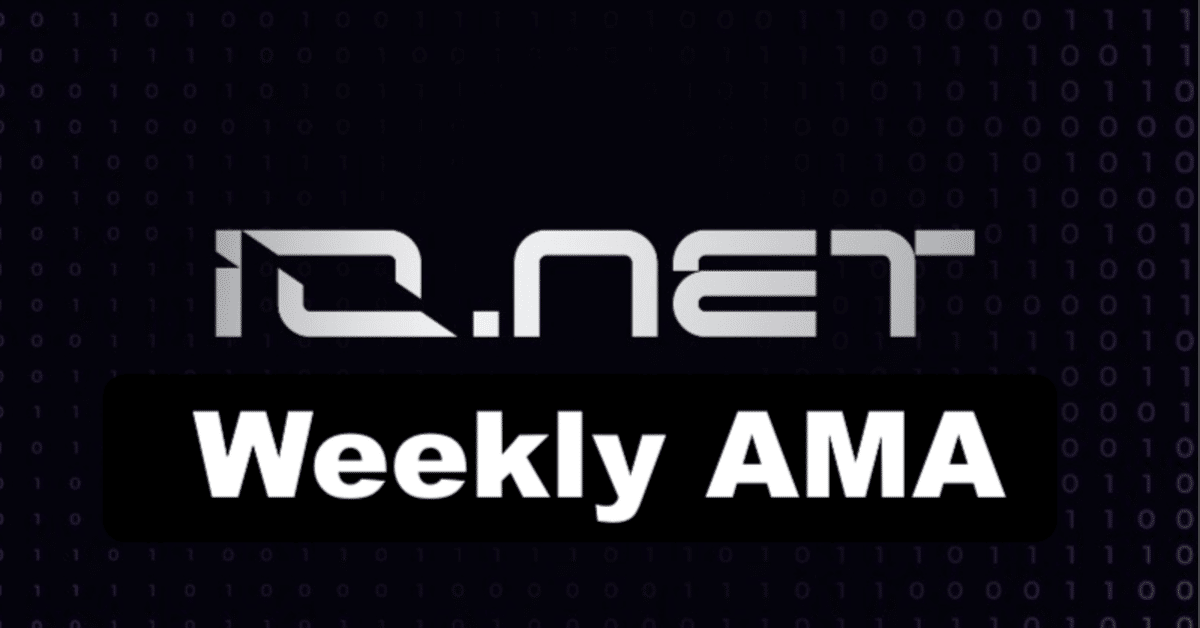
IO x OpenLedger Rountable AMA(日本語翻訳)
IO x OpenLedger Rountable AMA
— Gaurav_IO (@Gaurav_ionet) November 27, 2024
Io.netとOpen Ledger AMA - 主要なハイライトと要約
ホスト: Milan(Io.netのマーケティングVP)
参加者:
Io.net: Gaurav(CTO)、Tausif(BDおよびパートナーシップ責任者)
Open Ledger: Ram(コアコントリビューター)
目的:
Io.netとOpen Ledgerをコミュニティに紹介し、パートナーシップについて議論し、視聴者からの質問に回答。
Io.netについて
GPU向けのDePIN(Decentralized Physical Infrastructure Network)としてスタート。
モデル構築、提供、デプロイメントのためのツールを提供する、エンドツーエンドのAIエコシステムに進化。
焦点: 分散型インフラを通じてAIの民主化を目指す。
Open Ledgerについて
データ帰属、モデルトレーニング、AIサービスに焦点を当てた分散型データブロックチェーン。
主な特徴:
Data Nets: 特定の分野に特化したデータ貢献。
専門モデル: 提供されたデータを基に構築・微調整。
分散型インフラ: 信頼性とデータ帰属を確保。
Polychainを含む主要な支援者から800万ドルの資金を調達。
主な議論ポイント
LLMs(Large Language Models) vs. SLMs(Small Language Models)
LLMs: 汎用的でリソース集約型。
SLMs: 特定タスクに最適化された効率的なモデル。
未来: LLMsのコンテキスト能力とSLMsの精度を活用するハイブリッドアプローチ。
パートナーシップとコラボレーション
Io.netとOpen Ledgerは、計算インフラとデータ帰属機能を組み合わせることでAIの分散化を目指す。
パートナーシップは、分散型で民主化されたAIエコシステムを構築するビジョンと一致する必要がある。
データ帰属とコミュニティインセンティブ
重要性: データ提供者への透明性、信頼、報酬。
Io.net: クリーンで偏りのないデータを提供する貢献者にトークンで報酬を与える計画。
Open Ledger: ブロックチェーンを活用してデータ帰属を保証し、影響に基づいて報酬を提供。
AIエージェント
定義: AIを使用して人間の介入なしにタスクを実行する自律システム。
応用例: チャットボット、フィットネストラッキング、語学学習。
ビジョン: Io.netは多様な用途に対応するハイブリッドAIエージェントの構築を目指している。
三層モデル
データ貢献: ブロックチェーンを通じたデータの出所とインセンティブ化(Open Ledgerの焦点)。
モデル構築: モデルのトレーニングとデプロイメント用プラットフォーム(Io.netの次の段階)。
アプリケーション: コミュニティ向けにオープンソースAIモデルへの無料アクセスを提供(Io.netの現在の焦点)。
コミュニティエンゲージメント
Io.net: 2024年1月までにユーザーが独自のAIモデルをデプロイし、報酬を獲得できるようにする計画。
Open Ledger: 企業や個人がデータを透明に収益化し帰属できるよう支援。
締めの言葉
Io.netとOpen Ledgerのパートナーシップは、計算インフラとデータ帰属における補完的な強みを示している。
両社は、分散型AIの進展、貢献者のエンパワーメント、コラボレーションの促進に尽力している。
コミュニティ参加と透明性のある報酬が彼らの使命の中心にある。
全文翻訳
オープニング
さて、IonetとOpenLedgerのラウンドテーブルAMAにご参加いただきありがとうございます。私はMilanと申します。本日司会を務めます。
Ionetのマーケティング担当VPでもあります。本当にここにいることをうれしく思います。素晴らしいAMAになることでしょう。たくさんの内容がありますので、一緒に解き明かしていきましょう。
