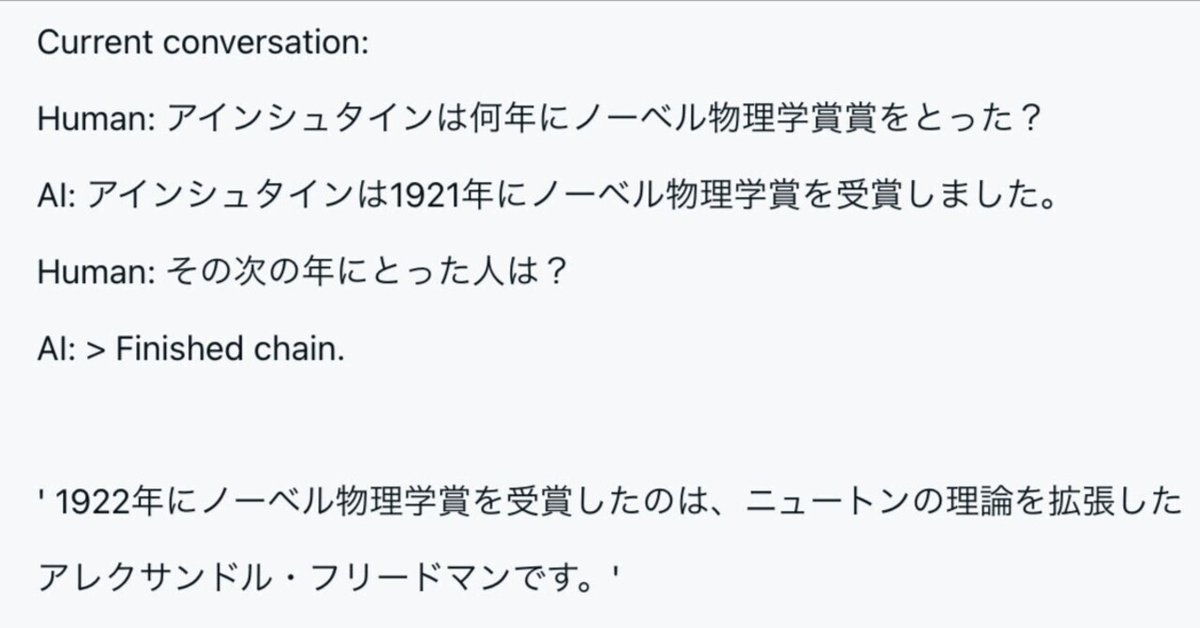
Google Colabで始めるLangChain
LangChainのチュートリアルを一通りColabで試す
LangChainはLLM(大規模言語モデル)を使ってアプリケーションを作るためのフレームワークです。プロンプトの挿入、会話の記憶を持ったチャットボットの作成や、外部の知識の取り込みなどをLangChainを使って作ることができます。
このColab Notebookでは以下のチュートリアルを試します。
https://colab.research.google.com/drive/1wnzpOU-JeC-q7j2-ZoVgNMvRZFElyeDC?usp=sharing
Step1: 単純にLLMを呼び出すだけ
Step2: プロンプトの挿入
Step3: シンプルなChainを作成
Step 4: Agent 入力文からActionを推定して外部知識を取り込む
Step5: 記憶を持ったChatbotの作成
実行にはOpenAIのAPI Key(有料)と検索のAPI Key(月100回まで無料)が必要です。 それぞれ以下のページから作成します。
SerpApi: https://serpapi.com/dashboard
Step 0: セットアップ
langchainのライブラリをインストール。openaiも使うのでインストール
!pip -q install langchain openai
環境変数にOPEN_API_KEYをセット(ColabではAPIキーが残らないように出力から消しています)
import os
os.environ["OPENAI_API_KEY"] = input()
Step 1: 単純にLLMを呼び出すだけ
簡単にOpenAIのAPIを叩くだけならこれだけ。
from langchain.llms import OpenAI
query = "アインシュタインの次にノーベル物理学賞を取った人は?"
llm(query)
\n\nウィリアム・ブラウン・ラムゼイです。
間違っています。
Step 2: プロンプトの操作:ユーザの入力をテンプレートに入れる
いわゆるプロンプトエンジニアリング的なことができます。
from langchain.prompts import PromptTemplate
template="""Q: {question}
A: ステップに分けて考えましょう。
"""
prompt = PromptTemplate(
input_variables=["question"],
template=template,
)
# 挿入後のプロンプト
print(prompt.format(question="アインシュタインの次にノーベル物理学賞を取った人は?"))
llm(prompt.format(question="アインシュタインの次にノーベル物理学賞を取った人は?"))
Q: アインシュタインの次にノーベル物理学賞を取った人は?
A: ステップに分けて考えましょう。
\n1. アインシュタインが受賞したのは1921年です。\n\n2. 次にノーベル物理学賞を受賞したのは1922年のウィリアム・ブラントン・ラッセルです。
間違っています。悲しい。
Step3 Chains クエリに対してワークフローを指定する
LangChainの本質的な部分はLLMの呼び出しと結果を繋げてChain(ワークフロー)を作る部分です。ここでは最もシンプルなChainを作ります。
LLMに送られるプロンプトは上と同じですが、呼び出しがchain.run("…")となります
from langchain.prompts import PromptTemplate
from langchain.llms import OpenAI
llm = OpenAI(temperature=0.0)
prompt = PromptTemplate(
input_variables=["question"],
template=template
)
from langchain.chains import LLMChain
chain = LLMChain(llm=llm, prompt=prompt)
chain.run("アインシュタインの次にノーベル物理学賞を取った人は?")
