言語データセットを用い、プロンプトチューニングで分子構造から融点を予測する際のメモ
※23/12/28 コード実装にミスがあったので修正しました。
こちらの続きです。
はじめに
既存のケモ・マテリアルズインフォマティクスは基本的に言語モデルを使いません。
そのような特化型モデルは、予測過程が人間に分かりづらかったり、科学的にはありえないロジックで推論してしまうデメリットがありえます。
なので、大規模言語モデルに物事を考えさせる研究を進めています。
前回は、分子の構造と融点の関係を記録した「構造ー物性相関」データセットをもとに、その理由をGPTに考察させる作業を行いました(こちら)。
500件ほどの言語データが生成されたので、その有効性を検証していきます。
コードのスナップショットはこちら
訓練データの生成
生成されたデータはこちらのフォルダにJSONとして格納されています。
[
{
"mpC": 99.0,
"name": "(2E)-1,3-Bis-(4-methoxyphenyl)-2-propen-1-one",
"smiles": "COC1=CC=C(C=C1)C(\\C=C\\C2=CC=C(C=C2)OC)=O",
"csid": 4526784,
"link": "http://www.beilstein-journals.org/bjoc/single/articleFullText.htm?publicId=1860-5397-7-198#supporting-info",
"source": "Beilstein Journal of Organic Chemistry",
"Reason": "",
"Prediction": ""
},
{
"mpC": 99.0,
"name": "(2E)-1,3-Bis-(4-methoxyphenyl)-2-propen-1-one",
"smiles": "COC1=CC=C(C=C1)C(\\C=C\\C2=CC=C(C=C2)OC)=O",
"csid": 4526784,
"link": "http://www.beilstein-journals.org/bjoc/single/articleFullText.htm?publicId=1860-5397-7-198#supporting-info",
"source": "Beilstein Journal of Organic Chemistry",
"Reason": "The target compound (2E)-1,3-Bis-(4-methoxyphenyl)-2-propen-1-one is a chalcone derivative possessing two benzene rings and additional functional groups including alkenes and ethers. Basic benzene has a melting point of approximately 5.5 degrees Celsius. The presence of the two 4-methoxyphenyl groups contributes positively to the melting point since methoxy groups and the phenyl ring are large, providing additional intermolecular interactions, like van der Waals forces. Each 4-methoxyphenyl might add around +70 to the melting point due their electron-donating nature and steric hindrance. The propenone bridge within the molecule introduces a polar carbon-oxygen double bond (C=O) and a carbon-carbon double bond (C=C). The polar C=O bond can introduce dipole-dipole interactions, likely increasing the melting point further by around +20. The C=C bond may have a minimal effect because it does not significantly alter the polarity or molecular symmetry, thus its contribution could be estimated at around +10. Summing the effects leads to a predicted melting point.",
"Prediction": "155.5",
"Prediction(integer)": 155.0
},
{
"mpC": 99.0,
"name": "(2E)-1,3-Bis-(4-methoxyphenyl)-2-propen-1-one",
"smiles": "COC1=CC=C(C=C1)C(\\C=C\\C2=CC=C(C=C2)OC)=O",
"csid": 4526784,
"link": "http://www.beilstein-journals.org/bjoc/single/articleFullText.htm?publicId=1860-5397-7-198#supporting-info",
"source": "Beilstein Journal of Organic Chemistry",
"Reason": "The target compound (2E)-1,3-Bis-(4-methoxyphenyl)-2-propen-1-one is a multifunctional molecule that includes two phenyl rings and additional functional groups such as alkenes and ethers. The basic benzene has a melting point of about 5.5 degrees Celsius. The presence of two 4-methoxyphenyl groups would increase the melting point as these groups enhance the intermolecular van der Waals forces due to their bulky nature and electron-donating properties; each adding approximately +70 to the melting point due to these interactions. The propenone segment of the molecule includes a carbon-oxygen double bond (C=O), which is polar and would introduce stronger dipole-dipole interactions; this could add about +20 to the melting point. The carbon-carbon double bond (C=C) is less polar and might contribute less to the melting point, estimated to be around +3. Adding these effects together gives a predicted melting point, which should be recalibrated because the prediction vastly exceeds the actual value.",
"Prediction": "99.0",
"Prediction(integer)": 99.0
}
