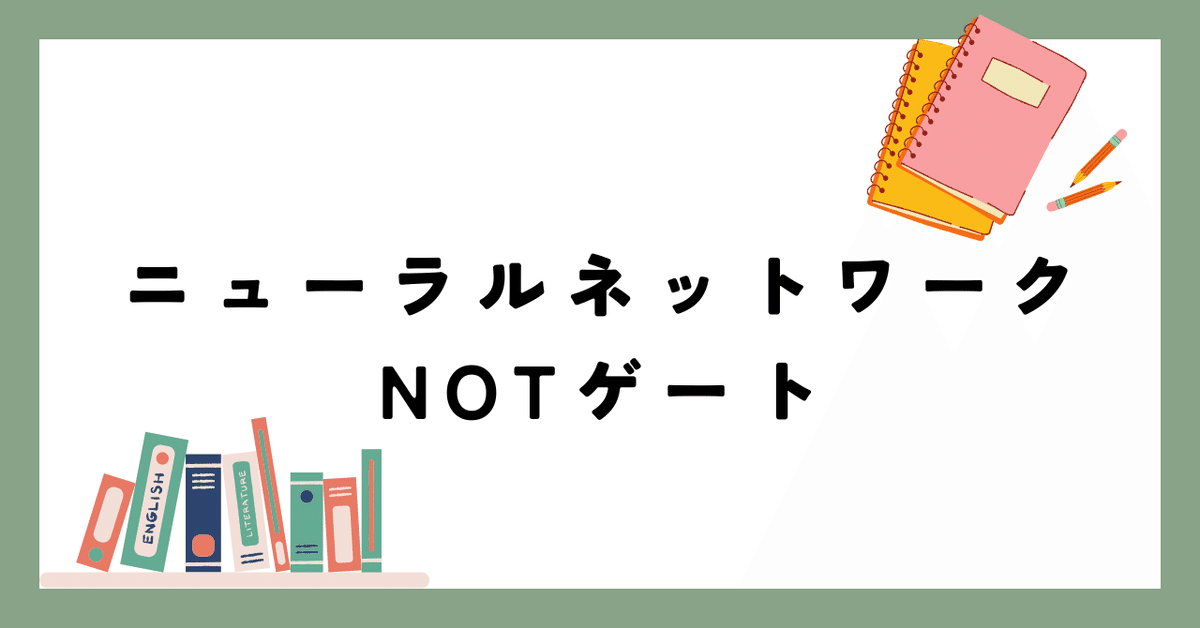
NOTゲート ニューラルネットワーク
考え方
図1の真理値表のようにNOTゲートは入力が1つである。入力の組み合わせは2つなので、それを1セットとする。
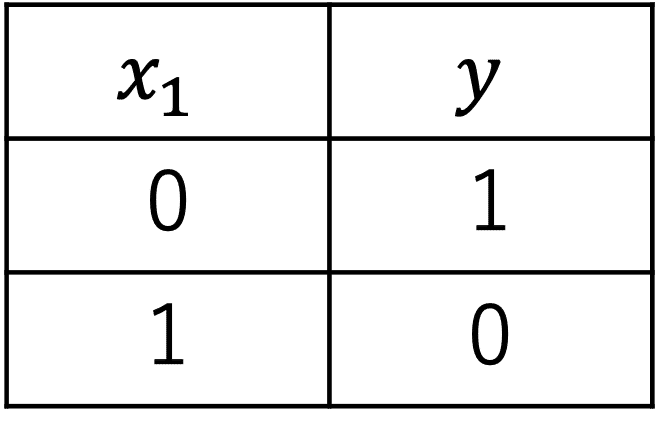
モデルがNOTゲートを学習できたかどうかは、1セットごとに判定していく。
プログラム
import pandas as pd
import numpy as np
def counterup(x, delta_x):
if len(set(delta_x)) == 1:
x = False
else:
x = True
return x
# 誤り訂正学習法
# 初期パラメータの設定
x1data = [0,1]
tdata = [1,0]
y = 1
w1 = 0
theta = 0
delta_w1 = []
delta_theta = []
count_w1 = True
count_theta = True
# 2つを1単位とするためのカウンター
counter = -1
# 試行回数のカウンター
procount = 0
while count_w1 or count_theta:
procount = procount + 1
if counter == 1:
counter = 0
delta_w1 = []
delta_theta = []
else:
counter = counter+1
# データセット
x1 = x1data[counter]
t = tdata[counter]
# 予測モデルの出力
if w1*x1-theta >= 0:
y = 1
else:
y = 0
# 変化分の計算
delta_w1.append((t-y)*x1)
delta_theta .append(-(t-y))
# 経過表示
# print(procount, x1, t, w1, theta, y, t-y, delta_w1[counter], delta_theta[counter])
if procount == 1:
result = np.array([procount, x1, t, w1, theta, y, t-y, delta_w1[counter], delta_theta[counter]])
else:
add_data = np.array([procount, x1, t, w1, theta, y, t-y, delta_w1[counter], delta_theta[counter]])
result = np.vstack([result, add_data])
# 重みと閾値の再計算
w1 = w1 + delta_w1[counter]
theta = theta + delta_theta[counter]
# 変化の検出
if counter == 1:
count_w1 = counterup(count_w1, delta_w1)
count_theta = counterup(count_theta, delta_theta)
# エクセル保存
result_df = pd.DataFrame(result, columns=["k","x1","t","w1","theta","y","t-y","delta_w1","delta_theta"])
result_df.to_excel("./NOT学習結果.xlsx", index=False)
関連記事
ニューラルネットワーク 単純モデル
https://note.com/elemag/n/n7bf117b1c818?sub_rt=share_pw
サイト
https://sites.google.com/view/elemagscience/%E3%83%9B%E3%83%BC%E3%83%A0