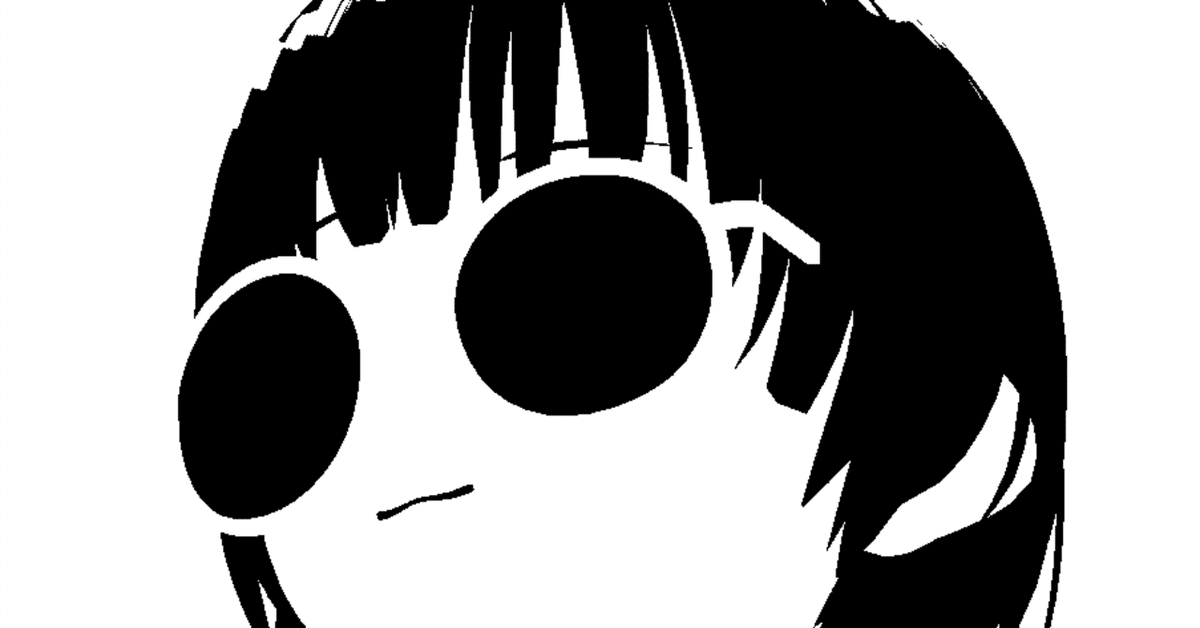
たまにはPythonとUnityでもちょもちょ
まずはPythonの環境をととのえます。
VSCodeはすでに入っているものとする。
Ctrl+@ でターミナルを開きます。
python -m venv .
フォルダと設定ファイルが出来上がります。
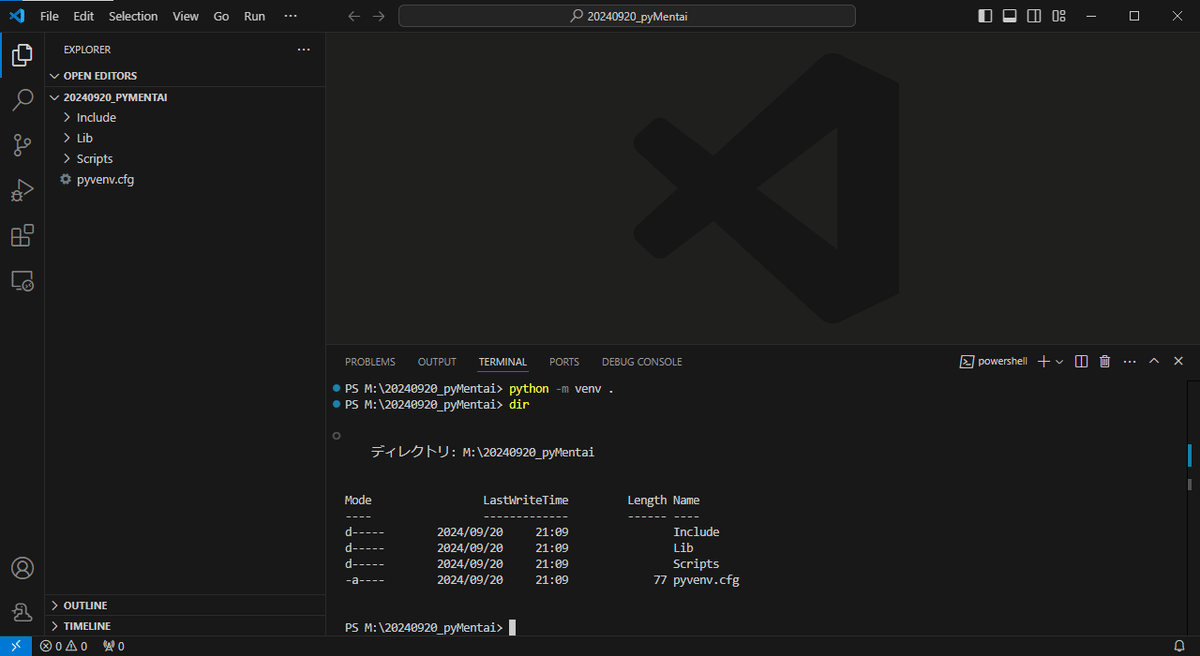
.\Scripts\Activate.ps1
仮想環境をアクティベートします。
これわすれると、システムのpythonがわにモジュール入っちゃうので注意。
手順に従ってインストール
pip install pyvirtualcam
つぎにソースを作成、お試しなので test1.pyとかでOK
import colorsys
import numpy as np
import pyvirtualcam
with pyvirtualcam.Camera(width=1280, height=720, fps=20) as cam:
print(f'Using virtual camera: {cam.device}')
frame = np.zeros((cam.height, cam.width, 3), np.uint8) # RGB
while True:
h, s, v = (cam.frames_sent % 100) / 100, 1.0, 1.0
r, g, b = colorsys.hsv_to_rgb(h, s, v)
frame[:] = (r * 255, g * 255, b * 255)
cam.send(frame)
cam.sleep_until_next_frame()
保存して、実行
> python.exe .\test1.py
Using virtual camera: OBS Virtual Camera
この状態で止まってるのが正常です。
もしだめならOBSがインストールされてないかも。
OBS起動して、ソースに映像キャプチャデバイス追加して、デバイスにOBS Virtual Camera を指定してなんだかギラギラした映像が出てきたらここまでOKです。OBSは閉じてしまおう。
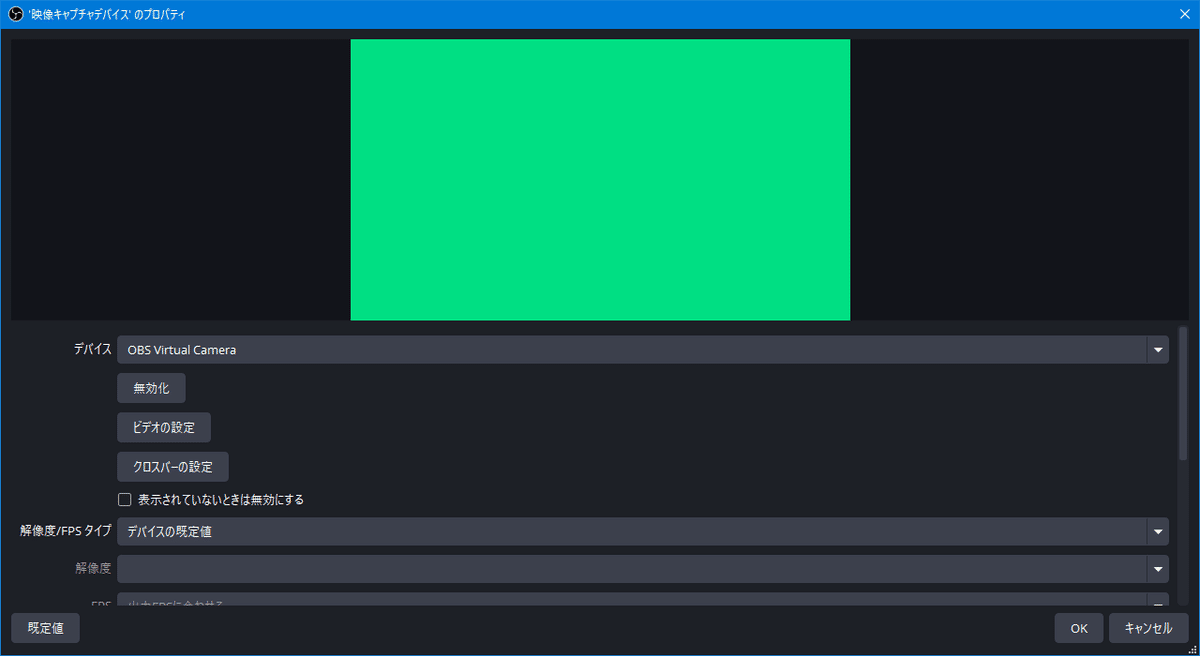
ここからは、Unity側作業です
Planeオブジェクト作って、下のスクリプトを WebCamController.cs で作って、アタッチ。
devices[3].name 3 の部分はカメラの数で変わるので OBS Virtual Camera に対応する番号に変えてね
using System.Collections;
using System.Collections.Generic;
using UnityEngine;
using UnityEngine.UI;
public class WebCamController : MonoBehaviour
{
int width = 1920;
int height = 1080;
int fps = 30;
WebCamTexture webcamTexture;
