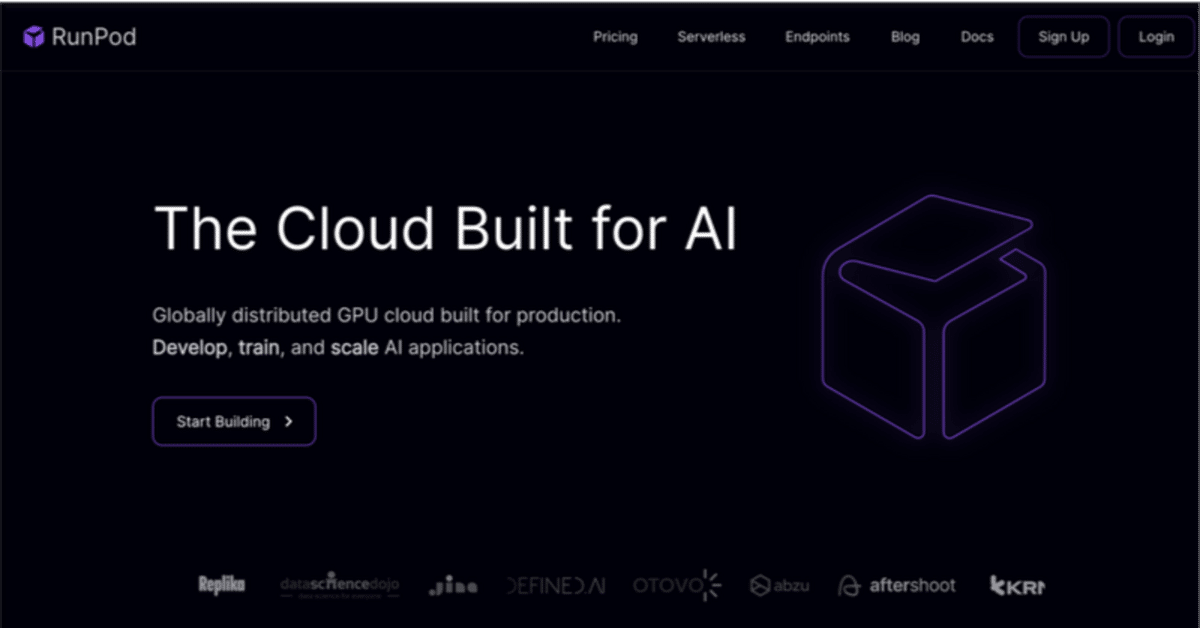
RunPodで独自モデルによるストリーム出力
概要
前回は独自モデルを利用するサンプルについて解説しました。LLMモデルを使う前に、ストリーム処理を行う方法を確認してみました。LLMは通常は長い実行時間が掛かるため、ストリーム処理を行うことで結果を待つストレスを軽減できます。
モデルの作成
RunPodでは、yieldで結果を返すことでストリーム処理が行なえます。以下のようにgenerate_with_streaming関数の中で、1秒おきに文字列を返すことにしました。ここでは簡単のため、"This is a pen."という文字列を1文字ずつ返すことにします。モデルのデプロイについては前回と同様に行っていきます。
import time
import runpod
def generate_with_streaming(text):
for t in text:
yield t
time.sleep(1)
def handler(event):
text = "This is a pen."
output = generate_with_streaming(text)
for res in output:
yield res
runpod.serverless.start({"handler": handler})
ストリーム処理の実行
デプロイしたAPIにアクセスしてストリーム処理をチェックしてみましょう。コードが少し長くなるので、分割して解説していきます。まず最初にAPIを呼び出してタスクを作成します。前回はrunsyncでしたが、今回は途中で非同期に実行するのでrunを利用します。タスクを作成したらtask_idを取得しておきます。
import requests
from time import sleep
endpoint_id = "<Endpoint ID>"
url = f"https://api.runpod.ai/v2/{endpoint_id}/run"
api_key = "<API Key>"
request = {
'input': {'query': 'Who am I?'}
}
headers = {
"Authorization": f"Bearer {api_key}"
}
response = requests.post(url, json=request, headers = headers)
data = response.json()
task_id = data['id']
メインの部分は以下になります。streamエンドポイントからデータを取得します。printだと改行されてしまうので、ここでは得られたデータをflushして出力していきます。途中で実行を停止するためには、Ctrl+Cなどで止めると、KeyboardInterruptの例外に飛びキャンセル処理を行います。
url = f"https://api.runpod.ai/v2/{endpoint_id}/stream/{task_id}"
headers = {
"Authorization": f"Bearer {api_key}"
}
try:
while True:
response = requests.get(url, headers=headers)
if response.status_code == 200:
data = response.json()
if len(data['stream']) > 0:
new_output = ''.join([s['output'] for s in data['stream']])
sys.stdout.write(new_output)
sys.stdout.flush()
if data['status'] == 'COMPLETED':
break
elif response.status_code >= 400:
print(response)
# Sleep for 0.1 seconds between each request
sleep(0.1)
except KeyboardInterrupt:
# キャンセル処理
task_cancel(task_id)
キャンセル処理は以下のようにcancelエンドポイントにPOSTを送ります。
def task_cancel(task_id):
# キャンセル処理
print("Cancel")
url = f"https://api.runpod.ai/v2/{endpoint_id}/cancel/{task_id}"
headers = {
"Authorization": f"Bearer {api_key}"
}
ret = requests.post(url, data=None, headers=headers)
print(ret.json())
実行結果ですが、本当はストリームで返ってきているのですが、noteなので表現できていませんね。以下のように文字列を取得できます。
This is a pen.
まとめ
LLMを動かす前にストリーム処理を実行する実験を行いました。次回こそはLLMを動かしていこうと思います。