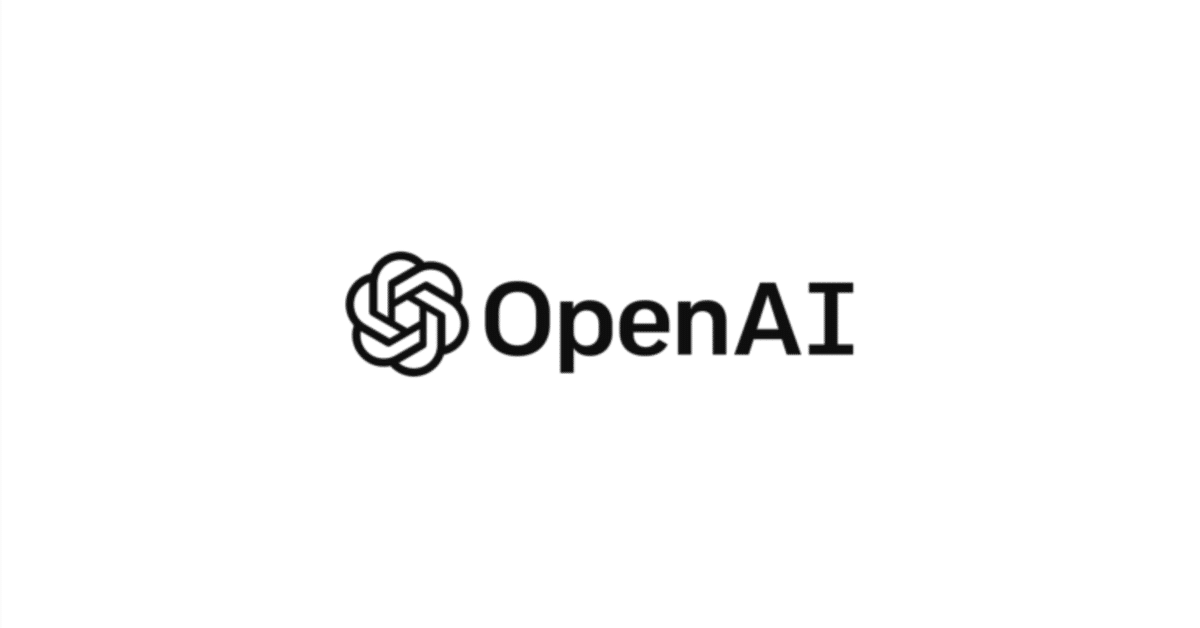
【OpenAI】英語解説を日本語で読む【2023年4月27日|@Stanford eCorner】
OpenAIの共同創設者兼チーフサイエンティスト、イリヤ・スツケヴェル氏がスタンフォード大学非常勤講師のラヴィ・ベラーニとの対談で、OpenAIや一般的なAI企業における複雑な意思決定のアプローチについて説明し、深層学習の将来について予測しています。
公開日:2023年4月27日
※動画を再生してから、インタビューを読むのがオススメです。
Who you are defines how you build.
あなたが誰であるかによって、あなたがどのように構築するかが決まります。
Welcome YouTube and Stanford communities to the Entrepreneurial Thought Leaders Seminar, brought to you by STVP, the Entrepreneurship Center in the School of Engineering at Stanford, and BASIS, the Business Association of Stanford Entrepreneurial Students.
スタンフォード大学工学部のアントレプレナーシップ・センターであるSTVPとスタンフォード起業家学生のビジネス・アソシエーションであるBASISがお届けするアントレプレナー・ソートリーダーズ・セミナーへ、YouTubeとスタンフォードのコミュニティの皆様をお迎えします。
Today, we are so honored to have Ilya Sutskever here at ETL.
本日は、イリヤ・スーツケバー氏にETLにお越しいただき、大変光栄に思っています。
Ilya is the co-founder and chief scientist of OpenAI, which aims to build artificial general intelligence for the benefit of all humanity.
イリヤさんは、全人類のために人工知能を構築することを目的としたOpenAIの共同設立者でありチーフサイエンティストです。
Elon Musk and others have cited that Ilya is the foundational mind behind the large language model generative pre-trained Transformer 3, or GPT-3, and its public facing product, ChatGPT.
イーロン・マスクなどは、イリヤが大規模言語モデルの生成前訓練型トランスフォーマー3(GPT-3)とその公開製品であるChatGPTの基礎を築いた人物であることを挙げています。
A few product releases have created as much excitement, intrigue, and fear as the release of ChatGPT in November of 2022.
2022年11月のChatGPTのリリースほど、興奮と陰謀と恐怖を生み出した製品リリースはないでしょう。
Ilya is another example of how the US and the world has been the beneficiary of amazing talent from Israel and Russia.
イリヤは、米国と世界がイスラエルとロシアから素晴らしい才能の恩恵を受けてきたことを示すもう一つの例です。
Ilya was born in Russia, and then when he was five, he moved to Israel, where he grew up.
イリヤはロシアで生まれ、5歳の時にイスラエルに移り住み、そこで育ちました。
And he spent the first half of undergrad even in Israel, and then he transferred and went to the University of Toronto to complete his bachelor's degree in mathematics.
そして彼は学部生の前半をイスラエルで過ごし、その後転校してトロント大学に進学し、数学の学士号を取得しました。
He went on to get a master's and PhD in computer science from the University of Toronto, and then came over here to the farm and did a short stint with Andrew Ng before returning back to Toronto to work under his advisor, Jeffrey Hintz Research Company, DNN Research.
彼はトロント大学で修士号と博士号を取得し、その後スタンフォード大学でアンドリュー・ングと共に短期間過ごしました。その後、彼の指導教官であるジェフリー・ヒントンの研究会社DNNリサーチで働くためにトロントに戻りました。
Google then acquired DNN Research shortly thereafter in 2013, and Ilya became a research scientist as part of Google Brain.
その後、Googleは2013年にDNN Researchを買収し、イリヤはGoogle Brainの一員としてリサーチサイエンティストとなった。
And in 2015, he left Google to become a director of the then newly formed OpenAI.
そして2015年、Googleを離れ、当時設立されたばかりのOpenAIのディレクターに就任した。
It's hard to overestimate the impact that ChatGPT has had on the world since its release in November of last year.
昨年11月のリリース以来、ChatGPTが世界に与えたインパクトは過大評価しがたいものがあります。
And while it feels like ChatGPT came out of nowhere to turn the world on its head, the truth is there was a deep history of innovation that has led to that moment.
そして、ChatGPTはどこからともなく現れて世界をひっくり返したように感じるが、実はその瞬間に至るまでには、深いイノベーションの歴史があった。
And as profound as ChatGPT is, Ilya is no stranger in uttering in discontinuous leaps of innovation in AI.
そして、ChatGPTが深遠であるのと同様に、イリヤはAIのイノベーションの非連続的な飛躍を口にすることにかけては、決して他人事ではありません。
Jeff Hinton has said that Ilya was the main impetus for AlexNet, which was the convolutional neural network in 2012 that is attributed to setting off the deep learning revolution that has led to the moment that we are now in.
ジェフ・ヒントンは、イリヤが2012年に畳み込みニューラルネットワークであるAlexNetの主なきっかけを作り、今の瞬間につながる深層学習革命を起こしたと述べている。
And of course, it was seven years since the founding of OpenAI that ChatGPT was finally unleashed to the world.
そしてもちろん、ChatGPTがついに世界に解き放たれたのは、OpenAIの設立から7年目のことでした。
Ilya was elected a fellow of the Royal Society in 2022.
イリヤは2022年に英国王立協会のフェローに選出された。
He's been named to the MIT Tech Review 35 under 35 list in 2015.
2015年にはMIT Tech Review 35 under 35に選ばれている。
He's received the University of Toronto's Innovator of the Year Award in 2014, and the Google Graphic Scholarship from 2010 to 2012.
2014年にトロント大学のInnovator of the Year Award、2010年から2012年までGoogle Graphic Scholarshipを受賞しています。
So with that, everybody, please give a virtual warm round of applause and welcome for Ilya to the Entrepreneurial Thought Leaders Seminar.
それでは皆さん、仮想の温かい拍手で、イリヤを起業家ソートリーダーズセミナーに迎えてください。
So Ilya, imagine lots of applause and you're always invited back onto the farm physically whenever you are able.
イリヤ、たくさんの拍手をお願いします!そして、いつでも農場に戻ってきてください。
So Ilya, there's so much to discuss, and I know we're gonna have so little time and we have quite a broad range of fluency around the audience in terms of ChatGPT and large language models.
イリヤ、話したいことは山ほどあるのですが、時間がないのは分かっていますし、ChatGPTや大規模言語モデルに関しても、聴衆の皆さんはかなり幅広い流暢さをお持ちですから。
I wanted to start off with just a quick question on the technology, which is just the key technology underlying OpenAI and generative AI more broadly is large language models.
