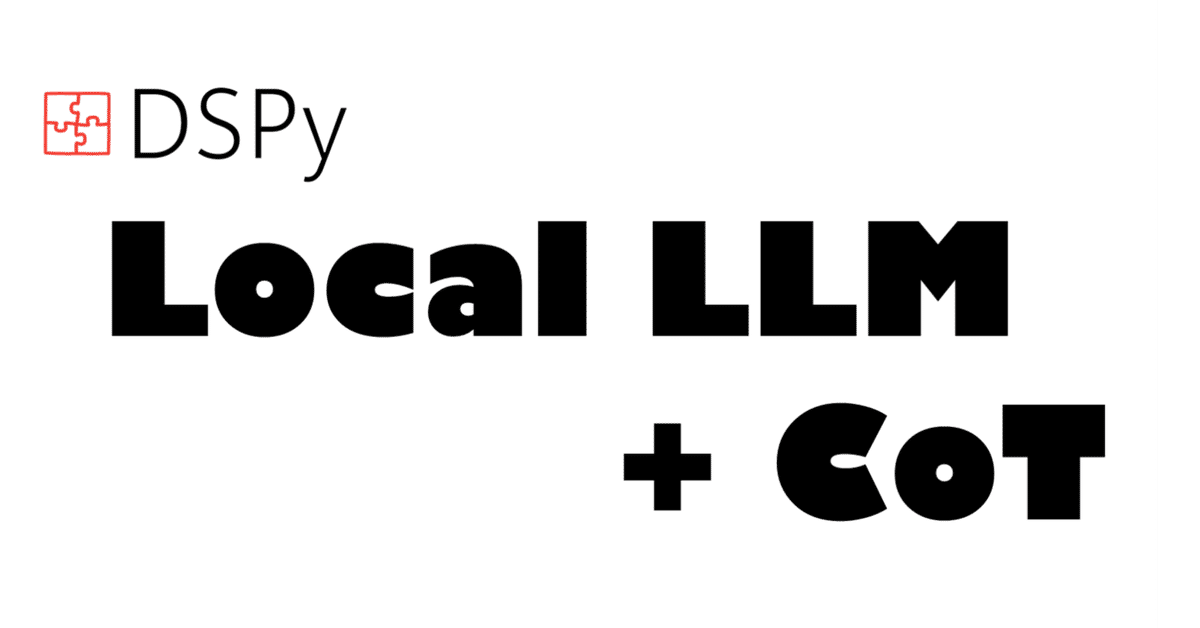
DSPy+CoT, RAG series 4/n
RAGシリーズ4回目。
今回は自動でprompt最適化してくれるDSPyを用いたRAGです。
Prompt Engineeringが面倒だと感じる私の助けになってくれるかもしれません。今回は推論タスクで効果を発揮するCoT(Chain-of-Thought)を用います。
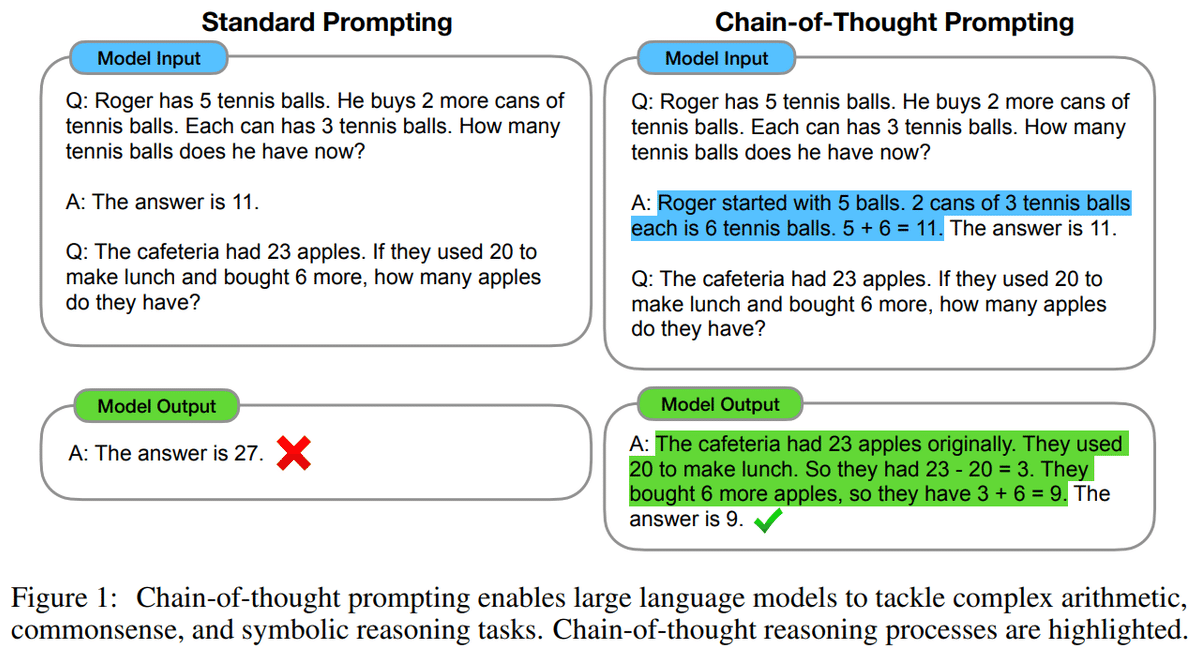
0. 環境
OS:Windows
CPU:Intel(R) Core i9-13900KF
RAM:128GB
GPU:RTX 4090
1. HotpotQA Dataset
今回はWikipediaの質問応答DatasetのHotpotQA Datasetを使います。
from dspy.datasets import HotPotQA
dataset = HotPotQA(train_seed=1, test_size=0, train_size=1000)
dataset = [x.with_inputs('question') for x in dataset.train]
2. Vector DB
読み込んだDatasetからRAGに使用するVector DBを作成します。
from dspy.retrieve.qdrant_rm import QdrantRM
from qdrant_client import QdrantClient
qdrant_client = QdrantClient(":memory:") # In-memory load
docs = [x.question + " -> " + x.answer for x in dataset]
ids = list(range(0,len(docs)))
qdrant_client.add(
collection_name="hotpotqa",
documents=docs,
ids=ids
)
qdrant_retriever_model = QdrantRM("hotpotqa", qdrant_client, k=3)
3. LLM modelの設定
modelはHODACHIさんのHODACHI/EZO-Common-T2-2B-gemma-2-itを使用しました。
import dspy
model_name = "HODACHI/EZO-Common-T2-2B-gemma-2-it"
llm = dspy.HFModel(model=model_name, hf_device_map='auto')
4. DSPyのCoTを設定
LLMが回答に用いるtextの抽出、そこから推論の生成、生成された推論に基づいた回答の生成を行います。
dspy.settings.configure(rm=qdrant_retriever_model, lm=llm)
class GenerateAnswer(dspy.Signature):
context = dspy.InputField(desc="may contain relevant facts or answer keywords")
question = dspy.InputField()
answer = dspy.OutputField(desc="an answer between 1 to 10 words")
class RAG(dspy.Module):
def __init__(self, num_passages=3):
super().__init__()
self.retrieve = dspy.Retrieve(k=num_passages)
