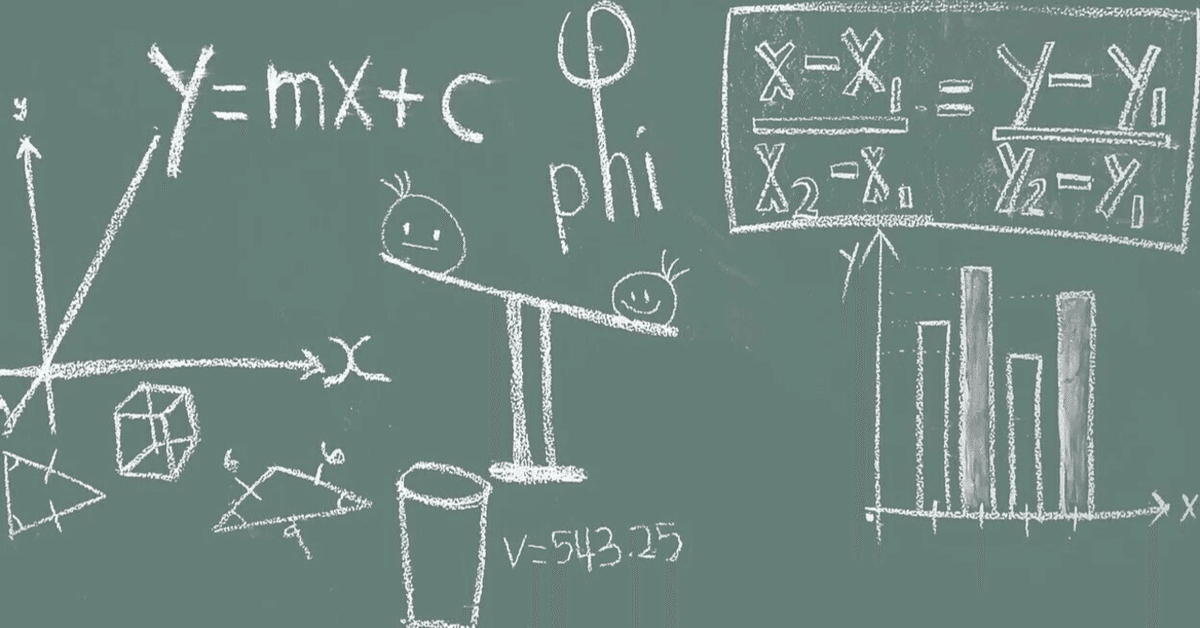
Photo by
yumihipiri_17
E検定対策(6) - K近傍法を用いた分類
今回はK近傍法( K-Nearest Neighbors Classification )の勉強をしました。
K近傍法とは
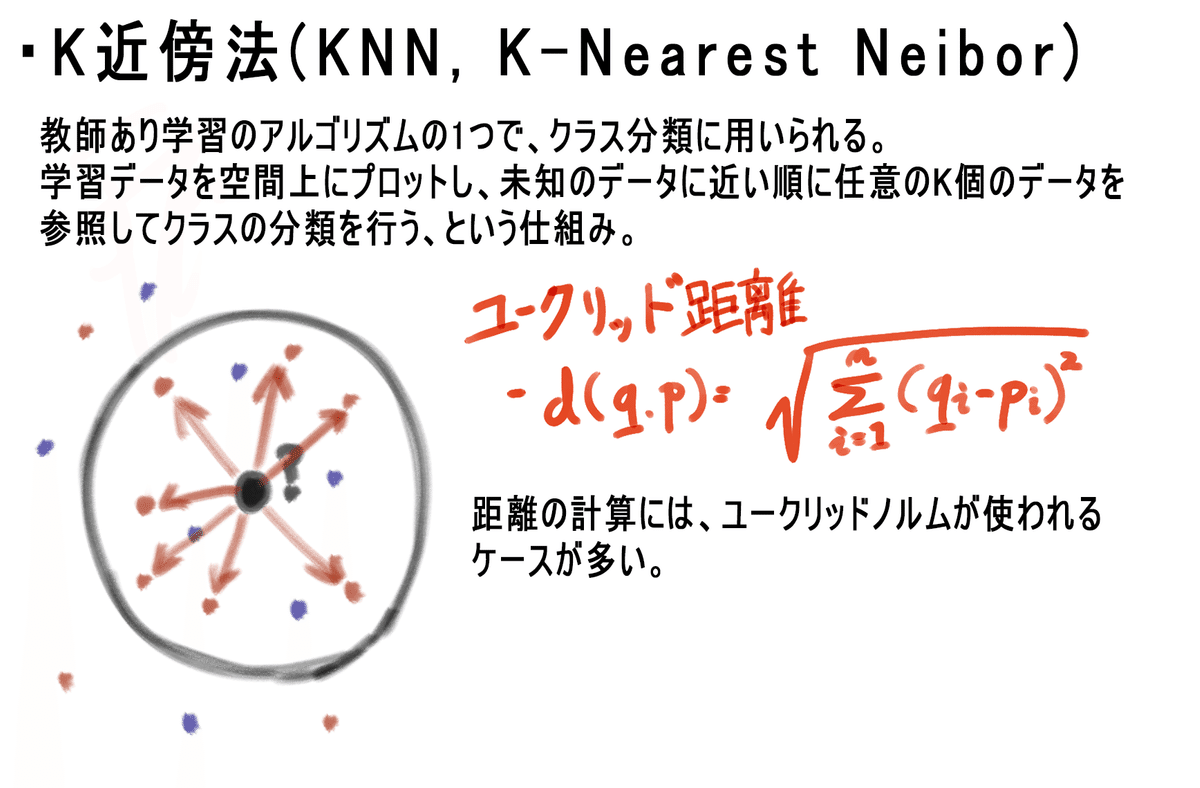
K近傍法を使ったIrisデータセットの分類
#必要なライブラリーをインポート
import matplotlib.pyplot as plt
import seaborn as sns
from matplotlib.colors import ListedColormap
from sklearn import neighbors, datasets
from sklearn.inspection import DecisionBoundaryDisplay
#Irisデータセットをロード
iris = datasets.load_iris()
#データの内、説明変数の最初の2つを利用する
X = iris.data[:, :2]
y = iris.target
# カラーマップを作成
cmap_light = ListedColormap(["orange", "cyan", "cornflowerblue"])
cmap_bold = ["darkorange", "c", "darkblue"]
n_neighbors = 15
for weights in ["distance"]:
