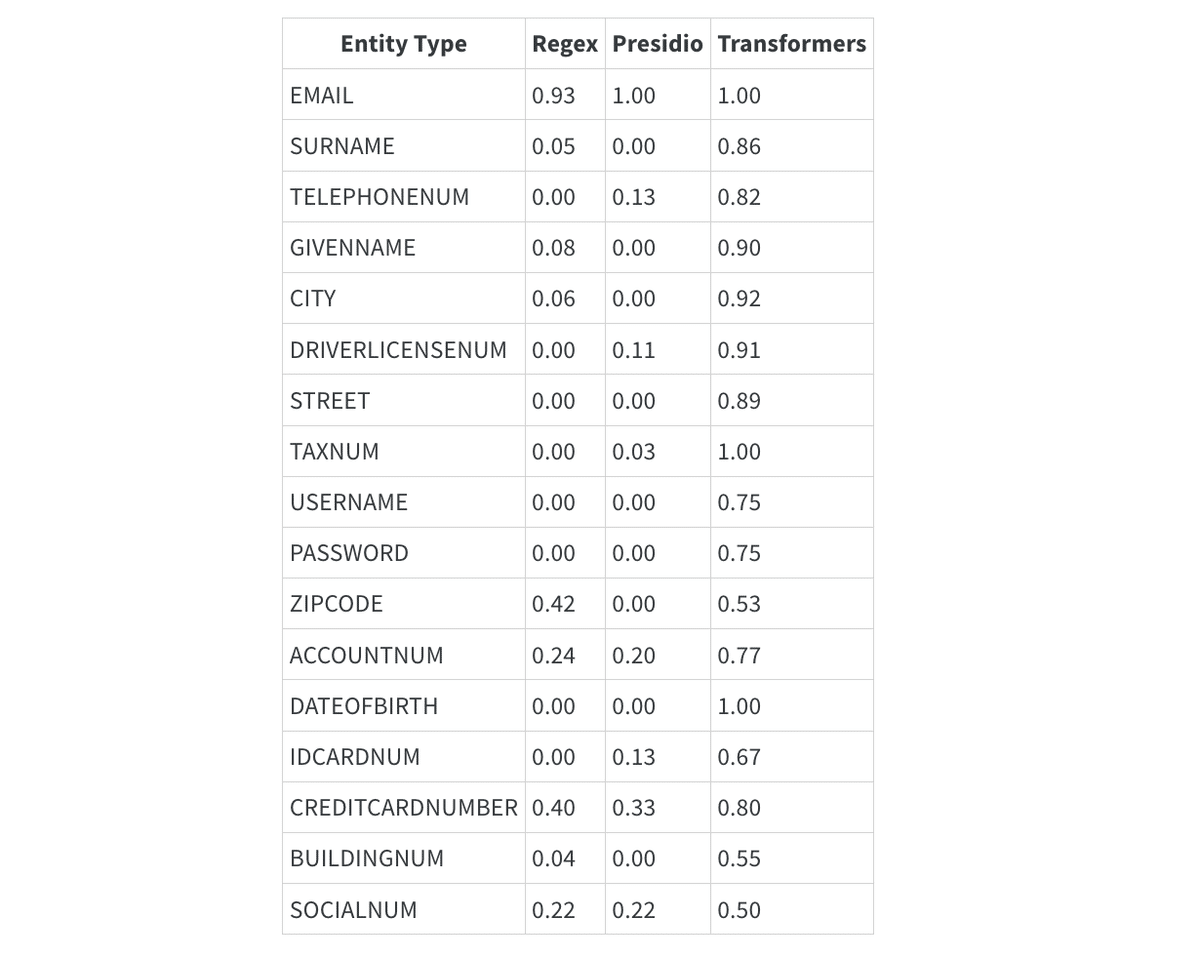
パフォーマンス分析
パフォーマンスの概要は、手法ごとにモデルの有効性に大きな差異があることを示しています。正規表現ベースのアプローチは成功率が0.0%、F1スコアが0.06と低く、精度や再現率も低い結果となり、関連するパターンを効果的に捉える能力が欠けていることがわかります。
Presidioは中程度のパフォーマンスを示し、成功率12.0%、F1スコア0.12を達成しています。これは、一部のエンティティを識別できるものの、精度と一貫性に課題があることを示しています。一方で、Transformerモデルは成功率77.0%、F1スコア0.82を記録し、その堅牢性とエンティティレベルでの精度と再現率のバランスを取る優れた能力を反映しています。これにより、Transformerのような高度な機械学習モデルが、正規表現やルールベースのアプローチといった従来の手法を大幅に上回るパフォーマンスを発揮することが明らかになりました。
複数の手法を組み合わせるアプローチは、効果的な戦略となり得ます。正規表現を用いて迅速にフィルタリングを行い、その後にフラグが付けられたコンテンツを詳細に分析することで精度を向上させる手法は、重要な用途において堅牢なパフォーマンスを確保します。また、特定のドメインに合わせて手法をカスタマイズすることも有効であり、業界特有のパターンを追加したり、ドメインに関連するデータ形式でモデルをトレーニングしたり、信頼性の閾値を調整したりすることが含まれます。
最後に、定期的なモニタリングはシステム改善の重要な原動力となります。これにより、偽陽性や偽陰性を追跡し、見逃されたケースに対応するためにパターンを更新し、モデルを再トレーニングして最新状態を保つことが可能になります。
結論
データ駆動型の世界において、PIIを検出し保護するための堅牢なガードレールは欠かせません。正規表現のような迅速で解釈性の高いアプローチを選ぶ場合でも、Transformersモデルのような高度な能力を活用する場合でも、ガードレール戦略は継続的なモニタリング、更新、そして規制基準への適合を優先すべきです。
Weaveのようなツールは、各ガードレールのパフォーマンスを可視化し、全体的なデータ保護戦略を洗練する助けとなります。各アプローチの強みと限界を理解し、それらを定期的に更新することで、高いプライバシーとセキュリティの基準を維持し、機密性の高い個人情報がしっかりと保護されるようにすることができます。