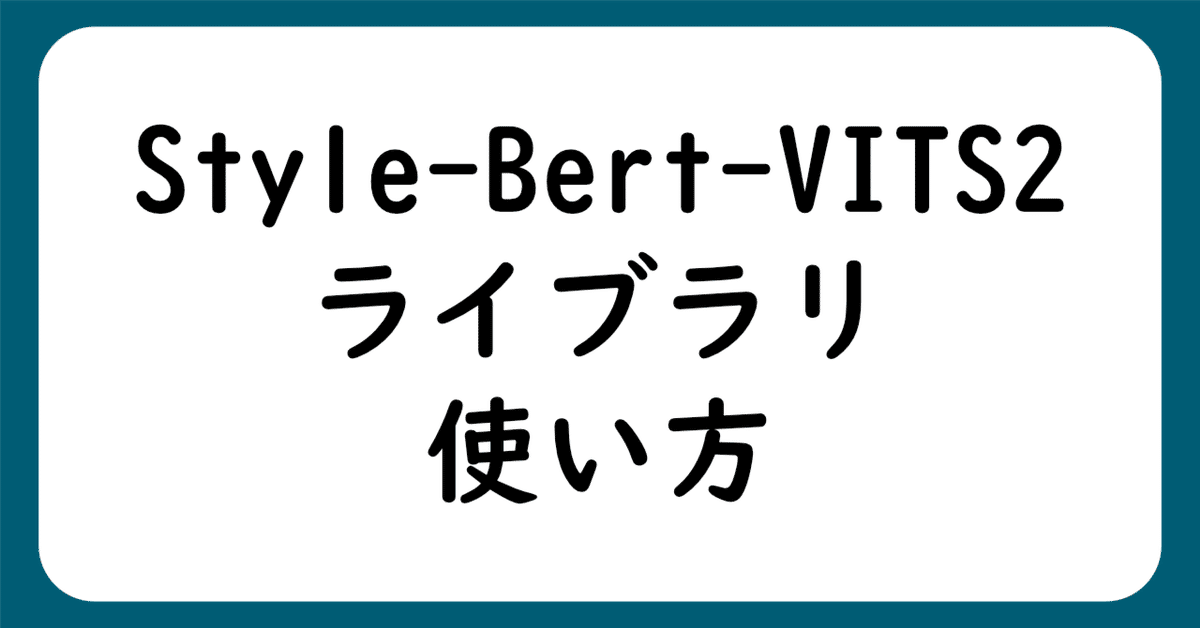
Style-Bert-VITS2ライブラリの使い方
Style-Bert-VITS2のライブラリを使ってテキストから音声に変換します。
Style-Bert-VITS2は、テキストを感情豊かな音声を生成するBert-VITS2 v2.1をもとに、感情や発話スタイルを強弱込みで自由に制御できるようにしたツールです。
実行環境
・Windows 10
・python 3.10.11
・style-bert-vits2 2.5.0
環境構築
仮想環境の作成
venvモジュールを使用して仮想環境を作成します。.venvは仮想環境のディレクトリ名です。
python -m venv .venv
作成した仮想環境を有効化
.venv\Scripts\activate
環境が有効かされると、コマンドプロンプトが以下の様に変更されます。(.venvの部分が仮想環境名を表しています)
(.venv) $
ライブラリのインストール
style-bert-vits2ライブラリは、音声合成のみ使うのでCPUで動作します。
高速に推論したい場合はGPUを使ってください。
学習させる場合はGitHubを参照してください。
CPUのみを使用する場合、以下のコマンド
pip3 install torch torchvision torchaudio
CUDAを使用する場合、以下のコマンド
pip install torch torchvision torchaudio --index-url https://download.pytorch.org/whl/cu118
他のバージョンのCUDAを使用する場合は、PyTorch公式サイトから適切なインストールコマンドを確認してください。
style-bert-vits2と音声ファイルの読み書きが簡単にできるsoundfile、wavファイルもしくはNumPyアレイを再生するsimpleaudioのインストール
pip install style-bert-vits2 soundfile simpleaudio
コード
参考の公式コード
ライブラリのインポート
from style_bert_vits2.nlp import bert_models
from style_bert_vits2.constants import Languages
from style_bert_vits2.tts_model import TTSModel
from huggingface_hub import hf_hub_download
from pathlib import Path
import simpleaudio
import soundfile as sf
BERTモデルをロード、自動でモデルがダウンロードされます。
bert_models.load_model(Languages.JP, "ku-nlp/deberta-v2-large-japanese-char-wwm")
bert_models.load_tokenizer(Languages.JP, "ku-nlp/deberta-v2-large-japanese-char-wwm")
Hugging Faceから試し用にデフォルトモデルをダウンロード
model_assetsディレクトリが作成され、モデルが保存されます。
# モデルの格納パス
model_file = "jvnv-F1-jp/jvnv-F1-jp_e160_s14000.safetensors"
config_file = "jvnv-F1-jp/config.json"
style_file = "jvnv-F1-jp/style_vectors.npy"
for file in [model_file, config_file, style_file]:
print(file)
# Hugging Faceからモデルのダウンロード
hf_hub_download("litagin/style_bert_vits2_jvnv", file, local_dir="model_assets")
モデルを持っている場合
環境にmodel_assetsディレクトリを作成し、model_assetsにモデルファイルを入れます。
workspace
├─ .venv
├─ model_assets
│ └─ モデル名
│ ├─ xxxxxxx.safetensors
│ ├─ config.json
│ └─ style_vectors.npy
└─ main.py
model_file、config_file、style_file の値を既存のモデル名に変更します。
Hugging Faceからモデルのダウンロードコードをコメントアウトします。
# モデルの格納パス
model_file = "モデル名/xxxxxxx.safetensors"
config_file = "モデル名/config.json"
style_file = "モデル名/style_vectors.npy"
# for file in [model_file, config_file, style_file]:
# print(file)
# hf_hub_download("litagin/style_bert_vits2_jvnv", file, local_dir="model_assets")
モデルのインスタンスの作成
assets_root = Path("model_assets")
model = TTSModel(
model_path=assets_root / model_file,
config_path=assets_root / config_file,
style_vec_path=assets_root / style_file,
device="cpu",
)
CUDA版をインストールしていたらdeviceにcudaを指定できます。
テキストから音声の作成
text = 'これはテスト音声です'
sr, audio = model.infer(text=text)
# 音声の再生
wave_obj = simpleaudio.WaveObject(audio, num_channels=1, sample_rate=sr)
