Time Series Split
今回は交差検証(Cross-Validation)のTime Series Splitを取り上げたいと思います。
以前、交差検証の最も基本的なアプローチであるK-fold 交差検証を取り上げましたが、Time Series SplitはK-foldのバリエーションになります。
この記事では、基本的なK-fold 交差検証について取り上げた後、時系列データに応用する際のテクニックについて取り上げましたが、時系列データやデータの順序が意味を持ってしまう場合、基本的にはK-fold 交差検証を利用することは難しいです。
K-fold 交差検証では、データを訓練データと検証用データに分割しますが、
各Splitでは検証用データの後に訓練データが来てしまい、その場合は将来のデータを使用して過去を予測することになってしまいます。
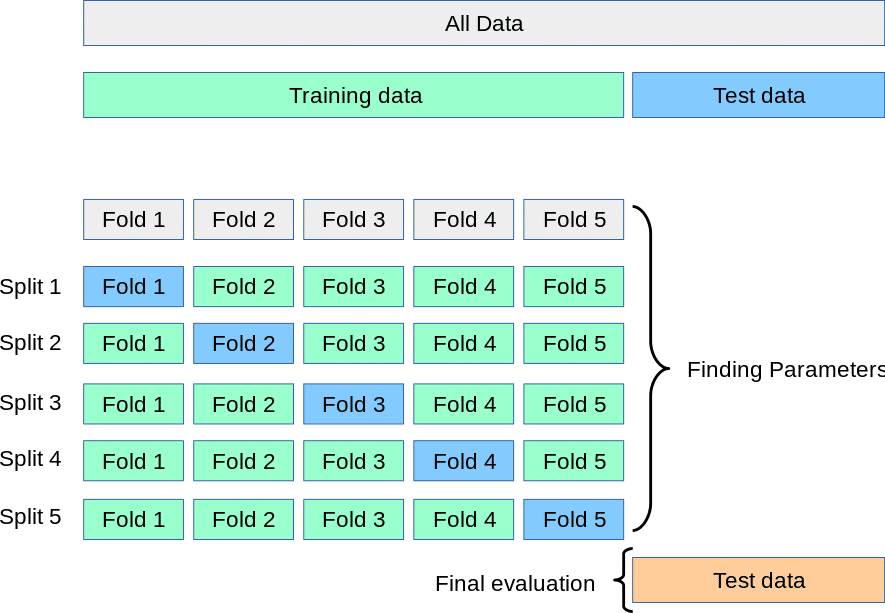
Time Series Split はこの問題を解決します。
Time Series Splitでは、下記の図にあるように訓練データのサイズが徐々に増加します。
訓練データは常に前回のイテレーションの訓練データを含むことになります。
Time Series Splitでは、最初の訓練データがテストセットに含まれることがありません。
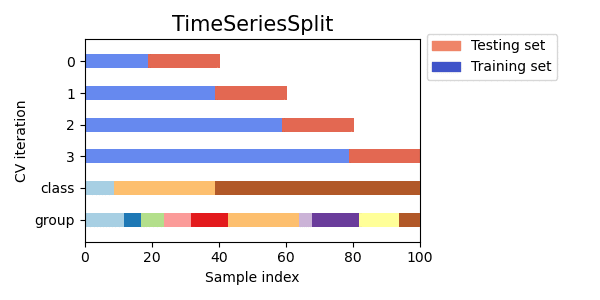
下記は、簡単なデモンストレーションです。
import numpy as np
from sklearn.model_selection import TimeSeriesSplit
X = np.array([[1], [2], [3], [4], [5], [6], [7], [8], [9], [10]])
tss = TimeSeriesSplit(n_splits=5)
for i, (train_index, test_index) in enumerate(tss.split(X)):
train_str = np.array2string(X[train_index], separator=',').replace('\n', '')
print(f"訓練用データ Index: {train_str}", end=' ')
test_str = np.array2string(X[test_index], separator=',').replace('\n', '')
print(f"検証用データ Index: {test_str}")
訓練用データ Index: [[1], [2], [3], [4], [5]] 検証用データ Index: [[6]]
訓練用データ Index: [[1], [2], [3], [4], [5], [6]] 検証用データ Index: [[7]]
訓練用データ Index: [[1], [2], [3], [4], [5], [6], [7]] 検証用データ Index: [[8]]
訓練用データ Index: [[1], [2], [3], [4], [5], [6], [7], [8]] 検証用データ Index: [[9]]
訓練用データ Index: [[1], [2], [3], [4], [5], [6], [7], [8], [9]] 検証用データ Index: [[10]]
上記の図のように、訓練データと検証用データが分割されているのが確認できます。