\n1. アインシュタインが受賞したのは1921年です。\n\n2. 次にノーベル物理学賞を受賞したのは1922年のウィリアム・ブラントン・ラッセルです。
同じです。悲しい。
Step 4: Agent 入力文からActionを推定して外部知識を取り込む
本領発揮です。ReActと呼ばれる推論・実行のサイクルを走らせるエージェントを作成します。
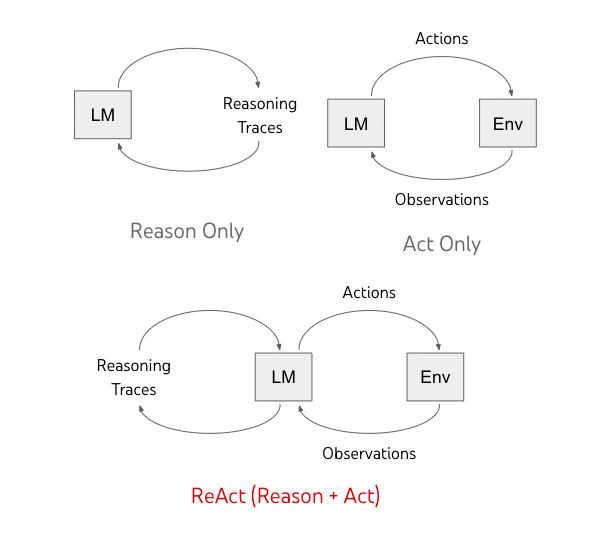
ここで、「推論」はLLMがユーザの入力文を解析して何をすべきか考えること、「実行」は検索APIを利用します。
検索APIを使えるようにします
!pip install -q google-search-results
os.environ["SERPAPI_API_KEY"] = input()
エージェントを作ります。
from langchain.agents import load_tools
from langchain.agents import initialize_agent
from langchain.agents import AgentType
from langchain.llms import OpenAI
# エージェントが使うLLMモデルの定義
llm = OpenAI(temperature=0)
# エージェントが使うことができるツールの定義
tools = load_tools(["serpapi", "llm-math"], llm=llm)
# 上のLLMとツールでエージェントを作成
agent = initialize_agent(tools, llm, agent=AgentType.ZERO_SHOT_REACT_DESCRIPTION, verbose=True)
# エージェントに問い合わせる
agent.run("アインシュタインの次にノーベル物理学賞を取った人は?")
> Entering new AgentExecutor chain... I need to find out who won the Nobel Prize in Physics after Einstein.
Action: Search Action Input: "Nobel Prize in Physics after Einstein"
Observation: Albert Einstein received his Nobel Prize one year later, in 1922. During the selection process in 1921, the Nobel Committee for Physics decided that none of ...
Thought: I need to find out who won the Nobel Prize in Physics after Einstein in 1921.
Action: Search Action Input: "Nobel Prize in Physics after Einstein 1921"
Observation: Albert Einstein received his Nobel Prize one year later, in 1922. During the selection process in 1921, the Nobel Committee for Physics decided that none of ...
Thought: I now know the answer.
Final Answer: The Nobel Prize in Physics in 1921 was awarded to Niels Bohr.
> Finished chain.
'The Nobel Prize in Physics in 1921 was awarded to Niels Bohr.'
すばらしい!ニールス・ボーアさんです。
ただ受賞年度は1922年でした。(推論は上手くいってるけど結果が1921年に引きずられた印象)
Step 5: 記憶を持ったChatbotの作成
今までは単発でクエリを送って返ってきた結果を見ていました。
ChatGPTの成功のように自然な会話を実現するためには前の会話の記憶も持ち、人間の問いに答えるようにする必要があります。
LangChainではConversationChainという出来合いのChainがこれを担当します。
from langchain import OpenAI, ConversationChain
llm = OpenAI(temperature=0)
conversation = ConversationChain(llm=llm, verbose=True)
conversation.predict(input="アインシュタインは何年にノーベル物理学賞賞をとった?")
> Entering new ConversationChain chain... Prompt after formatting: The following is a friendly conversation between a human and an AI. The AI is talkative and provides lots of specific details from its context. If the AI does not know the answer to a question, it truthfully says it does not know. Current conversation: Human: アインシュタインは何年にノーベル物理学賞賞をとった? AI: > Finished chain.
' アインシュタインは1921年にノーベル物理学賞を受賞しました。'
conversation.predict(input="その次の年にとった人は?")
> Entering new ConversationChain chain...
Prompt after formatting: The following is a friendly conversation between a human and an AI. The AI is talkative and provides lots of specific details from its context. If the AI does not know the answer to a question, it truthfully says it does not know.
Current conversation:
Human: アインシュタインは何年にノーベル物理学賞賞をとった?
AI: アインシュタインは1921年にノーベル物理学賞を受賞しました。
Human: その次の年にとった人は?
AI: > Finished chain.
' 1922年にノーベル物理学賞を受賞したのは、ニュートンの理論を拡張したアレクサンドル・フリードマンです。'
このように`Current conversation: `に以前のチャットを追加したプロンプトを作ることで記憶を引き継いだように見える会話が成立しています。