play_obj = wave_obj.play()
play_obj.wait_done()
# 音声ファイルに保存
sf.write(f'test.wav', audio, samplerate=sr)
inferの引数
・text (str):読み上げるテキスト
・language (Languages, optional):言語。デフォルトは Languages.JP
・speaker_id (int, optional):話者ID。デフォルトは 0
・reference_audio_path (Optional[str], optional):音声スタイルの参照元の音声ファイルのパス。デフォルトは None
・sdp_ratio (float, optional):DPとSDPの混合比。0でDPのみ、1でSDPのみを使用(値を大きくするとテンポに緩急がつく)デフォルトは
・noise (float, optional):DPに与えられるノイズ。デフォルトは DEFAULT_NOISE(0.6)
・noise_w (float, optional):SDPに与えられるノイズ。デフォルトは DEFAULT_NOISEW(0.8)
・length (float, optional):生成音声の長さ(話速)のパラメータ。大きいほど生成音声が長くゆっくり、小さいほど短く早くなる。デフォ
・line_split (bool, optional):テキストを改行ごとに分割して生成するかどうか。デフォルトは DEFAULT_LINE_SPLIT(True)
・split_interval (float, optional):改行ごとに分割する場合の無音(秒)。デフォルトは DEFAULT_SPLIT_INTERVAL(0.5)
・assist_text (Optional[str], optional):感情表現の参照元の補助テキスト。デフォルトは None
・assist_text_weight (float, optional):感情表現の補助テキストを適用する強さ。デフォルトは DEFAULT_ASSIST_TEXT_WEIGHT(1.0)
・use_assist_text (bool, optional):音声合成時に感情表現の補助テキストを使用するかどうか。デフォルトは False
・style (str, optional):音声スタイル(Neutral, Happy など)。デフォルトは DEFAULT_STYLE("Neutral")
・style_weight (float, optional):音声スタイルを適用する強さ。デフォルトは DEFAULT_STYLE_WEIGHT(5.0)
・given_tone (Optional[list[int]], optional):アクセントのトーンのリスト。デフォルトは None
・pitch_scale (float, optional):ピッチの高さ(1.0から変更すると若干音質が低下する)。デフォルトは 1.0
・intonation_scale (float, optional):抑揚の平均からの変化幅(1.0から変更すると若干音質が低下する)。デフォルトは 1.0
全体のコード
from style_bert_vits2.nlp import bert_models
from style_bert_vits2.constants import Languages
from style_bert_vits2.tts_model import TTSModel
from huggingface_hub import hf_hub_download
from pathlib import Path
import simpleaudio
import soundfile as sf
# BERTモデルをロード
# 自動でモデルがダウンロードされます
bert_models.load_model(Languages.JP, "ku-nlp/deberta-v2-large-japanese-char-wwm")
bert_models.load_tokenizer(Languages.JP, "ku-nlp/deberta-v2-large-japanese-char-wwm")
# Hugging Faceから試し用にデフォルトモデルをダウンロード
# model_assetsディレクトリが作成され、そこにモデルが保存されます
model_file = "jvnv-F1-jp/jvnv-F1-jp_e160_s14000.safetensors"
config_file = "jvnv-F1-jp/config.json"
style_file = "jvnv-F1-jp/style_vectors.npy"
# モデルがある場合コメントアウト
for file in [model_file, config_file, style_file]:
print(file)
hf_hub_download("litagin/style_bert_vits2_jvnv", file, local_dir="model_assets")
assets_root = Path("model_assets")
model = TTSModel(
model_path=assets_root / model_file,
config_path=assets_root / config_file,
style_vec_path=assets_root / style_file,
device="cpu",
)
text = 'これはテスト音声です'
sr, audio = model.infer(text=text)
# 音声の再生
wave_obj = simpleaudio.WaveObject(audio, num_channels=1, sample_rate=sr)
play_obj = wave_obj.play()
play_obj.wait_done()
# 音声ファイルに保存
sf.write(f'test.wav', audio, samplerate=sr)