[SerializeField] WebCamDevice[] devices;
void Start()
{
devices = WebCamTexture.devices;
foreach (WebCamDevice device in devices)
{
Debug.Log("device.name => " + device.name);
}
webcamTexture = new WebCamTexture(devices[3].name, this.width, this.height, this.fps);
GetComponent<Renderer>().material.mainTexture = webcamTexture;
webcamTexture.Play();
}
// Update is called once per frame
void Update()
{
}
}
OBSと同じような感じのになるけどマテリアルのせいてテカってます。きになるならunlit系に変える
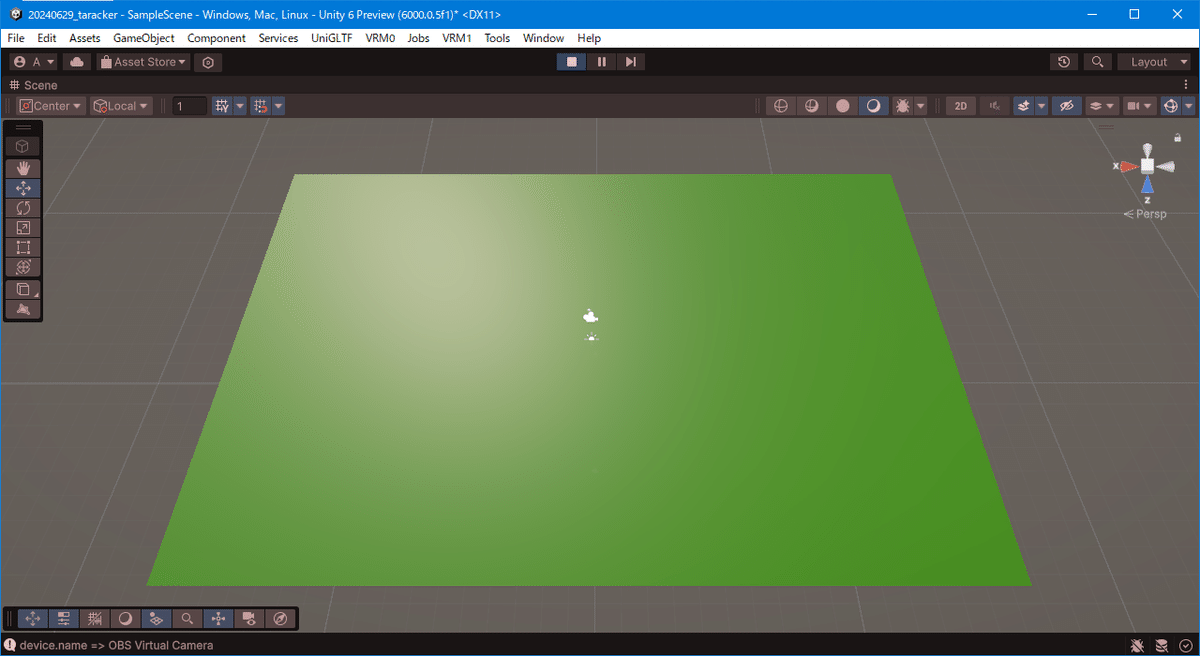
Pythonでの作業に戻ります。
mjpegを受け取ってフレームに入れてcam.sendです。
おいおい使うのでopencvのライブラリをいれます
pip install opencv-python
pip install opencv-contrib-python
おあそび!画像をギラギラさせる
test2_img.py
import colorsys
import numpy as np
import pyvirtualcam
import cv2
im = cv2.imread('img.jpg')
im_rgb = cv2.cvtColor(im,cv2.COLOR_BGR2RGB)
ang = 0
with pyvirtualcam.Camera(width=1280, height=720, fps=20) as cam:
print(f'Using virtual camera: {cam.device}')
frame = np.zeros((cam.height, cam.width, 3), np.uint8) # RGB
while True:
# h, s, v = (cam.frames_sent % 100) / 100, 1.0, 1.0
# r, g, b = colorsys.hsv_to_rgb(h, s, v)
# frame[:] = (r * 255, g * 255, b * 255)
ang = (ang + 10) % 180
hsv = cv2.cvtColor(im_rgb, cv2.COLOR_RGB2HSV)
hsv = hsv.astype(np.uint16) # uint8だと255以上で不定になるので型を変更
hsv[:,:,0] = (hsv[:,:,0]+ang)%180
hsv = hsv.astype(np.uint8) # 型を戻す
dst = cv2.cvtColor(hsv, cv2.COLOR_HSV2RGB)
cam.send(dst)
cam.sleep_until_next_frame()
