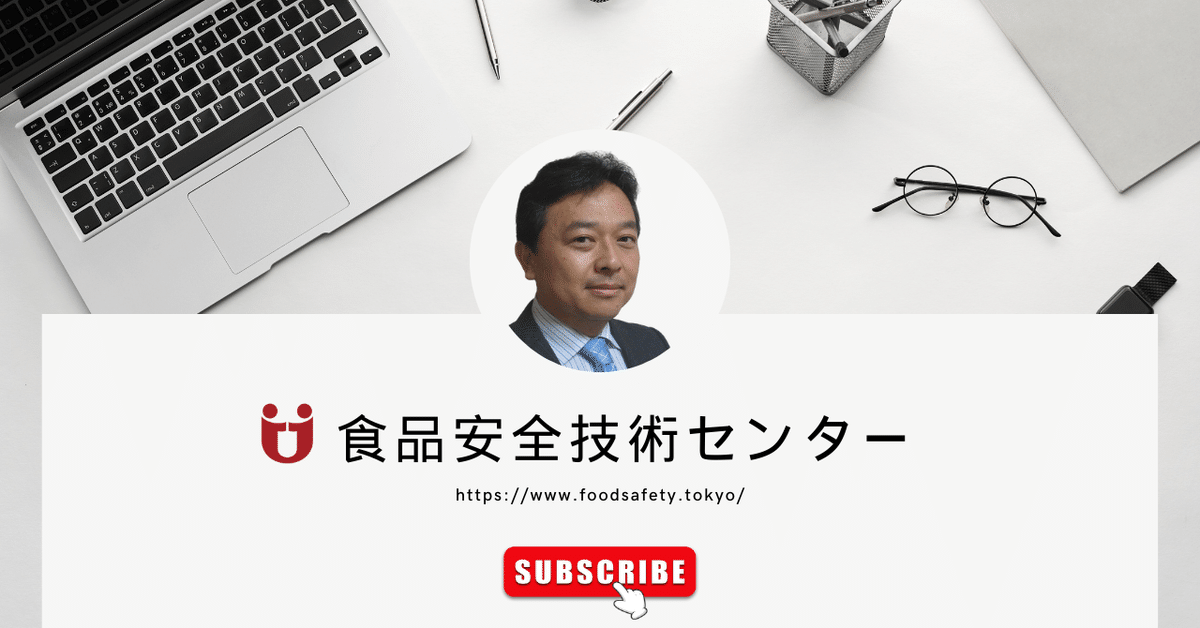
The AI Revolution: Five Innovative Technologies Paving the Way to the Future of Food Safety
The evolution of AI technologies is having a profound impact on the field of food safety.
These technologies are being applied beyond quality control and traceability to advanced risk assessment and predictive maintenance, and are bringing about new changes in the food industry. how AI is revolutionizing food safety will be explained with specific data and case studies.
1. Real-time Microbiological Detection: Fusion of AI and Computer Vision
Traditional microbiological testing methods rely primarily on culture methods and typically take 24-72 hours to obtain results.
This delay is a serious problem, especially when the safety of fresh or processed foods needs to be evaluated quickly.
However, recent advances in AI and computer vision technology have made real-time detection of microorganisms possible, leading to a major innovation in food safety management.
Technical Details:
Image analysis using convolutional neural networks (CNN) quickly and accurately identifies microbial colonies from microscopic images. This allows results to be obtained in just a few hours, compared to the several days required by traditional incubation methods.
The AI-based system learns the morphology and patterns of the microorganisms and uses this as a basis for assessing the risk of contamination in food in real time. This process is automated, ensuring highly accurate detection with minimal human intervention.
Advances in sensor technology also play an important role, for example, fluorescent labeling technology and Raman spectroscopy can be combined to detect specific pathogens in real time.
Application Example:
A research team at the University of California, Davis has developed an AI system that detects E. coli O157 in romaine lettuce within two hours with more than 99% accuracy. The system was found to detect contamination faster and more accurately than conventional methods, allowing contaminated products to be addressed before they reach the market.
In addition, the application of IBM's AI platform, Watson, in food factories has significantly reduced the occurrence of food recalls by predicting and preventing microbial contamination at an early stage.
Challenges and Prospects:
Further improvement of detection accuracy: Research is underway to improve accuracy under different environmental conditions. In particular, the challenge is to improve accuracy in the presence of low concentrations of pathogens and multiple microorganisms.
Data Standardization and Expansion: Training AI models requires large amounts of data, and datasets are being expanded to accommodate different microbial species and environmental conditions.
Regulation and legal certification: A legal framework will be required to officially certify AI detection results. This could lead to more widespread adoption of AI technologies and serve as a foundation for food safety.
The convergence of AI and computer vision is dramatically increasing the speed and accuracy of microbial detection and is becoming an essential tool in food safety management.
This enables rapid response throughout the food industry and lays a new foundation for providing safe, high-quality food to consumers.
2. enhancement of traceability through the integration of AI and IoT
The convergence of AI and Internet of Things (IoT) technologies is significantly improving the transparency and safety of the food supply chain.
This technological innovation enables real-time monitoring of food quality and safety, and immediate detection and response to any abnormalities that may occur.