#K近傍法を使ってデータをプロットする
clf = neighbors.KNeighborsClassifier(n_neighbors, weights=weights)
clf.fit(X, y)
_, ax = plt.subplots()
DecisionBoundaryDisplay.from_estimator(
clf,
X,
cmap=cmap_light,
ax=ax,
response_method="predict",
plot_method="pcolormesh",
xlabel=iris.feature_names[0],
ylabel=iris.feature_names[1],
shading="auto",
)
#トレーニングに使用したデータをプロット
sns.scatterplot(
x=X[:, 0],
y=X[:, 1],
hue=iris.target_names[y],
palette=cmap_bold,
alpha=1.0,
edgecolor="black",
)
plt.title(
"3-Class classification (k = %i, weights = '%s')" % (n_neighbors, weights)
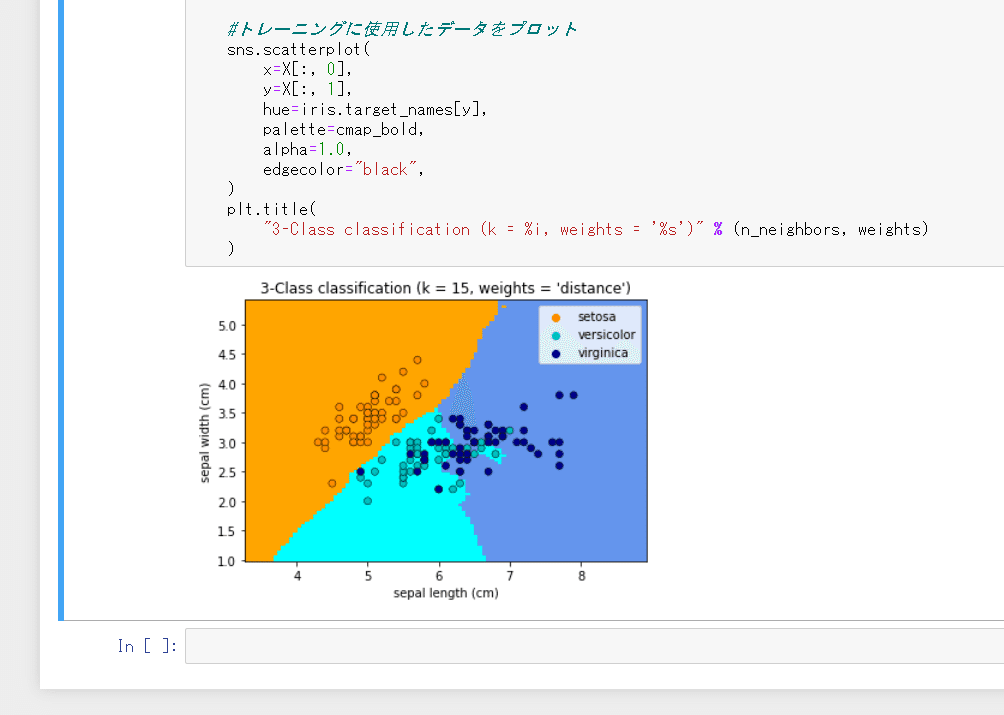
もともとデータの説明変数が2つでは足りないこともあってか、今回は精度は低めです。次回はTensorflowを使った教師なし学習・主成分分析( PCA )について書く予定です。楽しみですね!