本命!mjpegを仮想WEBCAMにしちゃう
test3_mjpeg.py
URLと、解像度は適切に変更
import colorsys
import numpy as np
import pyvirtualcam
import cv2
URL = "http://192.168.100.101"
AWB = True
# Face recognition and opencv setup
cap = cv2.VideoCapture(URL + ":81/stream")
with pyvirtualcam.Camera(width=320, height=240, fps=30) as cam:
print(f'Using virtual camera: {cam.device}')
frame = np.zeros((cam.height, cam.width, 3), np.uint8) # RGB
while True:
# h, s, v = (cam.frames_sent % 100) / 100, 1.0, 1.0
# r, g, b = colorsys.hsv_to_rgb(h, s, v)
# frame[:] = (r * 255, g * 255, b * 255)
ret, frame = cap.read()
cam.send(frame)
cam.sleep_until_next_frame()
コレも結構面白い!RTSPをWEBCAMに
おまけ。mediapipeをpythonで
必要になる追加のライブラリ
pip install python-osc
pip install mediapipe
face_landmarker.task を別途ダウンロードして同じフォルダに配置
test4_mp_fl.py
ソースはちょっと整理中
# Copyright 2023 The MediaPipe Authors. All Rights Reserved.
#
# Licensed under the Apache License, Version 2.0 (the "License");
# you may not use this file except in compliance with the License.
# You may obtain a copy of the License at
#
# http://www.apache.org/licenses/LICENSE-2.0
#
# Unless required by applicable law or agreed to in writing, software
# distributed under the License is distributed on an "AS IS" BASIS,
# WITHOUT WARRANTIES OR CONDITIONS OF ANY KIND, either express or implied.
# See the License for the specific language governing permissions and
# limitations under the License.
"""Main scripts to run face landmarker."""
import argparse
import sys
import time
from pythonosc import udp_client
import cv2
import mediapipe as mp
from mediapipe.tasks import python
from mediapipe.tasks.python import vision
from mediapipe.framework.formats import landmark_pb2
mp_face_mesh = mp.solutions.face_mesh
mp_drawing = mp.solutions.drawing_utils
mp_drawing_styles = mp.solutions.drawing_styles
# Global variables to calculate FPS
COUNTER, FPS = 0, 0
START_TIME = time.time()
DETECTION_RESULT = None
def run(model: str, num_faces: int,
min_face_detection_confidence: float,
min_face_presence_confidence: float, min_tracking_confidence: float,
camera_id: int, width: int, height: int) -> None:
"""Continuously run inference on images acquired from the camera.
Args:
model: Name of the face landmarker model bundle.
num_faces: Max number of faces that can be detected by the landmarker.
min_face_detection_confidence: The minimum confidence score for face
detection to be considered successful.
min_face_presence_confidence: The minimum confidence score of face
presence score in the face landmark detection.
min_tracking_confidence: The minimum confidence score for the face
tracking to be considered successful.
camera_id: The camera id to be passed to OpenCV.
width: The width of the frame captured from the camera.
height: The height of the frame captured from the camera.