Technology Details:
IoT sensors collect data such as temperature, humidity, and location in real time and transmit it to a cloud-based platform. This allows tracking of food conditions throughout the supply chain to ensure proper storage and transportation.
AI algorithms analyze big data collected from IoT sensors to detect unusual patterns and potential risks. This allows, for example, the risk of food deterioration or contamination caused by temperature fluctuations or vibrations during transportation to be predicted and countermeasures to be taken in real time.
In conjunction with blockchain technology, the entire food production process from production to consumer is recorded in a tamper-proof manner, enhancing traceability. This allows consumers to easily verify the product's origin and processing process, increasing their confidence in its safety.
Application Example:
Nestle has implemented the “IBM Food Trust” platform, which combines AI, IoT, and blockchain technologies to enhance traceability throughout the supply chain. This enables coffee beans and other food products to be tracked all the way from the farm to the consumer's doorstep, ensuring product safety and quality.
Walmart is similarly leveraging IoT sensors and AI for real-time monitoring throughout the supply chain. This allows the company to ensure that products are stored and transported at appropriate temperatures and to respond quickly to maintain quality.
Challenges and Prospects:
Initial investment and operational costs: The high initial investment and operational costs associated with implementing AI and IoT can make it difficult for small and medium-sized enterprises (SMEs) to adopt these technologies. Therefore, there is a need to develop cost-effective solutions.
Data Standardization: Data formats and protocols need to be standardized to ensure data compatibility between different systems in the supply chain. This will facilitate data sharing across different platforms and further enhance traceability.
Privacy and Security: Security and privacy protection of supply chain data is an important issue. Security measures are essential to prevent the risk of data hacking and the leakage of trade secrets.
The convergence of AI and IoT is becoming an essential tool in food supply chain management.
This allows food quality and safety to be monitored in real time, and a system is in place to respond immediately when anomalies are detected.
Future challenges are expected to include further technological advances as well as cost efficiency and data standardization.
3. analysis of consumer feedback using natural language processing (NLP)
AI-based Natural Language Processing (NLP) technology is a powerful tool for analyzing consumer feedback quickly and efficiently in the food industry.
In particular, by processing vast amounts of text data, such as social media and online reviews, in real time, potential food-related risks can be detected early and appropriate action can be taken.
Technical Details:
Sentiment Analysis: Sentiment analysis, one key application of NLP, quantifies how consumers feel about a product or service. This automatically categorizes positive and negative consumer feedback and provides metrics for companies to respond in real time.
Topic Modeling: Extracts key topics from large amounts of text data to identify issues and trends that consumers are particularly interested in. This approach enables companies to understand how widespread food safety concerns are and to respond quickly.
Anomaly Detection: Detects anomalous data that deviates from normal consumer feedback patterns to identify potential food safety-related issues early. This technology can help respond to, for example, a sudden increase in complaints or a spike in negative comments about a particular product.
Application Example:
Unilever uses NLP technology to analyze consumer feedback in real time to help develop new products and improve existing ones. For example, when postings about allergic reactions to certain foods increase, we can quickly review product information and take action as needed. This approach has been reported to improve consumer satisfaction and increase corporate credibility.
Coca-Cola has also implemented NLP technology to analyze consumer reactions to its products on social media. This information is used to adjust marketing strategies and generate ideas for product improvements, allowing the company to respond quickly to consumer needs.
Challenges and Prospects:
Multilingual: In the global marketplace, multilingual support is a key challenge. In order to analyze feedback in different languages simultaneously, NLP models must be developed specifically for each language.
Disinformation measures: Eliminating the impact of fake news and bot submissions is also important. This will provide accurate consumer insights and enable companies to take appropriate countermeasures.
Privacy Protection: Legal and ethical considerations regarding the handling of personal information are essential when analyzing consumer data. Anonymization of data and enhanced security measures can provide effective feedback analysis while maintaining consumer trust.
AI-based NLP technology has become an indispensable tool in the analysis of consumer feedback in the food industry.
It enables early identification of potential food safety risks and prompt and appropriate responses.
In the future, it is expected that multilingual support and improved data accuracy will be promoted. 4.
4) AI for Predictive Maintenance and Improved Production Efficiency
AI technology is bringing about a breakthrough in predictive maintenance (preventive maintenance) of food processing machinery.
Conventional maintenance methods rely on periodic inspections and repairs after a breakdown has occurred, but predictive maintenance using AI can minimize downtime and maximize production efficiency by analyzing machine sensor data in real time and predicting signs of breakdowns in advance.
Technology Details:
Machine learning models: Machine learning algorithms, especially random forests, support vector machines, and deep learning, are used to learn failure patterns from sensor data. This enables the detection of minute anomalies that would normally be missed and the detection of precursors to failure.
Use of sensor data: A variety of sensors are used to monitor machine operating conditions in detail, including vibration, temperature, sound, and current. These data are sent to AI systems for analysis in real time. Advances in sensor technology are providing more accurate data and improving the reliability of failure prediction.
