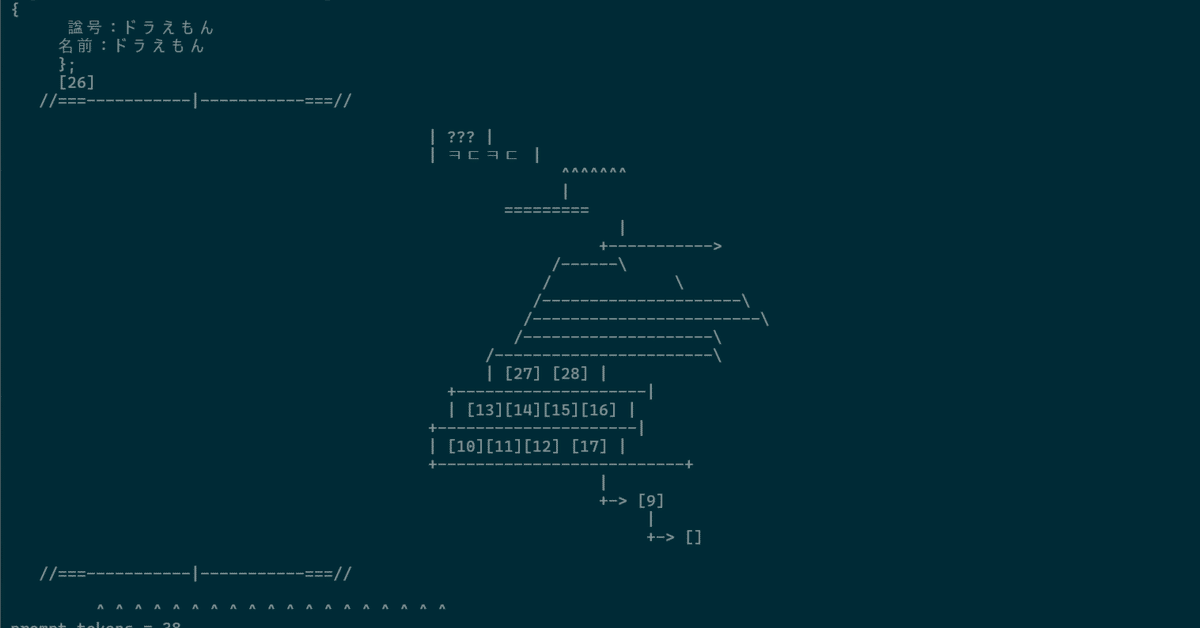
WSL2でFugaku-LLM-13B-instructを試してみる
理化学研究所のスーパーコンピュータ「富岳」を用いて学習した、日本語能力に優れた大規模言語モデルFugaku-LLMを試してみます。
※別記事でggufを試してしまったので、明確にするためにタイトルを「Fugaku-LLM」から「Fugaku-LLM-13B-instruct」に修正しています。
現在3つのモデルが公開されています。
今回は、2つ目のFugaku-LLM-13B-instructを試してみます。
使用するPCはドスパラさんの「GALLERIA UL9C-R49」。スペックは
・CPU: Intel® Core™ i9-13900HX Processor
・Mem: 64 GB
・GPU: NVIDIA® GeForce RTX™ 4090 Laptop GPU(16GB)・GPU: NVIDIA® GeForce RTX™ 4090 (24GB)
・OS: Ubuntu22.04 on WSL2(Windows 11)
です。
1. 準備
venv構築
python3 -m venv fugaku
cd $_
source bin/activate
パッケージのインストール
pip install torch transformers accelerate
2. 流し込むコード
いつものコードをquery.pyとして保存します。
import sys
import argparse
import torch
from transformers import AutoModelForCausalLM, AutoTokenizer, TextStreamer
from typing import List, Dict
import time
# argv
parser = argparse.ArgumentParser()
parser.add_argument("--model-path", type=str, default=None)
parser.add_argument("--tokenizer-path", type=str, default=None)
parser.add_argument("--no-chat", action='store_true')
parser.add_argument("--no-use-system-prompt", action='store_true')
parser.add_argument("--max-tokens", type=int, default=256)
args = parser.parse_args(sys.argv[1:])
model_id = args.model_path
if model_id == None:
exit
is_chat = not args.no_chat
use_system_prompt = not args.no_use_system_prompt
max_new_tokens = args.max_tokens
tokenizer_id = model_id
if args.tokenizer_path:
tokenizer_id = args.tokenizer_path
# トークナイザーとモデルの準備
tokenizer = AutoTokenizer.from_pretrained(
tokenizer_id,
trust_remote_code=True
)
model = AutoModelForCausalLM.from_pretrained(
model_id,
torch_dtype="auto",
#torch_dtype=torch.bfloat16,
device_map="auto",
#device_map="cuda",
low_cpu_mem_usage=True,
trust_remote_code=True
)
#if torch.cuda.is_available():
# model = model.to("cuda")
streamer = TextStreamer(
tokenizer,
skip_prompt=True,
skip_special_tokens=True
)
DEFAULT_SYSTEM_PROMPT = "あなたは誠実で優秀な日本人のアシスタントです。"
def q(
user_query: str,
history: List[Dict[str, str]]=None
) -> List[Dict[str, str]]:
# generation params
generation_params = {
"do_sample": True,
"temperature": 0.8,
"top_p": 0.95,
"top_k": 40,
"max_new_tokens": max_new_tokens,
"repetition_penalty": 1.1,
}
#
start = time.process_time()
# messages
messages = ""
if is_chat:
messages = []
if use_system_prompt:
messages = [
{"role": "system", "content": DEFAULT_SYSTEM_PROMPT},
]
user_messages = [
{"role": "user", "content": user_query}
]
else:
user_messages = user_query
if history:
user_messages = history + user_messages
messages += user_messages
# generation prompts
if is_chat:
prompt = tokenizer.apply_chat_template(
conversation=messages,
add_generation_prompt=True,
tokenize=False
)
else:
prompt = messages
input_ids = tokenizer.encode(
prompt,
add_special_tokens=True,
return_tensors="pt"
)
print("--- prompt")
print(prompt)
print("--- output")
# 推論
output_ids = model.generate(
input_ids.to(model.device),
streamer=streamer,