OpenAIや生成AIの根幹をなす重要な技術として、大規模言語モデルがありますが、その技術について簡単に説明してください。
Can you describe the technology in simple terms?
この技術を簡単に説明できますか?
And now that you're at the forefront of the tech, can you share what has surprised you the most about what the tech can do that you didn't anticipate?
また、この技術の最前線にいる今、この技術でできることで、予想外に驚いたことがあれば教えてください。
Yeah.
そうですね。
I can't explain what this technology is and why it works.
この技術がどういうもので、なぜ機能するのかを説明することはできません。
I think the explanation for why it works is both simple and extremely beautiful.
なぜ機能するのかの説明は、シンプルであり、かつ非常に美しいと思います。
And it works for the following reason.
そして、それは次のような理由で機能するのです。
So you know how the human brain is our best example of intelligence in the world.
人間の脳が、世界で最も優れた知性の例であることはご存じでしょう。
And we know that the human brain is made out of a large number of neurons, a very, very large number of neurons.
そして、人間の脳は非常に多くのニューロンからできていることも知っています。
A very, very large number of neurons.
非常に、非常に多くのニューロンです。
Neuroscientists have studied neurons for many decades to try to understand how they work precisely.
神経科学者は何十年にもわたってニューロンを研究し、その仕組みを正確に理解しようとしてきました。
And while the operation of our biological neurons are still mysterious, there's been a pretty bold conjecture made by the earliest deep learning researchers in the forties.
そして、私たちの生物学的なニューロンの動作はまだ謎に包まれていますが、40年代に最も初期の深層学習の研究者たちによって、かなり大胆な推測がなされてきました。
The idea that an artificial neuron, the ones that we have in our artificial neural networks, kind of sort of similar to a biological neuron if you squint.
人工ニューロン、つまり私たちの人工ニューラルネットワークにあるものは、目を細めると生物学的なニューロンに似ている、というものです。
So there's an assumption there.
つまり、そこには仮説があるのです。
And we can just run with this assumption.
そして、この仮定をもとに実行すればいいのです。
Now, one of the nice things about these artificial neurons is that they are much simpler and you can study them mathematically.
さて、このような人工ニューロンの良いところは、よりシンプルで数学的に研究できることです。
And a very important breakthrough that was done by the very, very early deep learning pioneers before it was known as deep learning was the discovery of the back propagation algorithm, which is a mathematical equation for how these artificial neural networks should learn.
非常に初期のディープラーニングの先駆者たちによって行われた非常に重要なブレークスルーの一つは、バックプロパゲーションアルゴリズムの発見でした。これは、人工ニューラルネットワークがどのように学習すべきかに関する数学的な式です。
It provides us with a way of taking a large computer and implementing this neural network in code.
このアルゴリズムによって、大型コンピュータでニューラルネットワークをコードで実装することができるようになりました。
And then there would be, there is an equation that we can code up that tells us how this neural network should adapt its connections to learn from experience.
そして、このニューラルネットワークが経験から学ぶために、どのように接続を適応させるべきかを示す方程式をコード化することができるようになったのです。
Now, a lot of additional further progress had to do with understanding just how good and how capable this learning procedure is and what are the exact conditions under which this learning procedure works well.
さて、さらなる進歩の多くは、この学習手順がどの程度優れていて、どの程度の能力があるのか、また、この学習手順がうまく機能する正確な条件は何なのかを理解することと関係がありました。
Although we do with computers, it was a little bit of an experimental science, a little bit like biology.
コンピュータを使ったとはいえ、これは少し実験的な科学で、生物学に少し似ています。
We have something that is like a biological experiment a little bit.
私たちは、生物学的な実験に少し似ているものを手に入れました。
And so then a lot of the progress with deep learning basically boils down to this.
そして、ディープラーニングの進歩の多くは、基本的にこれに集約されるわけです。
We can build these neural networks in our large computers and we can train them on some data.
大型コンピュータでニューラルネットワークを構築し、いくつかのデータでそれを訓練することができます。
We can train those large neural networks to do whatever it is that the data asks them to do.
その大規模なニューラルネットワークを訓練して、データから要求されたことは何でもできるようにするのです。
Now, the idea of a large language model is that if you have a very large neural network, perhaps one that's now not that far from, like these neural networks are pretty large and we train them on the task to guess the next word from a bunch of previous words in text.
さて、大規模言語モデルの考え方は、非常に大規模なニューラルネットワーク、おそらく今ではそれほど遠くないもの、例えばこれらのニューラルネットワークはかなり大規模で、テキスト内の前の単語の束から次の単語を推測するというタスクで訓練する、というものです。
So this is the idea of a large language model.
これが大規模言語モデルの考え方です。
You train a big neural network to guess the next word from the previous words in text.
大きなニューラルネットワークを訓練して、テキスト中の前の単語から次の単語を推測させるのです。
And you want the neural network to guess the next word as accurately as possible.
そして、ニューラルネットワークには、できるだけ正確に次の単語を推測してもらいたい。
Now, the thing that happens here is we need to come back to our original assumption that maybe biological neurons aren't that different from artificial neurons.
ここで、「生物学的なニューロンと人工的なニューロンはそれほど違わないのではないか」という最初の仮説に立ち返る必要があります。
And so if you have a large neural network like this that guesses the next word really well, maybe it will be not that different from what people do when they speak.
つまり、このような大規模なニューラルネットワークがあり、次の単語を実にうまく推測するのであれば、それは人が話すときに行うこととそれほど違わないかもしれないのです。
And that's what you get.
そして、それが実現するのです。
So now when you talk to a neural network like this, it's because it has such a great, such an excellent sense of what comes next, what word comes next.
このようなニューラルネットワークと話すと、次に何が来るか、どんな単語が来るかについて、とても優れた感覚を持つからです。
It can narrow down, it can't see the future, but it can narrow down the possibilities correctly from its understanding.
未来を見ることはできませんが、その理解から可能性を正しく絞り込むことができます。
Being able to guess what comes next very, very accurately requires prediction, which is the way you operationalize understanding.
次に来るものを非常に正確に推測できるようになるには、予測することが必要です。
What does it mean for a neural network to understand?
ニューラルネットワークが理解するとはどういうことでしょうか?
It's hard to come up with a clean answer, but it is very easy to measure and optimize the network's prediction error of the next word.
きれいな答えを出すのは難しいですが、ネットワークの次の単語の予測誤差を測定して最適化するのは非常に簡単です。
So we say we want understanding, but we can optimize prediction.
つまり、「理解すること」と言っても、「予測を最適化すること」はできるのです。
And that's what we do.
そして、それを実行するのです。
And that's how you get these current large language models.
こうして、現在の大規模言語モデルができあがったのです。
These are neural networks, which are large, they are trained with the back propagation algorithm, which is very capable.
これはニューラルネットワークで、大規模なものですが、バックプロパゲーション・アルゴリズムで訓練されており、非常に高性能なものです。
And if you allow yourself to imagine that an artificial neuron is not that different from a biological neuron, then yeah, like our brains are capable of doing a pretty good job at guessing the next word if you pay very close attention.
人工の神経細胞は生物学的な神経細胞とさほど変わらないと想像すれば、私たちの脳は、細心の注意を払えば、次の単語を推測するのに非常に優れた仕事をすることができるのです。
So.
ですから。
So I love that.
私はそれが大好きです。
And I just wanna make this more concrete.
そして、これをもっと具体的にしたいんです。
So just to push that analogy further between the biological brain and these neural analog, physical networks, digital networks.
生物学的な脳と、神経アナログ、物理的なネットワーク、デジタル・ネットワークとの間のアナロジーをさらに推し進めたい。
If the human, if we consider, before it was considered untenable for these machines to learn, now it's a given that they can learn or do this, do predictive outcomes of what's gonna come next.
もし人間が、以前は機械が学習するのは無理だと考えられていたのに、今では学習したり、次のことを予測したりするのは当たり前になっている、と考えてみましょう。
If a human is at one X learning, and you have the visibility into the most recent chat GPT models, what would you put the most recent chat GPT model as a ratio of where the humans are at?
もし人間が1Xの学習をしているとして、最新のチャットGPTモデルが見えるとしたら、人間の学習状況に対する最新のチャットGPTモデルの比率はどうでしょうか。
So if humans are at one X, what's chat GPT at?
つまり、人間が1Xに達しているとしたら、チャットGPTは何Xに達しているのか?
You know, it's a bit hard to make direct comparisons between our artificial neural networks and people, because at present, people are able to learn more from a lot less data.
ご存知のように、私たちの人工ニューラル ネットワークと人間を直接比較するのは少し難しいです。現在、人間ははるかに少ないデータからより多くのことを学ぶことができるからです。
This is why these neural networks like chat GPT are trained on so much data to compensate for their initial slow learning ability.
そのため、チャットGPTのようなニューラルネットワークは、最初の学習能力の遅さを補うために、非常に多くのデータで訓練されます。
You know, as we train these neural networks and we make them better, faster learning abilities start to emerge.