self.generate_answer = dspy.ChainOfThought(GenerateAnswer, max_tokens=4000)
def forward(self, question):
context = self.retrieve(question).passages
prediction = self.generate_answer(context=context, question=question)
return dspy.Prediction(context=context, answer=prediction.answer)
uncompiled_rag = RAG()
5. 質疑
質問
my_question = "Was George Alan O'Dowd the most popular in the late 2000s with his rock band?"
response = uncompiled_rag(my_question)
start = response.answer.find("Answer: ")
end = response.answer[start:].find("\n")
print(response.answer[start:start+end])
回答
Answer: No, George O'Dowd was not popular in the late 2000s.
contextの出力
start = response.answer.find("Context:")
end = response.answer[start:].find("\n\n")
print(response.answer[start:start+end])
Context:
[1] «Who has been on a British television music competition show and was was most popular in the 80's with the pop band 'Culture Club'? -> George Alan O'Dowd»
[2] «Who was dubbed the father of the type of rock music that emerged from post-punk in the late 1970s? -> Brian Healy»
[3] «Alan Forbes has done posters for an American rock band that formed in 1996 in what city in California? -> Palm Desert»
Reasoningの出力
start = response.answer.find("Reasoning: ")
end = response.answer[start:].find("\n")
print(response.answer[start:start+end])
Reasoning: Let's think step by step in order to determine if George O'Dowd was still popular in the late 2000s. We know from context that he was popular in the 80s with Culture Club. The late 2000s fall outside of his peak popularity period.
一応、一連の理由に基づいて質問に回答してはいるようです。
少々タスクが簡単すぎたかもしれません。