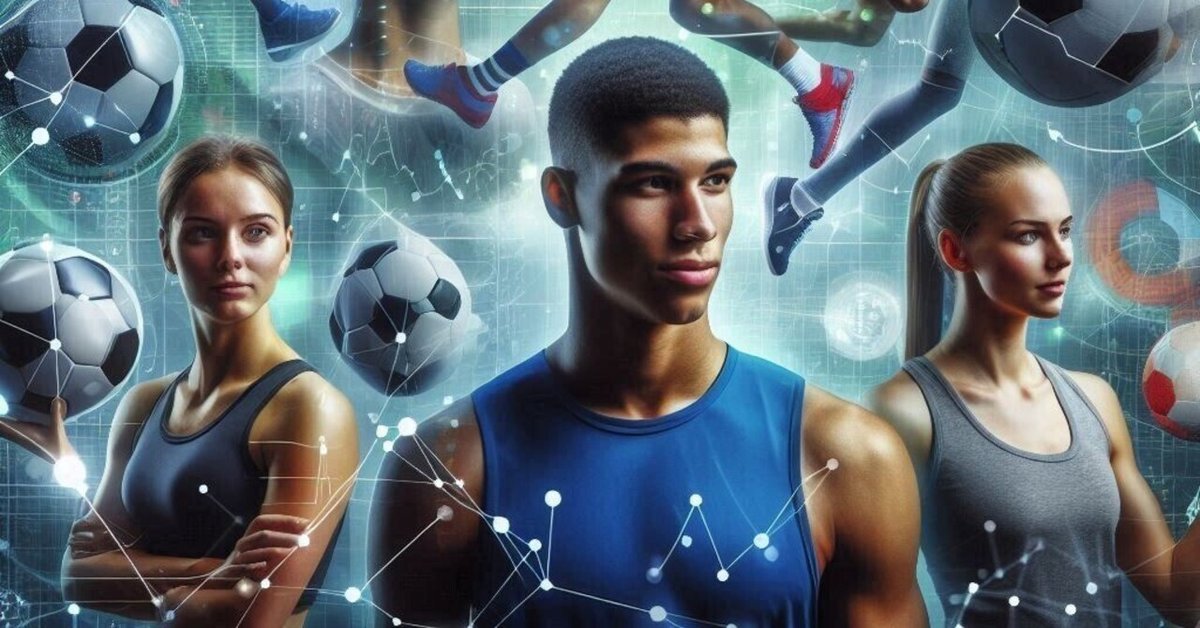
Data and Sports
Data analysis has become an indispensable element in modern sports. Utilizing data in various competitions has enabled performance improvement for athletes, strategy formulation, and optimization of team management. Below, I will explain in detail the relationship between data and sports, including specific examples.
Examples of Data Analysis Application
Baseball: Sabermetrics
In the baseball world, a statistical analysis method called sabermetrics is widely used. This method analyzes not only basic statistics like batting average and home runs but also detailed data such as on-base percentage and slugging percentage to evaluate players' true value. A specific example is the approach taken by Billy Beane at the Oakland Athletics in MLB. Beane successfully rebuilt the Athletics, which was a weak team, by utilizing data analysis.There is also a movie on Netflix about this data-driven baseball approach:"Moneyball | Sony Pictures Official
Starring Brad Pitt, from the team behind 'The Social Network.' This is the true story that overturned Major League Baseball's common sense."
Soccer: Utilization of GPS Data
In soccer, GPS sensors are used to analyze players' movements and physical output in detail. For instance, J-League's Giravanz Kitakyushu has installed 10 cameras in their training ground, constantly filming and analyzing players' movements using AI. Utilizing this data yields the following effects:
Improvement of player performance
Formulation of effective tactics
Reduction of injury risks
Basketball: Shot Analysis
In the NBA, a player tracking system is used to analyze players' positions and ball movement in detail. This allows for detailed understanding of individual stats such as shot success rates and rebound numbers, which are used to improve tactics and performance.
Advantages of Data Analysis
Data analysis in sports offers the following advantages:
Objective decision-making: Using concrete data based on numbers rather than just feelings or intuition allows for clearer and fairer judgments
Performance optimization: By quantifying players' strengths and weaknesses, effective training menus can be devised
Improved accuracy in strategy planning: More effective strategies can be formulated by analyzing opponent teams and understanding one's own team's tendencies
Injury prevention: By analyzing players' physical data, excessive loads can be avoided, reducing the risk of injuries
Challenges in Data Analysis
On the other hand, data analysis in sports also faces the following challenges:
Data quality: Using inaccurate or biased data can lead to incorrect conclusions
Need for expertise: Data analysis requires knowledge of statistics and programming, which not all stakeholders can easily utilize
Neglect of human elements: Over-reliance on data may lead to underestimating factors that are difficult to quantify, such as players' intuition, experience, and team atmosphere
Future Prospects
With the development of AI and machine learning, the accuracy and scope of sports data analysis are expected to expand further. For example, real-time tactical suggestions and more precise injury predictions may become possible. Additionally, with the evolution of wearable technology, collection of more detailed biometric data will be possible, which can be used for managing players' conditions and devising optimal training plans.Data analysis will contribute not only to improving sports performance but also to strengthening fan engagement and developing sports businesses. However, balancing data-driven decisions with human intuition and experience will remain an important challenge in the future.