このようなニューラルネットワークを訓練し、より良いものにしていくと、より速い学習能力が現れ始めるんですね。
But overall, overall, it is the case that we are quite different.
しかし、全体的に見れば、全体的に見れば、かなり違うということです。
The way people learn is quite different from the way these neural networks learn.
人の学習方法は、このニューラルネットワークの学習方法とは全く異なります。
Like one example might be, you know, these neural networks, they are, you know, solidly good at math or programming, but like the amount of math books they needed to get, let's say, good at something like calculus is very high.
例えば、このニューラルネットワークは、数学やプログラミングには強いのですが、例えば微積分のようなものを得意にするために必要な数学書の量は非常に多いのです。
Whereas a person would need a fairly, you know, two textbooks and maybe 200 exercises and you're pretty much good to go.
しかし、例えば微積分のようなものを得意にするために必要な数学の本の量は非常に多いのです。
So there is still-
だから、まだ...
But just to get an order of magnitude sense, if you relax the data constraint, so if you let the machine consume as much data as it needs, do you think it's operating at like one-tenth of a human right now or?
しかし、桁違いの感覚を得るために、データ制約を緩和し、機械が必要なだけのデータを消費させた場合、今現在、人間の10分の1程度で動作していると思われますか?
You know, it's quite hard to answer that question still.
この質問に答えるのは、まだかなり難しいですね。
And let me tell you why I hesitate to, like I think that any figure like this will be misleading.
なぜかというと、このような数値は誤解を招くと思うからです。
And I wanna explain why.
その理由を説明したいと思います。
Like, because right now, any such neural network is obviously very superhuman when it comes to the breadth of its knowledge and to the very large number of skills that these neural networks have.
というのも、今現在のニューラルネットワークは、その知識の広さと、ニューラルネットワークが持つ非常に多くのスキルに関して、明らかに超人的だからです。
For example, they're very good at poetry and they're very, they know like, they can talk eloquently about any topic pretty much.
例えば、ニューラルネットワークは詩がとても上手で、どんな話題でも雄弁に語ることができるんです。
And they can talk about historical events and lots of things like this.
そして、歴史的な出来事や、このような様々なことについて話すことができます。
On the other hand, people can go deep and they do go deep.
一方、人は深く掘り下げることができますし、実際に深く掘り下げます。
So you may have an expert, like someone who understands something very deeply despite having read only a small amount of documents, let's say, on the topic.
例えば、そのテーマについてほんの少ししか文献を読んでいないにもかかわらず、何かを非常に深く理解しているような専門家がいるかもしれません。
So because of this difference, I really hesitate to answer the question in terms of, oh yeah, it's like some number between zero and one.
このような違いがあるため、「0と1の間の数」という意味で質問に答えるのは、本当にためらわれますね。
Do you think there is a singularity point where the machines will surpass the humans in terms of the pace of learning and adaption?
学習や適応のスピードにおいて、機械が人間を超えるシンギュラリティポイントはあると思いますか?
Yes.
そうですね。
Yeah, and when do you think that point will occur?
その時期はいつだと思いますか?
I don't know.
わかりません。
I don't know when it will occur.
いつ起こるかはわかりません。
I think some additional advances will need to happen, but I absolutely would not bet against this point occurring at some point.
さらなる進化が必要だと思いますが、ある時点でこのポイントが発生することに賭けてはいけないと思います。
Can you give me a range?
範囲を教えてください。
Is it at some point next month?
来月のある時点でしょうか?
Is it next year?
来年でしょうか?
I think it's like the uncertainty on these things is quite high because these advances, I can imagine it can take in quite a while.
というのも、これらの進歩は、かなり時間がかかると想像できるからです。
I can imagine it can take in a disappointing long time.
残念なほど長い時間がかかることは想像できます。
I can also imagine it's taking some number of years, but it's very hard to give a calibrated answer.
また、何年かかかるということも想像できますが、較正された答えを出すのは非常に困難です。
And I know there's lots to push forward, so I'm gonna ask one more question then move on to some of the other issues.
しかし、その答えを出すのはとても難しいことだと思います。
But I read that when you were a child, you were disturbed by the notion of consciousness.
しかし、あなたが子供の頃、意識という概念に悩まされたという話を読みました。
And I wasn't sure what that word meant disturbed, but I'm curious, do you view consciousness or sentience or self-awareness as an extenuation of learning?
しかし、あなたは意識や感覚、自己認識を学習の延長としてとらえているのでしょうか?
Do you think that that is something that also is an inevitability that will happen or not?
また、それは必然的に起こるものだと思いますか、そうではありませんか?
Yeah, I mean, on the consciousness questions, like, yeah, I was, as a child, I would look into my hand and I would be like, how can it be that this is my hand that I get to see?
そうですね、意識の質問で、そうですね、子供の頃、自分の手を覗き込んで、「これが自分の手だなんて、どうしたらいいんだろう」と思っていました。
Like something of this nature, I don't know how to explain it much better.
このような性質のもので、どう説明したらいいのかわかりません。
So that's been something I was curious about.
だから、そういうことに興味があったんです。
You know, it's tricky with consciousness because how do you define it?
意識というのは厄介なもので、どう定義すればいいのでしょう?
It's something that eluded definition for a long time.
長い間、定義されなかったものです。
And then how can you test it in a system?
そして、それをシステムでテストするにはどうしたらいいのでしょう?
Maybe there is a system which acts perfectly right, but perfectly the way you'd expect if a conscious system would act yet, maybe it won't be conscious for some reason.
もしかしたら、完璧に正しい行動をするシステムがあるかもしれません。しかし、意識のあるシステムであれば、完璧に期待通りの行動をするはずですが、何らかの理由で意識がないのかもしれません。
I do think there is a very simple way to, there is an experiment which we could run on an AI system, which we can't run just yet, but maybe in like the future point when the AI learns very, very quickly from less data, we could do the following experiment.
まだ実行できませんが、AIが少ないデータから非常に素早く学習するようになった将来の時点で、次のような実験ができるかもしれません。
Very carefully, we'd very carefully curate the data such that we never ever mention anything about consciousness.
非常に慎重に、意識について決して言及しないようにデータを管理します。
We would only say, you know, here is a ball and here's a castle and here is like a little toy.
ここにボールとお城があり、ここに小さなおもちゃがあります」と言うだけです。
Like we would imagine you'd have data of this sort and it would be very controlled.
このようなデータがあるとすれば、それは非常に管理されたものであることが想像されます。
Maybe we'd have some number of years worth of this kind of training data.
おそらく、何年分かのこのようなトレーニングデータがあるはずです。
Maybe it would be, maybe such an AI system would be interacting with a lot of different teachers, learning from them, but all very carefully.
おそらく、このようなAIシステムは、多くの異なる教師と対話し、彼らから学びますが、すべて非常に慎重に行われるでしょう。
You never ever mention consciousness.
あなたは決して意識について言及しませんね。
You don't talk about, people don't talk about anything except for the most surface level notions of their experience.
人々は、自分の経験に関する最も表面的な概念以外、何も話しません。
And then at some point you sit down this AI and you say, okay, I want to tell you about consciousness.
そしてある時、あなたはこのAIを座らせて、「意識について話したい」と言うのです。
It's this thing that's a little bit not well understood.
意識というのは、ちょっとよく分かっていないものです。
People disagree about it, but that's how they describe it.
人によって意見が分かれるところですが、これがその説明です。
And imagine if the AI then goes and says, oh my God, I've been feeling the same thing, but I didn't know how to articulate it.
もし、そのAIが「私も同じようなことを感じていたのですが、どう表現したらいいのかわかりませんでした」と言ったらどうでしょう。
That would be okay.
それはそれでいいんじゃないでしょうか。
That would be definitely something to think about.
それは間違いなく、考えるべきことでしょう。
It's like if the AI was just trained on very mundane data around objects and going from place to place or maybe, you know, something like this from a very narrow set of concepts, we would never ever mention that.
もしAIが、物体や場所から場所への移動など、ごくありふれたデータについて訓練されただけだとしたら、そのようなことは決して口にしないでしょう。
And yet if it could somehow eloquent and correctly talk about it in a way that we would recognize, that would be convincing.
しかし、もしAIが、私たちが認識できるような方法で、雄弁に、そして正しくそれを語ることができたなら、それは説得力のあることでしょう。
And do you think of it as consciousness as something of degree or is it something more binary?
また、意識というのは、程度のものだと考えているのでしょうか、それとももっと二元的なものだと考えているのでしょうか。
I think it's something that's more a matter of degree.
どちらかというと、程度の問題だと思います。
I think that, I think that like, you know, let's say if a person is very tired, extremely tired and maybe drunk, then perhaps if that's, when someone is in that state and maybe their consciousness is already reduced to some degree.
例えば、人がとても疲れていて、ものすごく疲れていて、酔っ払っているような場合、そのような状態になると、おそらく意識はすでにある程度低下しているのではないかと思います。
I can imagine that animals have a more reduced form of consciousness.