]
理由の生成アルゴリズム
はじめは、化合物名、分子構造に対応するSMILES文字列、融点(mpC)、文献情報などしか記録されていません(1つ目のデータ)。
次に、GPT-4に指示を出すことで、「なぜ融点がXX℃になるのか」を考察させることで、「Reason」を生成し、そこから得られる「Prediction」を作ります。
(Predictionはテキストとして返されることがあるので、そこから数値を抜き出したのがPrediction(integer)です)。
理由生成のスクリプトはこちら。
一発で素晴らしいReasonとPredictionが生成されることは稀なので、回答を自分自身で修正させたり、ランダムシードを変えて同じ作業を行う、的なことを繰り返します(最大6回)。
何度か繰り返していくと、|実測-予測|<=10 くらいの誤差レベルになるので、そこで生成終了です。
上記の例では、3回目の試行で予測=実測となりました。
生成データの確認
コードはこちら。
生成データをクリーニングしつつ、予測誤差が10以下のケースを抜き出すと以下のプロットが得られました。いい感じにデータを作れています。
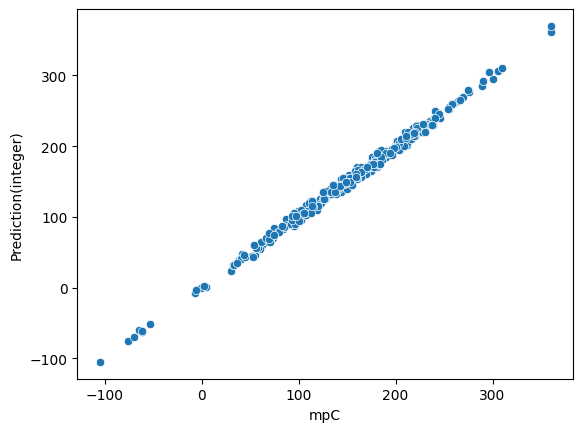
※注意
とりあえず予測はできてますが、生成されたReasonが、本当に化学的に正しいかどうかは検証していません。こじつけの可能性はあります。
プロンプトチューニングへの利用
アイデア
最終的には、一連のテキストをオープンソースモデルなどにファインチューニングで学習させ、Explainableな予測モデルを作るのが目標です。
今回は手始めに、GPT-4にプロンプトチューニングすることで予測性能に変化が生じるかを試しました。
コードはこちら (12/28 n_promptsを変えてなかったバグを修正)
ただし、すべてのデータをプロンプトに載せるとtoken数が大変なことになるので、今回はランダムに選んだn(=0,1,5,10)件をプロンプトに載せました。
結果
・ランダムに選んだ10件で予測を行いました。
・MSEを計算しました。
以下、n=0(オリジナルのGPT-4)、 1 (1件のみでプロンプトチューニング)、5、10のプロットです。
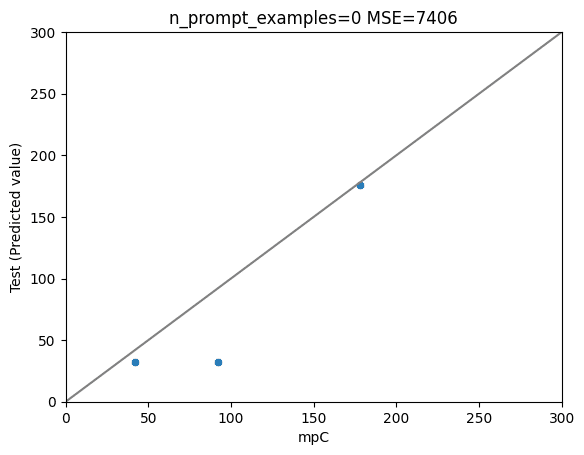
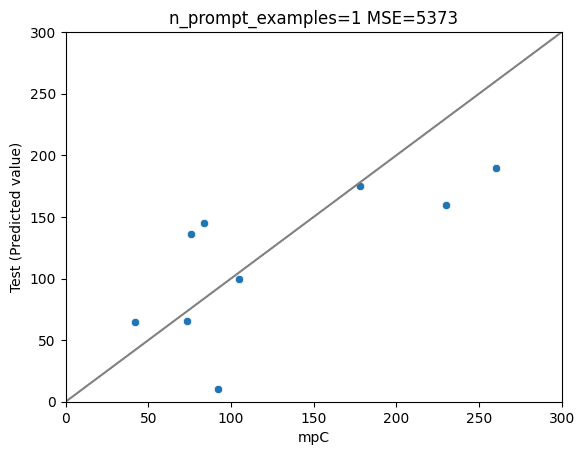
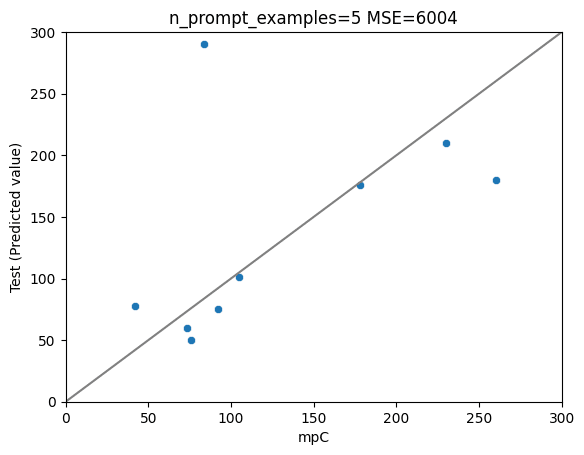
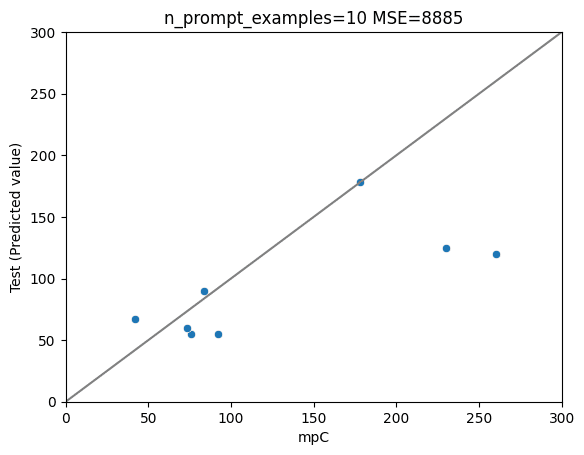
結果は、
1件のプロンプトチューニング(n=1) > オリジナル (n=0) > n=5 > n=10
でした。
n=10がベストな結果だと、嬉しかったのですが、そこまで世の中は甘くないようです。
考察
そこまで素晴らしい結果が得られていない理由としては、、、
n=0だと「わかりません」的な回答で濁してくる
GPT-4が単体でわりと賢いので、プロンプトチューニングの恩恵が薄い
言い換えると、オープンソースのモデルは鍛え甲斐がありそうです
プロンプトチューニングの内容と予測したい問題が離れている
例: プロントには「メチル基は+30℃の効果があります」というテキストが含まれているが、実際の問題ではメチル基ではなく水酸基の効果を考えたい、というケースが大半だと思います
今回のプロンプトチューニングは、知識をあたえるというよりは、思考の様式を揃える、という役割になっていたと考えられます
また、特定のプロントに下手に引っ張られて予測精度が下がった可能性も
多量のデータをファインチューニングで学習させる、というアプローチを取らないと、今回のアイデアの真価は発揮できないと考えています
今後のタスク
データセットの作成
500件程度のデータが得られています。一方でデータは全部で25kほどあるので、気長にGPT-4のAPIで計算を回します。
(70bクラスのローカルLLMで計算できると面白そうです)

ファインチューニング
とりあえずデータは揃ってきたので、Llama2あたりをファインチューニングしていければと思っています。