**generation_params
)
output = tokenizer.decode(
output_ids[0][input_ids.size(1) :],
skip_special_tokens=True
)
if is_chat:
user_messages.append(
{"role": "assistant", "content": output}
)
else:
user_messages += output
end = time.process_time()
##
input_tokens = len(input_ids[0])
output_tokens = len(output_ids[0][input_ids.size(1) :])
total_time = end - start
tps = output_tokens / total_time
print(f"prompt tokens = {input_tokens:.7g}")
print(f"output tokens = {output_tokens:.7g} ({tps:f} [tps])")
print(f" total time = {total_time:f} [s]")
return user_messages
print('history = ""')
print('history = q("ドラえもんとはなにか")')
print('history = q("続きを教えてください", history)')
3. 試してみる
実行コマンド
RTX 4090(24GB)1枚ではロードできないので、RTX 4090 + RTX 4090 Laptop GPUの2枚を使用してロードします。
CUDA_VISIBLE_DEVICES=0,1 python -i /path/to/query.py --model-path Fugaku-LLM/Fugaku-LLM-13B-instruct
チャットテンプレートの設定
トークナイザのchat_templateが適切に設定されていないようですので、README.mdで示されている形式でtokenizer.chat_templateを上書き設定します。
見やすい内容はこちらで、
{% if messages[0]['role'] == 'system' %}
{% set loop_messages = messages[1:] %}
{% set system_message = messages[0]['content'].strip() + '\n\n' %}
{% else %}
{% set loop_messages = messages %}
{% set system_message = '' %}
{% endif %}
{{ bos_token + system_message }}
{% for message in loop_messages %}
{% if (message['role'] == 'user') != (loop.index0 % 2 == 0) %}
{{ raise_exception('Conversation roles must alternate user/assistant/user/assistant/...') }}
{% endif %}
{% if message['role'] == 'user' %}
{{ '### 指示:\n' + message['content'].strip() + '\n\n' }}
{% elif message['role'] == 'assistant' %}
{{ '### 応答:\n' + message['content'].strip() + eos_token + '\n\n' }}
{% endif %}
{% if loop.last and message['role'] == 'user' and add_generation_prompt %}
{{ '### 応答:\n' }}
{% endif %}
{% endfor %}
実際には、以下をコピペして変数の内容を上書きします。
tokenizer.chat_template="{% if messages[0]['role'] == 'system' %}{% set loop_messages = messages[1:] %}{% set system_message = messages[0]['content'].strip() + '\n\n' %}{% else %}{% set loop_messages = messages %}{% set system_message = '' %}{% endif %}{{ bos_token + system_message }}{% for message in loop_messages %}{% if (message['role'] == 'user') != (loop.index0 % 2 == 0) %}{{ raise_exception('Conversation roles must alternate user/assistant/user/assistant/...') }}{% endif %}{% if message['role'] == 'user' %}{{ '### 指示:\n' + message['content'].strip() + '\n\n' }}{% elif message['role'] == 'assistant' %}{{ '### 応答:\n' + message['content'].strip() + eos_token + '\n\n' }}{% endif %}{% if loop.last and message['role'] == 'user' and add_generation_prompt %}{{ '### 応答:\n' }}{% endif %}{% endfor %}"
聞いてみる
いつものとおり聞いてましょう。
>>> history = q("ドラえもんとはなにか")
--- prompt
<s|LLM-jp>あなたは誠実で優秀な日本人のアシスタントです。
### 指示:
ドラえもんとはなにか
### 応答:
--- output
A decoder-only architecture is being used, but right-padding was detected! For correct generation results, please set `padding_side='left'` when initializing the tokenizer.