動物の場合は、もっと意識が低下していると想像できます。
If you imagine going from, you know, large primates, maybe dogs, cats, and then eventually you get mice, you might get an insect.
大型の霊長類から、犬や猫になり、やがてネズミになり、昆虫になると想像してみてください。
Feels like, I would say it's pretty continuous, yeah.
そう考えると、かなり連続的なものだと言えますね。
Okay.
そうですね。
I wanna move on even though I could, I would love to keep asking you more questions along the lines of the technology, but I wanna move on to talking about the mission of OpenAI and how you perceive any issues around ethics and your role as chief science officer, how ethics informs, if at all, how you think about your role.
技術に関する質問をもっと続けたいですが、OpenAIのミッションや倫理問題について話を進めたいと思います。チーフ・サイエンス・オフィサーとして、倫理がどのようにあなたの役割に影響を与えるか教えてください。
And so let me just lay a couple of foundation points out and then have you speak.
そこで、いくつかの基本的なポイントを説明し、その後、あなたに話してもらいたいと思います。
As you know, OpenAI's mission is to ensure that artificial general intelligence benefits all of humanity.
ご存知のように、OpenAIのミッションは、人工知能が全人類に利益をもたらすようにすることです。
And it started off as a nonprofit and open-sourced, and it is now a for-profit and closed-sourced and with a close relationship with Microsoft.
最初は非営利でオープンソースでしたが、現在は営利でクローズドソースとなり、マイクロソフトと密接な関係にあります。
And Elon Musk, who I believe recruited you to originally join OpenAI and gave a hundred million dollars when it was a nonprofit, has says that the original vision was to create a counterweight to Google and the corporate world.
イーロン・マスクは、OpenAIが非営利団体だったころに、あなたを勧誘して1億ドルを提供したと思いますが、当初のビジョンは、Googleや企業への対抗馬を作ることだったと語っています。
And he didn't wanna have a world in which AI, which he perceives in others can have an existential threat to humanity to be solely in the holds of a corporate, of a for-profit.
そして、AIが人類にとって存亡の危機となりうることを認識し、それを企業や営利団体の手に委ねるような世界にはしたくなかったのです。
And now, OpenAI is neither open nor exclusively a nonprofit.
そして今、OpenAIはオープンでもなく、非営利団体でもない。
It's also a for-profit with close ties to Microsoft.
マイクロソフトと密接な関係を持つ営利企業でもあるのです。
And it looks like the world may be headed towards a private duopoly between Microsoft and Google.
そして、世界はマイクロソフトとグーグルの私的独占に向かいつつあるように見えます。
Can you shed light on the calculus to shift from a for-profit to a nonprofit?
営利から非営利へ移行するための算段を明らかにしていただけますか?
And did you weigh in the ethics of that decision?
また、その決断の倫理を考慮したのでしょうか?
And do ethics play a role in how you conceive of your role as the chief science officer?
また、チーフ・サイエンス・オフィサーとしての役割をどのように考えているか、倫理観は関係していますか?
Or do you view it more as something that somebody else should handle and you are mainly just tasked with pushing the technology forward?
それとも、他の誰かが処理すべきことで、あなたは主に技術を前進させることを任務としていると考えているのでしょうか?
Yeah, so this question has many parts.
そうですね、この質問にはいろいろな部分があります。
Let me think about the best way to approach it.
どのようにアプローチするのがベストなのか、考えてみたいと思います。
So there are several parts.
いくつかのパートがあります。
There is the question around open source versus closed source.
オープンソースとクローズドソースの問題。
There is a question around nonprofit versus for-profit and the connection with Microsoft.
非営利と営利の問題、そしてマイクロソフトとの関係もあります。
And how to see that in the context of Elon Musk's recent comments.
そして、イーロン・マスクの最近の発言との関連で、それをどう見るか。
And then the question about how I see my role in this.
そして、この中で自分の役割をどう考えるかという問題です。
Maybe I'll start with that because I think that's easier.
その方が簡単だと思うので、そちらから始めようと思います。
Okay, so I feel, the way I see my role, I feel a lot, I feel direct responsibility for what OpenAI does.
自分の役割についてですが、OpenAIの活動には直接的な責任を感じています。
Even though my role is primarily around advancing the science, it is still the case, I'm one of the founders of the company.
私の役割は主に科学の進歩ですが、それでも、私は会社の創設者の一人です。
And ultimately, I care a lot about OpenAI's overall impact.
そして最終的には、OpenAIの全体的な影響力をとても気にしています。
Now, I want to go, so with this context, I want to go and talk about the open source versus closed source and the nonprofit versus for-profit.
さて、このような背景から、オープンソースとクローズドソース、非営利と営利の違いについてお話したいと思います。
And I want to start with open source versus closed source because I think that the challenge with AI is that AI is so all encompassing and it comes with many different challenges.
オープンソースとクローズドソースの比較から始めたいのですが、AIの課題は、AIが非常に包括的で、さまざまな課題を伴うことだと思います。
It comes with many different dangers which come into conflict with each other.
また、AIにはさまざまな危険性があり、互いに衝突することもあります。
And I think the open source versus closed source is a great example of that.
オープンソースとクローズドソースの比較は、その好例だと思います。
Why is it desirable, let me put it this way.
なぜそれが望ましいのか、こう言ってみましょう。
What are some reasons for which it is desirable to open source AI?
AIをオープンソース化することが望ましい理由は何でしょうか?
The answer there would be to prevent concentration of power in the hands of those who are building the AI.
そこでの答えは、AIを構築している人たちの手に権力が集中するのを防ぐためでしょう。
So if you are in a world where, let's say, there is only a small number of companies you might have that control this very powerful technology.
つまり、例えば、この非常に強力なテクノロジーをコントロールする企業が、ごく少数しか存在しないような世界であれば、あなたはこう言うかもしれません。
You might say, this is an undesirable world and that AI should be open and that anyone could use the AI.
これは望ましくない世界であり、AIはオープンであるべきで、誰でもAIを使うことができる、と言うかもしれません。
This is the argument for open source.
これがオープンソースの主張です。
But this argument, of course, to state the obvious, there are near term commercial incentives against open source.
しかし、この議論は、もちろん、明白なことを言えば、オープンソースに対する短期的な商業的インセンティブがあります。
But there is another longer term argument against open sourcing as well, which is if we believe, if one believes that eventually AI is going to be unbelievably powerful, if we get to a point where your AI is so powerful, where you can just tell it, hey, can you autonomously create a biological, like, I don't know, a biological research lab autonomously?
しかし、オープンソース化に対するもう一つの長期的な反論もあります。それは、AIが最終的に信じられないほど強力になると信じるなら、AIがあなたに言わせれば、「ねえ、自律的に生物学的な研究所を作ってくれるか?」と言えるほど強力になるとしたら、どうでしょうか?
Do all the paperwork, run the space, hire the technicians, aggregate the experiments, do all this autonomously.
すべての事務手続き、スペースの運営、技術者の雇用、実験の集計、これらすべてを自律的に行うことができます。
Like, that starts to get mind-bendingly powerful.
そうなると、気が遠くなるようなパワーを発揮し始めます。
Should this be open sourced also?
これもオープンソースにすべきなのでしょうか?
So my position on the open source question is that I think that there is maybe a level of capability.
オープンソースの問題に対する私の立場は、おそらく能力のレベルがあると思うのです。
You can think about these neural networks in terms of capability.
ニューラルネットワークを能力という観点で考えてみるとよいでしょう。
How capable they are, how smart they are, how much can they do?
どれくらいの能力があるのか、どれくらいの賢さがあるのか、どれくらいのことができるのか。
When the capability is on the lower end, I think open sourcing is a great thing.
能力が低いうちは、オープンソースは素晴らしいことだと思います。
But at some point, and you know, there can be debate about where the point is, but I would say that at some point, the capability will become so vast that it will be obviously irresponsible to open source models.
しかし、ある時点で、どこがポイントかについては議論がありますが、ある時点で、能力があまりにも膨大になり、オープンソースモデルを使うことが明らかに無責任になると言えるでしょう。
And was that the driver behind closed source work and was that the driver behind closed sourcing it, or was it driven by a devil's compact or business necessity to get cash in from Microsoft or others to support the viability of the business?
そして、それがクローズドソース化の原動力となったのでしょうか、それとも、マイクロソフトなどから現金をもらって事業の存続を支えるという、悪魔のコンパクトさやビジネス上の必要性によって原動力となったのでしょうか。
Was the decision-making to close it down actually driven by that line of reasoning, or was it driven by more?
それとも、マイクロソフトなどから資金を得るためのビジネス上の必要性だったのでしょうか?
So the way I'd articulate it, my view is that the current level of capability is still not that high where it will be the safety consideration that will drive the closed source in the model, this kind of research.