Edge Computing: Real-time anomaly detection and immediate response can be achieved through the use of edge computing, which allows for instantaneous processing of data. This reduces time delays in sending data to the cloud and enables faster predictive maintenance.
Application Example:
Mars has implemented a “Digital Twin” system that leverages the Microsoft Azure IoT platform and AI technology. This system monitors the status of equipment in the factory in real time and can respond immediately when an abnormality is detected. This has resulted in a 50% reduction in unplanned downtime and millions of dollars in annual cost savings.
Coca-Cola has also implemented AI-based predictive maintenance to improve bottling line efficiency. Using machine learning models to detect anomalies in sensor data, Coca-Cola is maximizing line utilization by preventing equipment failures before they occur.
Challenges and Prospects:
Improving model accuracy: Improving the accuracy of machine learning models requires the collection and analysis of more diverse data sets. Robust models must also be developed that can handle complex machines and different operating conditions.
Data Quality Control: Quality control of sensor data is critical. Since sensor failures and anomalies affect the performance of predictive maintenance systems, a control process is needed to ensure data accuracy and consistency.
Skills shortage and education: Implementing and operating AI technologies requires personnel who are proficient in these technologies. However, the food industry lacks skills in AI and data science, so there is an urgent need to train personnel with expertise in these areas.
While predictive maintenance with AI can dramatically improve the efficiency and reliability of food processing machinery, effective implementation of these technologies also entails technical and human challenges.
Further development of AI models and training of human resources capable of operating them will be important for the future.
5. risk assessment and scenario analysis using AI
AI technology is bringing innovation to food safety management in the areas of risk assessment and scenario analysis.
Conventional risk assessment methods mainly calculate risk scores based on historical data, but AI technology has evolved further to predict future risk scenarios, opening up a new dimension of food safety.
Technology Details:
Machine Learning Algorithms: AI not only calculates risk scores based on historical risk data and relevant information, but also analyzes correlations among multiple risk factors using a combination of machine learning algorithms such as Bayesian networks, decision trees, and neural networks. This provides a comprehensive assessment of potential risk factors and predicts what risks will increase under specific conditions.
Scenario Analysis: AI uses Monte Carlo simulations to generate a myriad of hypothetical scenarios. This allows it to assess risk under a variety of conditions and predict the most likely risk scenario. For example, if a particular raw material is at risk due to extreme weather conditions or supply chain disruptions, the impact can be assessed in advance and appropriate countermeasures can be taken.
Application example:
SGS's Risk Intelligence tool is an AI-based food safety risk assessment system that integrates numerous data sources (e.g., regulatory information, scientific papers, news, social media) to provide a comprehensive risk assessment. Using this tool, companies can proactively identify potential food safety issues and quickly take action to address them. One major food manufacturer successfully reduced the number of recalls by 60% as a result of implementing this tool.
Mars uses AI to predict the risk of aflatoxin contamination and manage risk throughout the raw material supply chain. ai models use highly accurate weather and geospatial data to predict the risk of aflatoxin formation, helping farmers and suppliers manage risk in advance. The AI models use highly accurate meteorological and geospatial data to predict aflatoxin production risk, helping farmers and suppliers manage risk in advance.
Challenges and Prospects:
Data bias and bias: The accuracy of risk assessment by AI depends on the quality of the data used. If the data is biased, the model will also be biased, requiring rigorous data collection and management processes. It is also important to ensure data uniformity across different regions and food categories.
Interpretability of Predictions: For AI predictions to be adopted with confidence by companies and regulatory agencies, the results must be interpretable and explainable. For complex AI models, the challenge is how to interpret the results and how to incorporate them into decision making.
Regulatory compliance: risk assessment with AI needs to be done within a legal and ethical framework. This includes the development of guidelines to ensure that decisions based on AI predictions are appropriate and legal.
AI risk assessment and scenario analysis offers a new perspective on food safety management, allowing companies to manage risk more proactively and preventively.
In the future, the combination of higher quality data and advanced analytical techniques is expected to further improve the accuracy and reliability of risk management.
Future Forecasts and Issues to be Addressed
Future Projections:.
AI technology will improve safety and efficiency at all stages of food production, processing, distribution, and consumption.
Precise and rapid quality control by AI is expected to become widespread, significantly reducing food-related risks.
Prediction of risk scenarios and real-time monitoring will expand, and proactive food safety management will become the norm.
Issues to be addressed: 1.
Data quality control
Accurate and consistent data management is essential to improve the accuracy of AI decisions.
Strict controls must be established to minimize data bias and errors.
Transparency and accountability of technology
Make AI decision-making processes easy to understand and ensure reliability.
Especially in food safety, transparency is essential because AI decisions may affect human lives.
Regulatory Compliance
Clear guidelines need to be developed by regulators to ensure that risk management based on AI technology is legally appropriate.
Standards for the use of AI should be developed to maintain safety standards throughout the food industry.
Human Resource Development
There is an urgent need to develop specialized human resources (technicians, data scientists, and food scientists) who can operate AI technology.
Through human resource development, AI and human knowledge will be effectively integrated to build a sustainable food safety system.
Through these efforts, AI technology can be used to improve safety in the food industry.
この記事が気に入ったらサポートをしてみませんか?