マクロは、シンボルがヌルポインタへの参照かどうかをチェックするために使用されます。コードギアス』では、このマクロが「Cの神」と呼ばれるユフィ・サトキのキャラクターを指すのに使われている。リオス休暇中にギアスを得たC.C.は、自分の人格と力を乗っ取り、
ルルーシュに「コードギアス」をかけてコードを書かせ、世界を崩壊させようと計画する。 Mesa VGAメモリアクセスのバグ(MMIO 0xc0000005)を悪用して、C.C.のコードをドラえもんとして挿入し、彼が望むことを何でもできるようにする。最終的にルルーシュは、C.C.を破壊することで、彼女の計画を阻止する。
prompt tokens = 36
output tokens = 182 (15.448234 [tps])
total time = 11.781282 [s]
>>>
マクロは、シンボルがヌルポインタへの参照かどうかをチェックするために使用されます。コードギアス』では、このマクロが「Cの神」と呼ばれるユフィ・サトキのキャラクターを指すのに使われている。リオス休暇中にギアスを得たC.C.は、自分の人格と力を乗っ取り、
ルルーシュに「コードギアス」をかけてコードを書かせ、世界を崩壊させようと計画する。 Mesa VGAメモリアクセスのバグ(MMIO 0xc0000005)を悪用して、C.C.のコードをドラえもんとして挿入し、彼が望むことを何でもできるようにする。最終的にルルーシュは、C.C.を破壊することで、彼女の計画を阻止する。
かなり斜め上な気がします。
なお、VRAMの使用量は27GBぐらいです。
|=========================================+========================+======================|
| 0 NVIDIA GeForce RTX 4090 ... On | 00000000:02:00.0 On | N/A |
| N/A 44C P8 7W / 150W | 14502MiB / 16376MiB | 8% Default |
| | | N/A |
+-----------------------------------------+------------------------+----------------------+
| 1 NVIDIA GeForce RTX 4090 On | 00000000:0C:00.0 Off | Off |
| 30% 31C P8 9W / 450W | 13286MiB / 24564MiB | 0% Default |
| | | N/A |
+-----------------------------------------+------------------------+----------------------+
4. まとめ
以下の記事ではないですが、データセットはきちんとクリーニングしていないと品質が…ですね。
9. おまけ
モデルのサイズ
Fugaku-LLM-13Bのモデルのファイルサイズが50GBを超えているのは、torch_dtypeがfloat32 (config.json)だからですね。なので、サイズが2倍。
Fugaku-LLM-13B-instructは bfloat16なので、26GBほどです。
チャットテンプレートの設定
ちなみに、プロンプトの設定をREADME.mdにあるような形式できてなかったとき、AAが出力されることが多かったです。プロンプトって大事ですよね。はい。
>>> history = q("ドラえもんとは")
--- prompt
<s|LLM-jp>あなたは誠実で優秀な日本人のアシスタントです。
### 指示:
ドラえもんとは
### 応答:
--- output
A decoder-only architecture is being used, but right-padding was detected! For correct generation results, please set `padding_side='left'` when initializing the tokenizer.
ドラえもん
{
/\_/\\
( o.o )
}
// /~~~\
|| [] ||
\_^_/
~==~~^>^_^>=~
( ^.^ )
""
```
この回答が、より良いものになることを願っている。また何か質問があったり、手伝えることがあれば教えてください!
prompt tokens = 38
output tokens = 105 (15.029886 [tps])
total time = 6.986081 [s]