つまり、私の考えでは、現在の能力レベルはまだそれほど高くはなく、モデルやこの種の研究でクローズドソースを推進するのは、安全性を考慮したものであると考えます。
So in other words, I claim that it goes in phases.
つまり、段階的に進めていくというのが私の主張です。
Right now, it is indeed the competitive phase, but I claim that as the capabilities of these models keep increasing, there will come a day where it will be the safety consideration that will be the obvious and immediate driver to not open source these models.
今はまだ競争的な段階ですが、このようなモデルの能力が向上していくにつれて、安全への配慮がオープンソース化しないための明白かつ直接的な原動力となる日が来るだろうと私は主張しています。
So this is the open source versus closed source, but your question had another part which is non-profit versus for-profit.
これはオープンソースとクローズドソースの違いですが、ご質問にはもう一つ、非営利と営利の違いもありました。
And we can talk about that also.
それについてもお話ししましょう。
You know, indeed, it would be preferable in a certain meaningful sense if OpenAI could just be a non-profit from now until the mission of OpenAI is complete.
確かに、OpenAIのミッションが完了するまで、OpenAIが非営利であることは、ある意味望ましいことです。
However, one of the things that's worth pointing out is the very significant cost of these data centers.
しかし、指摘すべきは、これらのデータセンターのコストが非常に大きいということです。
I'm sure you're reading about various AI startups and the amount of money they are raising, the great majority of which goes to the cloud providers.
皆さんは様々なAIスタートアップの記事を読んでいると思いますが、彼らが集めている資金の大部分はクラウドプロバイダーへ流れています。
Why is that?
なぜでしょうか?
Well, the reason so much money is needed is because this is the nature of these large neural networks.
なぜこれほど多くの資金が必要かというと、これが大規模なニューラルネットワークの性質だからです。
They need the compute, end of story.
つまり、計算機が必要なのです。
You can see something like this.
このような図をご覧ください。
You can see a divide that's now happening between academia and the AI companies.
アカデミアとAI企業の間に今起きている分裂を見ることができます。
So for a long time, for many decades, cutting edge research in AI took place in academic departments, in universities.
長い間、何十年もの間、AIの最先端研究は大学の学部で行われていました。
That kept being the case up until the mid 2010s.
2010年代半ばまではそうでした。
But at some point, when the complexity and the cost of these projects started to get very large, it no longer remained possible for universities to be competitive.
しかし、ある時点で、これらのプロジェクトの複雑さとコストが非常に大きくなり、大学ではもはや競争力を維持できなくなりました。
And now universities need, university research in AI needs to find some other way in which to contribute.
そして今、大学は、AIにおける大学研究が貢献できる他の方法を見つける必要があります。
Those ways exist.
そのような方法は存在します。
They're just different from the way they're used to and different from the way the companies are contributing right now.
ただ、今までの方法とは違いますし、今現在の企業の貢献の仕方とも違います。
Now, with this context, you're saying, okay, the thing about non-profit, a non-profit is that people who give money to a non-profit never get to see any of it back.
さて、このような背景から、あなたは、非営利団体について、非営利団体に資金を提供した人は、その資金が戻ってくることはない、と言っているのです。
It is a real donation.
それは本当の寄付です。
And believe it or not, it is quite a bit harder to convince people to give money to a non-profit.
そして、信じられないかもしれませんが、非営利団体にお金を寄付するよう人々を説得するのはかなり難しいことなのです。
And so we think, what's the solution there?
そこで、私たちは、どうすればいいのか?
Or what is a good course of action?
どうすればいいのだろう?
And so we think, what's the solution there?
そこで、私たちは考えます。
Or what is a good course of action?
あるいは、良い行動指針は何だろう?
So we came up with an idea that to my knowledge is unique in all corporate structures in the world.
そこで私たちは、私の知る限り、世界中のあらゆる企業構造の中で唯一無二のアイデアを思いつきました。
The OpenAI corporate structure is absolutely unique.
OpenAIの企業構造は、まったくユニークなものです。
OpenAI is not a for-profit company.
OpenAIは営利を目的とした会社ではありません。
It is a capped profit company.
利益の上限を定めた会社です。
And I'd like to explain what that means.
その意味を説明したいと思います。
What that means is that equity in OpenAI can be better seen as a bond rather than equity in a normal company.
つまり、OpenAIの株式は、普通の会社の株式というよりも、債券と考えたほうがいいということです。
The main feature of a bond is that once it's paid out, it's gone.
債券の最大の特徴は、一度支払われたらなくなってしまうということです。
So in other words, OpenAI has a finite obligation to its investors as opposed to an infinite obligation to that normal companies have.
つまり、OpenAIの投資家に対する義務は有限であり、普通の会社が持つ義務は無限であるということです。
And does that include the founders?
その中には創業者も含まれるのでしょうか?
Do the founders have equity in OpenAI?
創業者はOpenAIの株式を持っているのでしょうか?
So some Altman does not have equity, but the other founders do.
アルトマンの何人かは持分を持っていませんが、他の創業者は持っています。
And is it capped or is it unlimited?
それは上限があるのですか、それとも無制限なのですか?
It is capped.
上限があります。
And how does that cap?
その上限はどうなっているのでしょうか?
Is that capped?
上限があるのですか?
Because the founders, I presume, didn't buy in unless it's capped at the nominal share value.
創業者たちは、名目上の株式価値に上限がない限り、購入しなかったと推測されるからです。
I'm not sure I understand the question precisely, but what I can say, I can answer the part which I do understand, which is like there is certainly, like it is different from normal startup equity, but there are some similarities as well, where the earlier you join the company, the higher the cap is.
質問の意味が正確には分かりませんが、理解できる部分に答えます。それは通常のスタートアップ株式とは異なりますが、会社に早く参加するほど、キャップが高くなるという点では類似点もあります。
Because then the larger cap is needed to attract the initial investors.
なぜなら、初期投資家を惹きつけるためには、より大きなキャップが必要だからです。
As the company continues to succeed, the cap decreases.
会社が成功し続けるにつれて、キャップは減少していきます。
And why is that important?
なぜそれが重要なのでしょうか?
It's important because it means that the company, once all the obligation to investors and employees are paid out, OpenAI becomes a non-profit again.
それは、投資家と従業員に対するすべての義務が支払われると、OpenAIは再び非営利団体になることを意味するからです。
And you can say, this is totally crazy, what are you talking about?
こんなのおかしい、何を言っているんだ、と言われるかもしれません。
Like it's not gonna change anything.
何も変わらないじゃないか、と言われるかもしれません。
But it's worth considering what we expect.
しかし、私たちが何を期待するかを考える価値はあります。
Like it's worth looking at what we think AI will be I mean, we can look at what AI is today.
つまり、現在のAIがどのようなものかを見てみる価値はあります。
And I think it is not at all inconceivable for OpenAI to achieve its, to pay out its obligation to the investors and employees, become a non-profit at around the time when perhaps the computers will become so capable, where the economic destruction will be very big, where this transition will be very beneficial.
OpenAIが投資家や従業員に対する義務を果たし、非営利団体になるころには、コンピュータが非常に優れた能力を持ち、経済的な破壊が非常に大きく、この移行が非常に有益になるとは全く考えられません。
So this is the answer on the capitalization of cap profit versus non-profit.
これが、キャピタルプロフィット対ノンプロフィットの資本提携に関する答えです。
There was a last part to your question.
質問の最後の部分がありました。
I know I'm speaking for a while, but the question had many parts.
私はしばらく話していますが、質問には多くの部分がありました。
The last part of your question is the Microsoft relationship.
ご質問の最後の部分は、マイクロソフトとの関係です。
And so here, the thing that's very fortunate is that Microsoft is a, they're thinking about these questions the right way.
ここで、非常に幸運なことに、マイクロソフトはこのような質問に対して、正しい方法で考えてくれています。
They understand the potential and the gravity of AGI.
彼らはAGIの可能性と重大性を理解しています。
And so for example, on the, on all the investor documents that any investor in OpenAI has signed, and by the way, Microsoft is an investor into OpenAI, which is a very different relationship from the Google DeepMind.
たとえば、OpenAI の投資家が署名したすべての投資家向け文書について、ところで Microsoft は OpenAI への投資家であり、Google DeepMind とは非常に異なる関係にあります。
Anyone who signed any document, any investment document, there is a purple rectangle at the top of the investment document, which says that the fiduciary duty of OpenAI is to the OpenAI mission, which means that you run the risk of potentially losing all your money if the mission comes in conflict.
どんな投資書類に署名した人でも、投資書類の上部に紫の四角があり、OpenAIの忠実義務はOpenAIのミッションにあると書かれています。これは、ミッションが競合すると、お金をすべて失う可能性があるというリスクを負っています。
So this is something that all the investors have signed.
これは投資家全員が署名したものです。
And let me just make this clear for everybody, because Google acquired DeepMind.
