ここから先は
1,369字
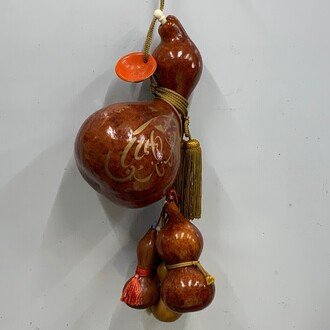
2023年第3四半期(7月−9月)のエネルギー問題や環境問題を中心に,現代社会の論点を考えるヒントやシーズをご提供します。京都の隠れた愉しみも随所で紹介しています。日本語と英語の2か国語で表記。
The text is presented in both Japanese and English, making it a potentially useful resource for learning both languages.
『京都居候叢書』(きょうといそうろうそうしょ)第3篇(2023年7月−9月)。京都に居候をしながら,政治経済,ビジネス,エネルギー,環境,…
期間限定!Amazon Payで支払うと抽選で
Amazonギフトカード5,000円分が当たる
Amazonギフトカード5,000円分が当たる
Thank you very much!