"""
# Start capturing video input from the camera
# cap = cv2.VideoCapture(camera_id)
# cap.set(cv2.CAP_PROP_FRAME_WIDTH, width)
# cap.set(cv2.CAP_PROP_FRAME_HEIGHT, height)
URL = "http://192.168.100.101"
cap = cv2.VideoCapture(URL + ":81/stream")
cap.set(cv2.CAP_PROP_FRAME_WIDTH, width)
cap.set(cv2.CAP_PROP_FRAME_HEIGHT, height)
# Visualization parameters
row_size = 50 # pixels
left_margin = 24 # pixels
text_color = (0, 0, 0) # black
font_size = 1
font_thickness = 1
fps_avg_frame_count = 10
# Label box parameters
label_background_color = (255, 255, 255) # White
label_padding_width = 1500 # pixels
def save_result(result: vision.FaceLandmarkerResult,
unused_output_image: mp.Image, timestamp_ms: int):
global FPS, COUNTER, START_TIME, DETECTION_RESULT
# Calculate the FPS
if COUNTER % fps_avg_frame_count == 0:
FPS = fps_avg_frame_count / (time.time() - START_TIME)
START_TIME = time.time()
DETECTION_RESULT = result
COUNTER += 1
# Initialize the face landmarker model
base_options = python.BaseOptions(model_asset_path=model)
options = vision.FaceLandmarkerOptions(
base_options=base_options,
running_mode=vision.RunningMode.LIVE_STREAM,
num_faces=num_faces,
min_face_detection_confidence=min_face_detection_confidence,
min_face_presence_confidence=min_face_presence_confidence,
min_tracking_confidence=min_tracking_confidence,
output_face_blendshapes=True,
result_callback=save_result)
detector = vision.FaceLandmarker.create_from_options(options)
# Continuously capture images from the camera and run inference
while cap.isOpened():
success, image = cap.read()
if not success:
sys.exit(
'ERROR: Unable to read from webcam. Please verify your webcam settings.'
)
image = cv2.flip(image, 1)
# Convert the image from BGR to RGB as required by the TFLite model.
rgb_image = cv2.cvtColor(image, cv2.COLOR_BGR2RGB)
mp_image = mp.Image(image_format=mp.ImageFormat.SRGB, data=rgb_image)
# Run face landmarker using the model.
detector.detect_async(mp_image, time.time_ns() // 1_000_000)
# Show the FPS
fps_text = 'FPS = {:.1f}'.format(FPS)
text_location = (left_margin, row_size)
current_frame = image
cv2.putText(current_frame, fps_text, text_location,
cv2.FONT_HERSHEY_DUPLEX,
font_size, text_color, font_thickness, cv2.LINE_AA)
if DETECTION_RESULT:
face_blendshapes = DETECTION_RESULT.face_blendshapes
if face_blendshapes:
# print(vars(face_blendshapes[0]))
for idx, category in enumerate(face_blendshapes[0]):
category_name = category.category_name
score = round(category.score, 2)
if category_name.startswith('cheekPuff') :
print(vars(category))
if DETECTION_RESULT:
# Draw landmarks.
for face_landmarks in DETECTION_RESULT.face_landmarks:
face_landmarks_proto = landmark_pb2.NormalizedLandmarkList()
face_landmarks_proto.landmark.extend([
landmark_pb2.NormalizedLandmark(x=landmark.x,
y=landmark.y,
z=landmark.z) for
landmark in
face_landmarks
])
mp_drawing.draw_landmarks(
image=current_frame,
landmark_list=face_landmarks_proto,
connections=mp_face_mesh.FACEMESH_TESSELATION,
landmark_drawing_spec=None,
connection_drawing_spec=mp.solutions.drawing_styles
.get_default_face_mesh_tesselation_style())
mp_drawing.draw_landmarks(
image=current_frame,
landmark_list=face_landmarks_proto,
connections=mp_face_mesh.FACEMESH_CONTOURS,
landmark_drawing_spec=None,
connection_drawing_spec=mp.solutions.drawing_styles
.get_default_face_mesh_contours_style())
mp_drawing.draw_landmarks(
image=current_frame,
landmark_list=face_landmarks_proto,
connections=mp_face_mesh.FACEMESH_IRISES,
landmark_drawing_spec=None,
connection_drawing_spec=mp.solutions.drawing_styles
.get_default_face_mesh_iris_connections_style())