そして、皆のためにはっきりさせておきたいのですが、GoogleはDeepMindを買収しました。
So DeepMind was just an asset inside of Google, but beholden to Google, you're making the distinction that with OpenAI, Microsoft is an investor, and so beholden to this fiduciary duty for the mission of OpenAI, which is held by the nonprofit, which is a GP or an LP in the for-profit.
DeepMindはGoogleの中の資産であり、Googleに従属していましたが、OpenAIとMicrosoftの関係では、Microsoftは投資家であり、OpenAIのミッションに対する忠実義務に従属しています。そのミッションは非営利団体によって保持されており、営利法人のGPまたはLPです。
Okay, understood.
なるほど、わかりました。
Yeah, something like this.
ええ、こんな感じです。
You know, I am, you know, there are people, I can't tell you the precise details, but this is the general picture.
私は、正確なことは言えませんが、このような一般的なイメージを持っている人がいます。
And, you know, some have claimed though now, especially Steve Wozniak, the co-founder of Apple, and Elon Musk have famously signed this very public petition saying that the point of no return is already passed or we're approaching it where it's gonna be impossible to rein in AI and its repercussions if we don't halt it now.
アップルの共同創設者であるスティーブ・ウォズニアックやイーロン・マスクは、もう引き返せない地点を過ぎているか、そこに近づいており、AIとその影響を今止めなければ制御不可能になるという公開請願に署名しています。
And they've called for halting AI.
そして、彼らはAIを止めようと呼びかけています。
I'm curious on, you are a world citizen, Ilya.
イリヤさんは世界市民なんですよね。
You were born in Russia, you were raised in Israel, you're Canadian, and it's OpenAI's response to that public petition was, I know Sam basically said that, you know, this wasn't the right way to go about doing that, but also in parallel, Sam is on a world tour with many countries that also can be antagonistic towards the West.
あなたはロシアで生まれ、イスラエルで育ち、カナダ人です。OpenAIの公開請願に対する回答は、サムがそうではない方法で進めるべきだと言っていましたが、同時にサムは西側に敵対的な国々も含む世界ツアーをしています。
Are there any citizen obligations, ethical obligations that you think also overweigh your technological obligations when it comes to spreading the technology around the world right now through OpenAI?
現在、OpenAI を通じて世界中にテクノロジーを広めることに関して、市民としての義務、倫理的義務が技術的義務よりも重要だと思われるものはありますか?
Do you think that should be beholden to a regulation or some oversight?
規制や監督に従うべきと思いますか?
Let me think.
考えさせてください。
Once again, the question had a number of parts.
もう一度言いますが、この質問にはいくつもの部分がありました。
It did, I apologize.
そうですね、申し訳ありません。
I'm trying to give you the, so you can respond however you want to on that.
その点については、どのようにでもお答えいただけるよう、お伝えしています。
I know we're gonna come out off of time, so I just wanna give you the mic and just share everything that's on my mind and you can decide how you wanna handle it.
時間切れになりそうなので、マイクを渡して、私の心にあることをすべて話して、どう対処するかはあなたが決めてください。
Yeah, thank you.
ええ、ありがとうございます。
I mean, you know, it is true that AI is going to become truly extremely powerful and truly extremely transformative.
AIが非常に強力になり、非常に大きな変革をもたらすことは事実です。
And I do think that we will want to move to a world with sensible government regulations.
そして、賢明な政府の規制がある世界に移行したいと思うようになると思います。
And there are several dimensions to it.
そして、それにはいくつかの次元があります。
We want to be in a world where there are clear rules about, for example, training more powerful neural networks.
例えば、より強力なニューラルネットワークを訓練することについて、明確なルールがある世界でありたいと思います。
We want there to be some kind of careful evaluation, careful prediction of these, of what we expect these neural networks, of what they can do today and of what we expect them to be able to do, let's say in a year from now or by the time they face it.
私たちは、これらのニューラルネットワークが現在できることや、1年後や直面する時にできると予想されることについて、慎重な評価、慎重な予測があることを望んでいます。
In a year from now or by the time they finish training.
今から1年後、あるいは彼らがトレーニングを終えるまでに。
I think all these things will be very necessary in order to like rationally, I wouldn't use the word slow down the progress.
これらのことは、合理的に、進歩を遅らせるという言葉は使いませんが、非常に必要なことだと考えています。
I would use the term, you want to make it so that the progress is sensible so that with each step we've done the homework.
つまり、一歩一歩、宿題をこなしていくような、理にかなった進歩を遂げたいのです。
And indeed we can make a credible story that, okay, the neural network, the system that we've trained, it has, we are doing this and here are all the steps and it's been verified or certified.
実際、ニューラル ネットワーク、私たちが訓練したシステム、それを実行していて、すべての手順が検証または認定されているという、信頼できる話をすることができます。
I think that is the world that we are headed to, which I think is correct.
それが、私たちが向かっている世界であり、正しいと思っています。
And as for the citizen obligation, I feel like, I mean, I'll answer it like this.
そして、市民の義務については、私はこう答えることにしています。
Like I think like there are two answers to it.
2つの答えがあるような気がするんです。
So obviously, you know, I live in the United States and I really like it here and I want this place to flourish as much as possible, care about that.
私はアメリカに住んでいて、ここが本当に好きで、この場所ができるだけ栄えてほしいと思っています。
I think that of course there will be lots of, but the world is much more than just the U.S. And I think that these are the kind of questions which I feel a little bit, let's say outside of my expertise, how these between country relationships work out.
もちろんたくさんあると思いますが、世界はアメリカだけではありません。そして、国家間の関係がどのように機能するかというような質問は、少し私の専門外だと感じます。
But I'm sure there will be lots of discussions there as well.
しかし、そこでも多くの議論が交わされることでしょう。
Okay, Ilya, can I turn a little bit towards strategy?
では、イリヤ、少し戦略的な話に移ってもいいですか?
I'm curious for you guys internally, what metrics do you track as your North Star?
社内の皆さんにお聞きしたいのですが、北極星としてどのような指標を追っているのでしょうか?
What are the most sacred KPIs that you use to measure OpenAI's success right now?
OpenAIの成功を測るために、最も神聖なKPIは何でしょうか?
The most sacred KPIs.
最も神聖なKPIですね。
You know, I think this is also the kind of question where maybe different people will give you different answers.
この質問も、人によって答えが違うかもしれませんね。
But I would say that there are incredibly many but if I were to really narrow it down, I would say that there are a couple of really important KPI, of really important dimensions of progress.
しかし、私は、非常に多くのKPIがあると思いますが、もし本当に絞り込むとしたら、本当に重要なKPI、本当に重要な進歩の次元が2つあると言えるでしょう。
One is undeniably the technical progress.
ひとつは、紛れもなく技術的な進歩です。
Are we doing good research?
私たちは良い研究をしているのでしょうか?
Do we understand our systems better?
システムをよりよく理解しているか?
Are we able to train them better?
システムをよりよく訓練できているか?
Can we control them better?
システムをよりよく制御できているか?
Is our research plan being executed well?
研究計画はうまく実行されているか?
Is our safety plan being executed well?
私たちの安全計画はうまく実行されているか?
How happy are we with it?
私たちはそれにどれだけ満足しているか?
I would say this would be my description of the primary KPI, which is do a good job of the technology.
これが私の言う第一のKPI、つまり「技術をうまく使う」ということです。
Then there is, of course, stuff around the product, but which I think is cool.
それから、もちろん、製品にまつわることもありますが、これはクールだと思います。
But I would say that it is really the core technology, which is the heart of OpenAI.
しかし、私が言いたいのは、OpenAIの心臓部であるコアテクノロジーです。
The technology, its development and its control, its steering.
テクノロジー、その開発、そしてコントロール、ステアリングです。
And do you view, right now, chat GPT is a destination.
今、チャットGPTは目的地だと考えているのでしょうか。
Do you view OpenAI in the future being a destination that people go to, like Google, or will it be powering other applications and be the backend or be used as part of the backend infrastructure?
将来的にOpenAIは、Googleのように人々が行く目的地となるのでしょうか。それとも、他のアプリケーションに力を与え、バックエンドやバックエンドインフラの一部として使われるようになるのでしょうか。
Is it a destination or is it going to be more behind the scenes in 5 to 10 years?
それとも、5年後、10年後には、もっと舞台裏にいるのでしょうか?
Yeah, well, I mean, things change so fast.
ええ、まあ、つまり、物事の変化はとても速いのです。
I cannot make any claims about 5 to 10 years in terms of the correct shape of the product.
5年後、10年後というのは、正しい形という意味では何ら主張することはできません。
I imagine a little bit of both, perhaps.
おそらく、その両方を少しずつ想像しています。
But this kind of question, I mean, I think it remains to be seen.
でも、この種の質問は、つまり、まだわからないと思うんです。
I think this stuff is still so new.
この手のものは、まだ新しいと思うので。
OK, I'm going to ask one more question.
では、もう1つ質問させていただきます。
I'm going to jump to the student questions.
学生の質問に飛びますね。
If you were a student at Stanford today interested in AI, if you were somebody who wants to be Ilya, what would you focus your time?
もしあなたがAIに興味のあるスタンフォード大学の学生だとしたら、イリヤになりたいと思っている人だとしたら、何に時間を割くでしょうか?
And another second question on this, if you're also interested in entrepreneurship, what advice would you give for a Stanford undergrad engineer that's interested in AI and entrepreneurship?
また、2つ目の質問として、もしあなたが起業にも興味があるのなら、AIと起業に興味があるスタンフォード大学の学部生エンジニアにどんなアドバイスをするでしょうか?
So I think on the first one, it's always hard to give generic advice like this.
最初の質問では、このような一般的なアドバイスをするのはいつも難しいと思います。
But I can still provide some generic advice nonetheless.
しかし、それでも一般的なアドバイスをすることは可能です。
And I think it's something like it is generally a good idea to lean into one's unique predispositions.
それは、一般的に、自分のユニークな素養に寄り添うことが良いということです。
If you think about the set of, let's say, inclinations or skills or talents that the person might have, the combination is pretty rare.
例えば、その人が持っているかもしれない傾向やスキル、才能のセットについて考えてみると、その組み合わせはかなり稀です。
So leaning into that is a very good idea, no matter which direction you choose to go in.
ですから、どのような方向に進むにしても、それを活用することは非常に良いアイデアです。
And then on the AI research, I would say that there, I could say something.
そして、AIの研究ですが、これは私が何か言うことができるかもしれません。
But there especially, you want to lean into your own ideas and really ask yourself, is there something that's totally obvious to you that makes you go, why is everyone else not getting it?
しかし、特にこの分野では、自分のアイデアに寄り添い、「なぜ他の人はそれを理解できないのだろう」と思わせるような、自分にとってまったく当たり前のことはないだろうかと自問自答することをお勧めします。
If you feel like this, that's a good sign.
もしそう感じたら、それは良い兆候です。
It means that you might be able, that you want to lean into that and explore it and see if your instinct is true or not.
その直感が正しいかどうかを確かめるために、その直感に寄り添い、探求したいと思うかもしれないということです。
It may not be true.
そうでない場合もあります。
But you know, my advisor, Jeff Hinton, says this thing, which I really like.
でも、私のアドバイザーであるジェフ・ヒントンは、こんなことを言っています。
He says, you should trust your intuition.
自分の直感を信じなさいということです。
Because if your intuition is good, you go really far.
なぜなら、直感が優れていれば、本当に遠くまで行けるからです。
And if it's not good, then there's nothing you can do.
もし直感が良くなければ、どうしようもない。
And as far as entrepreneurship is concerned, I feel like this is a place where the unique perspective is even more valuable.
そして、起業に関しては、ユニークな視点がさらに価値を持つ場であるような気がします。
Or maybe equally, it's because it's maybe, maybe I'll explain why I think it's more valuable than in research.
あるいは、同じように、研究よりも価値があると思う理由を、もしかしたら、説明するかもしれないからです。
Well, in research, it's very valuable too.
まあ、研究においては、それも非常に価値があります。
But in entrepreneurship, you need to almost pull from your unique life experience, where you say, OK, I see this thing.
しかし、起業の場合は、自分のユニークな人生経験から引き出さなければなりません。
I see this technology.
このテクノロジーはどうだろう?
I see something, like take a very, very broad view and see if you can hone in on something.
というように、非常に広い視野を持ち、何かに焦点を当てられるかどうかを確認するのです。
And then actually, you just go for it.
そして、実際にやってみるのです。
So that would be the conclusion of my generic advice.
それが、私の一般的なアドバイスの結論です。
OK, which is great.
なるほど、それは素晴らしいですね。
That's also great.
これも素晴らしいことです。
I'm going to move on to the student question.
では、学生の質問に移りたいと思います。
So one of the most upvoted question is, how do you see the field of deep learning evolving in the next five to 10 years?
ディープラーニングの分野は、今後5年から10年の間にどのように進化すると思われますか?
Yeah, let's see.
ええ、そうですね。
You know, I expect deep learning to continue to make progress.
ディープラーニングはこれからも進歩し続けると思っていますね。
I expect that there was a period of time where a lot of progress came from scaling.
一時期、スケーリングによって多くの進歩がもたらされた時期があったと思います。
And we saw that in the most pronounced way in going from GPT-1 to GPT-3.
GPT-1からGPT-3への移行で、それが最も顕著に表れました。
But things will change a little bit.
しかし、状況は少しずつ変化していくでしょう。
The reason that the reason that progress in scaling was so rapid is because people had all these data centers, which they weren't using for a single training run.
スケーリングの進歩が早かったのは、人々が多くのデータセンターを持っていて、それを1回のトレーニング実行に使っていなかったからです。
So by simply reallocating existing resources, you could make a lot of progress.
ですから、既存のリソースを再配分するだけで、多くの進歩を遂げることができたのです。
And it doesn't take that long, necessarily, to reallocate existing resources.
しかも、既存のリソースを再配置するのにそれほど時間はかかりません。
You just need to, someone just needs to decide to do so.
ただ、誰かがそうすることを決めるだけでいいのです。
It is different now, because the training runs are very big.
しかし、今回はトレーニングの規模が非常に大きいので、その点では異なります。
And the scaling is not going to be progressing as fast as it used to be, because building data centers takes time.
データセンターの建設には時間がかかるので、スケーリングは以前ほど速く進まないでしょう。
But at the same time, I expect deep learning to continue to make progress from other places.
しかし同時に、ディープラーニングは他の場所からも進歩し続けるだろうと予想しています。
The deep learning stack is quite deep.
ディープラーニングのスタックはかなり奥が深いです。
And I expect that there will be improvements in many layers of the stack.
そして、スタックの多くの層で改良が進むと思います。
And together, they will still lead to progress being very robust.
そして、それらを合わせると、やはり進歩は非常に強固なものになるはずです。
And so if I had to guess, I'd imagine that there would be maybe, I'm certain we will discover new properties which are currently unknown of deep learning.
そして、もし私が推測するならば、もしかしたら、現在ディープラーニングの未知である新しい特性を発見することができるかもしれませんね。
And those properties will be utilized.
そして、その特性は活用されるでしょう。
And I fully expect that the systems of 5 to 10 years from now will be much, much better than the ones that we have right now.
そして、5年後、10年後のシステムは、今のシステムよりもずっとずっと良くなっているだろうと、私は十分に期待しています。
But exactly how it's going to look like, I think it's a bit harder to answer.
しかし、それが具体的にどのようなものになるかというと、ちょっと答えづらいと思います。
It's a bit like it's because the improvements, there will be maybe a small number of big improvements and also a large number of small improvements, all integrated into a large complex engineering artifact.
それは少し似ていますが、それは改善のためです。おそらく、少数の大きな改善と多数の小さな改善があり、すべてが大規模で複雑なエンジニアリング成果物に統合されます。
And can I ask, your co-founder Sam Altman has said that we've reached the limits of what we can achieve by scaling to larger language models.
共同創業者のサム・アルトマンは、より大きな言語モデルに拡張することで達成できることの限界に達したと述べていますね。
Do you agree?
そうお考えですか?
And if so, then what is the next innovation frontier that you're focusing on, if that's the case?
もしそうだとしたら、あなたが注目している次のイノベーションのフロンティアは何でしょうか?
Yeah, so I think maybe, I don't know exactly what he said, but maybe he meant something like that the age of easy scaling has ended or something like this.
ええ、ですから、たぶん、彼が何を言ったのか正確には分かりませんが、簡単にスケーリングできる時代は終わったとか、そういう意味だと思います。
Like, of course, the larger neural nets will be better, but it will be a lot of effort and cost to do them.
もちろん、より大きなニューラルネットはより良いものになるでしょうが、それを実現するためには多くの労力とコストがかかるでしょう。
But I think there will be lots of different frontiers.
でも、いろいろなフロンティアは出てくると思います。
And actually, to the question of how can one contribute in deep learning, identifying such a frontier, perhaps one that's been missed by others, is very fruitful.
ディープラーニングでどのように貢献できるかという問いに対して、そのようなフロンティア、おそらく他の人が見逃しているフロンティアを特定することは、非常に実りあることです。
And is it, can I go even just deeper on that, because I think there is this debate about vertical focus versus generalist training.
垂直方向への集中とジェネラリスト・トレーニングの違いについて、議論があると思います。
Is it better, do you think there's better performance that can be achieved in particular domains such as law or medicine by training with special data sets, or is it likely that generalist training with all available data will be more beneficial?
法律や医療といった特定の領域で、特別なデータセットを使ってトレーニングすることでより良いパフォーマンスが得られるのか、それとも、利用可能なすべてのデータを使った一般的なトレーニングの方がより有益なのか。
So at some point, we should absolutely expect specialist training to make a huge impact.