# Expand the right side frame to show the blendshapes.
current_frame = cv2.copyMakeBorder(current_frame, 0, 0, 0,
label_padding_width,
cv2.BORDER_CONSTANT, None,
label_background_color)
if DETECTION_RESULT:
# Define parameters for the bars and text
legend_x = current_frame.shape[
1] - label_padding_width + 20 # Starting X-coordinate (20 as a margin)
legend_y = 30 # Starting Y-coordinate
bar_max_width = label_padding_width - 40 # Max width of the bar with some margin
bar_height = 8 # Height of the bar
gap_between_bars = 5 # Gap between two bars
text_gap = 5 # Gap between the end of the text and the start of the bar
face_blendshapes = DETECTION_RESULT.face_blendshapes
if face_blendshapes:
for idx, category in enumerate(face_blendshapes[0]):
category_name = category.category_name
score = round(category.score, 2)
# Prepare text and get its width
text = "{} ({:.2f})".format(category_name, score)
(text_width, _), _ = cv2.getTextSize(text,
cv2.FONT_HERSHEY_SIMPLEX,
0.4, 1)
# Display the blendshape name and score
cv2.putText(current_frame, text,
(legend_x, legend_y + (bar_height // 2) + 5),
# Position adjusted for vertical centering
cv2.FONT_HERSHEY_SIMPLEX,
0.4, # Font size
(0, 0, 0), # Black color
1,
cv2.LINE_AA) # Thickness
# Calculate bar width based on score
bar_width = int(bar_max_width * score)
# Draw the bar to the right of the text
cv2.rectangle(current_frame,
(legend_x + text_width + text_gap, legend_y),
(legend_x + text_width + text_gap + bar_width,
legend_y + bar_height),
(0, 255, 0), # Green color
-1) # Filled bar
# Update the Y-coordinate for the next bar
legend_y += (bar_height + gap_between_bars)
cv2.imshow('face_landmarker', current_frame)
# Stop the program if the ESC key is pressed.
if cv2.waitKey(1) == 27:
break
detector.close()
cap.release()
cv2.destroyAllWindows()
def main():
parser = argparse.ArgumentParser(
formatter_class=argparse.ArgumentDefaultsHelpFormatter)
parser.add_argument(
'--model',
help='Name of face landmarker model.',
required=False,
default='face_landmarker.task')
parser.add_argument(
'--numFaces',
help='Max number of faces that can be detected by the landmarker.',
required=False,
default=1)
parser.add_argument(
'--minFaceDetectionConfidence',
help='The minimum confidence score for face detection to be considered '
'successful.',
required=False,
default=0.5)
parser.add_argument(
'--minFacePresenceConfidence',
help='The minimum confidence score of face presence score in the face '
'landmark detection.',
required=False,
default=0.5)
parser.add_argument(
'--minTrackingConfidence',
help='The minimum confidence score for the face tracking to be '
'considered successful.',
required=False,
default=0.5)
# Finding the camera ID can be very reliant on platform-dependent methods.
# One common approach is to use the fact that camera IDs are usually indexed sequentially by the OS, starting from 0.
# Here, we use OpenCV and create a VideoCapture object for each potential ID with 'cap = cv2.VideoCapture(i)'.
# If 'cap' is None or not 'cap.isOpened()', it indicates the camera ID is not available.
parser.add_argument(
'--cameraId', help='Id of camera.', required=False, default=0)
parser.add_argument(
'--frameWidth',
help='Width of frame to capture from camera.',
required=False,
default=1280)
parser.add_argument(
'--frameHeight',
help='Height of frame to capture from camera.',
required=False,
default=960)
args = parser.parse_args()
run(args.model, int(args.numFaces), args.minFaceDetectionConfidence,
args.minFacePresenceConfidence, args.minTrackingConfidence,
int(args.cameraId), args.frameWidth, args.frameHeight)
if __name__ == '__main__':
main()
以上!
この記事が気に入ったらサポートをしてみませんか?