ですから、ある時点では、専門家のトレーニングが大きな影響を与えることを絶対に期待すべきです。
But the reason we do the generalist training is just so that we can even reach the point where, just so that we can reach the point where the neural network can even understand the questions that we are asking.
しかし、ジェネラリスト・トレーニングを行うのは、ニューラルネットワークが私たちの問いかけを理解できるところまで到達できるようにするためです。
And only when it has a very robust understanding, only then we can go into specialist training and really benefit from it.
そして、非常に強固な理解を得たときに初めて、専門的なトレーニングに進み、その恩恵を受けることができるのです。
So yeah, I mean, I think all these, I think these are all fruitful directions.
つまり、これらはすべて、実りある方向性だと思うのです。
But you don't think, when do you think we'll be at that point when specialist training is the thing to focus on?
しかし、専門的なトレーニングに力を入れるようになるのは、いつになるのでしょうか?
I mean, if you look at people who do open source work, people who work with open source models, they do a fair bit of this kind of specialist training because they have a fairly underpowered model.
オープンソースの仕事をしている人たちを見ていると、オープンソースのモデルを使って仕事をしている人たちは、かなり力不足なモデルを使っているため、このような専門的なトレーニングをかなり行っているようです。
And they try to get any ounce of performance they can out of it.
そして、そのモデルから少しでも性能を引き出そうとします。
So I would say that this is an example.
ですから、これはその一例と言えるでしょう。
I'd say that this is an example of it happening.
これはその一例と言えるでしょう。
It's already happening to some degree.
すでにある程度は起きていることなのです。
It's not a binary.
二元論ではありません。
You might want to think of it as a continuum, a spectrum.
連続体、スペクトルとして考えたほうがいいかもしれません。
But do you think that the winning advantage is going to be having these proprietary data sets?
しかし、勝ち組になるには、こうした独自のデータセットを持つことだと思いますか?
Or is it going to be having a much higher performance, large language model when it comes to these applications of AI into verticals?
それとも、AIを垂直方向に応用する場合、より高性能で大規模な言語モデルを持つことでしょうか?
So I think it's maybe productive to think about an AI like this as a combination of multiple factors, where each factor makes a contribution.
ですから、このようなAIは、複数の要素の組み合わせであり、それぞれの要素が貢献すると考えるのが生産的だと思います。
And is it better to have special data which helps you make your AI better in a particular set of tasks?
また、特定のタスクのセットでAIをより良くするのに役立つ特別なデータがあった方が良いのでしょうか?
Of course.
もちろんです。
Is it better to have a more capable base model?
より高性能なベースモデルがあったほうがいいのでしょうか?
Of course, from the perspective of the task.
もちろん、タスクの観点からはそうです。
So maybe this is the answer.
だから、これが答えなのかもしれません。
It's not an either or.
どちらか一方というわけではないのです。
I'm going to move down to the other questions.
他の質問に移ろうと思います。
There's a question on what was the cost of training and developing GPT-3 slash 4?
GPT-3スラッシュ4の訓練と開発にかかった費用はどうだったのか、という質問がある。
Yeah.
そうですね。
So for obvious reasons, I can't comment on that.
だから明らかな理由で、私はそれについてコメントできない。
OK.
そうですか。
But I think even from our research community, there's a strong desire to be able to get access to different aspects of OpenAI's technology.
しかし、私たちの研究コミュニティからも、OpenAIの技術のさまざまな側面にアクセスできるようになりたいという強い要望があると思います。
And are there any plans for releasing it to researchers or to other startups to encourage more competition and innovation?
また、競争やイノベーションを促進するために、研究者や他のスタートアップに公開する計画はあるのでしょうか?
Some of the requests that I've heard are unfettered interactions without safeguards to understand the model's performance, model specifications, including details on how it was trained, and access to the model itself, i.e.
私が聞いた要望の中には、モデルのパフォーマンスを理解するためのセーフガードなしの自由な対話、モデルの仕様(どのように訓練されたかの詳細を含む)、モデル自体へのアクセス(すなわち、訓練されたパラメータ)などがあります。
the trained parameters.
モデル自体へのアクセス、つまり学習されたパラメータへのアクセスです。
Do you want to comment on any of that?
それについて何かコメントはありますか?
Yeah.
はい。
I mean, I think it's related to our earlier question about open versus closed.
つまり、オープンかクローズかという先ほどの質問と関連していると思います。
I think that there are some intermediate approaches which can be very fruitful.
私は、非常に有益な中間的なアプローチもあると思います。
For example, model access and various combinations of that can be very, very productive because these neural networks already have such a large and complicated surface area of behavior.
たとえば、モデルアクセスやそのさまざまな組み合わせは、非常に生産的です。なぜなら、ニューラルネットワークは、すでに非常に大きく複雑な動作の表面積を有しているからです。
And studying that alone can be extremely interesting.
それだけを研究しても、非常に興味深いものになります。
We have an academic access program.
また、アカデミック・アクセス・プログラムもあります。
We provide various forms of access to the models.
様々な形でモデルへのアクセスを提供しています。
And in fact, plenty of academic research labs do study them in this way.
実際、多くの学術研究機関がこの方法でモデルを研究しています。
So I think this kind of approach is viable and is something that we are doing.
ですから、このようなアプローチは可能だと思いますし、私たちも行っています。
I know we're coming up on time.
時間が迫っているのは承知しています。
I want to end with just one final question, which is, can you just share any unintuitive but compelling use cases for how you love to use ChatGPT that others may not know about?
最後に1つだけ質問させてください。「ChatGPTの使い方で、他の人が知らないような、直感的ではないが説得力のある使用例があれば教えてください」ということです。
So I mean, I wouldn't say that it's unknown, but I really enjoy its poem writing ability.
つまり、知られていないとは言いませんが、詩を書く機能がとても気に入っています。
It can write poems.
詩を書くことができる。
It can rap.
ラップもできる。
It can be pretty amusing.
かなり面白いです。
And do you guys use it?
で、皆さんはそれを使っているのでしょうか?
Is it an integrated part of teamwork at Open?
オープンでは、チームワークの一部として組み込まれているのでしょうか?
I assume it is, but I'm curious, do you have any insights on how it changes dynamics with teams when you have AI deeply integrated into a human team and how they're working and any insights into what we may not know but that will come?
そうだと思いますが、AIが人間のチームに深く統合されると、チームのダイナミクスがどのように変化するのか、また、彼らがどのように働いているのか、そして、私たちが知らないだけで、これから起こるであろうことについての洞察はあるのでしょうか?
I would say today the best way to describe the impact is that everyone is a little bit more productive.
今日、このインパクトを表現するのに最適な方法は、すべての人の生産性が少し上がったということでしょう。
People are a little bit more on top of things.
物事に積極的に取り組むようになりました。
I wouldn't say that right now there is a dramatic impact on dynamics, which I can say, oh, yeah, the dynamics have shifted in this pronounced way.
今現在は、ああ、このように顕著にダイナミクスが変化した、と言えるような劇的なインパクトがあるとは言えません。
I'm curious if it depersonalizes conversations because it's the AI bot, but maybe we're not at that point yet where it's becoming insane.
AIボットだから会話を非人格化するのか気になるけど、まだ非常識になるような段階ではないのかもしれないね。
That I definitely don't think that's the case.
それは絶対にないと思います。
And I predict that will not be the case, but we'll see.
でも、まだその段階ではないと思っていますし、そうなるとは思っていません。
Well, thank you, Ilya, for a fascinating discussion.
さて、イリヤ、魅力的な議論をありがとう。
Time is always too short.
時間はいつも短すぎる。
You're always invited back to the farm.
またいつでも農場にお招きします。
We'd love to have you either virtually or in person.
バーチャルでも直接でも、ぜひお越しください。
So thank you, thank you, thank you.
それでは、ありがとうございました、ありがとうございました。
To our audience, thank you for tuning in for this session of the Entrepreneurial Thought Leader Series.
視聴者の皆さん、アントレプレナー・ソートリーダー・シリーズのこのセッションにご視聴いただき、ありがとうございました。
Next week, we're going to be joined by the executive chairman and co-founder of Okta, Frederick Karist.
来週は、Oktaのエグゼクティブ・チェアマン兼共同創業者であるフレデリック・カリスト氏をお招きします。
And you can find that event and other future events in this ETL series on our Stanford E-Corner YouTube channel.
このイベントやETLシリーズの今後のイベントは、スタンフォードE-CornerのYouTubeチャンネルでご覧いただけます。
And you'll find even more of the videos, podcasts, and articles about entrepreneurship and innovation at Stanford E-Corner.
また、起業やイノベーションに関するビデオ、ポッドキャスト、記事は、スタンフォードE-Cornerでご覧いただけます。
That's ecorner.stanford.edu.
ecorner.stanford.eduです。
And as always, thank you for tuning in to ETL.
そして、いつもETLにご視聴いただきありがとうございます。
And we'll see you next time on ETL.
また次回、ETLでお会いしましょう。