最初に簡単な自己紹介から始めます。
今日はIonationのメンバーだけでなく、OpenLedgerの方々も多く参加されています。
それでは、Gaurav、OpenLedgerの参加者の方々がIonetについて十分に知らない可能性がありますので、Ionetが何をしているのかを簡単に説明していただけますか?数文で教えてください。
Gauravの回答
こんにちは、皆さん。私たちは基本的にDePIN(分散型物理インフラネットワーク)としての旅を始めました。そして、誕生から6ヶ月以内で、GPU市場で最大級のDePINの1つとなりました。
その後、AI全般を扱うプレイヤーとして、単にGPUを提供するだけでなく、モデルを構築し、提供するためのプラットフォームを提供する、AIエコシステム全体を構築する旅を開始しました。
私たちは現在、AIに関連するあらゆることを可能にするプラットフォームを目指しています。
素晴らしい、共有ありがとうございます、Gaurav。
それでは、Ramさんに伺います。OpenLedgerが何をしているのかを知らない方々のために、簡単にご説明いただけますか?
Ramの回答
まず最初に、この場に招いていただきありがとうございます。皆さんと一緒にいられてうれしいです。そしてGauravさん、お久しぶりです。
さて、OpenLedgerはAI用のデータブロックチェーンです。私たちは、専門的なモデルを構築するための分散型信頼インフラを提供しています。
私たちのプラットフォームには3つの主要なステークホルダーがいます。
1つ目は「データネット」。ここでは、人々がユニークなデータセットを提供します。これらはドメインに特化したデータセットと考えてください。
2つ目は、これらのデータセットを使って専門的なモデルを構築します。具体的には、データセットを微調整してモデルを作成します。
3つ目は、これらのモデルがエージェント、アプリ、またはAIサービスで推論されることです。
これがOpenLedgerの仕組みです。この全体を調整するプラットフォームを構築しています。
共有ありがとうございます、Ramさん。
OpenLedgerでのご自身の役割についても教えていただけますか?
Ramの回答
私はOpenLedgerのコア貢献者の1人です。
2017年からこの業界に携わり、主にブロックチェーンの研究開発を行ってきました。Polygon、Hedera Hashgraph、Aptosなどの主要ブロックチェーンと協力してきました。
また、ViacomやFlipkart(Walmartのインド子会社)などのWeb2プロジェクトをWeb3に移行させるプロジェクトにも取り組んできました。
現在、OpenLedgerは約1年間取り組んでいるプロジェクトで、Polychainが主導するラウンドで800万ドルの資金を調達しました。Borderless Capitalや他の興味深い投資家も加わっています。
素晴らしいですね、Ramさん。共有いただきありがとうございます。
Gauravについて知らない方のために簡単に紹介します。Gauravは私たちの最高技術責任者(CTO)で、皆さんが見ている素晴らしい技術を担当しています。そして、Tausifは私たちのビジネス開発およびパートナーシップを率いています。
Milanの進行
次に進む前に、コミュニティメンバーの皆さんにお知らせがあります。
今回のAMAでは、参加者の中から賞品が当たるチャンスがあります!
AMAに参加しているスクリーンショットを撮り、それをOpenLedgerのコミュニティDiscordチャンネルに投稿してください。
最初の50名に賞品が当たりますので、ぜひ参加してみてくださいね。
さて、最初の質問に進みましょう。
Milanの質問
最初の質問です。
LLM(Large Language Models)とSLM(Small Language Models)の未来をどのように考えているか、というものです。
IonetやOpenLedgerのような企業が、それぞれどのようにLLMとSLMの将来を捉えているのかを説明してください。
LLMは大規模言語モデル、SLMは小規模言語モデルのことです。これらの技術がどの方向に進むのか、ぜひお聞かせください。
まずRamさん、あなたからお願いします。
Ramの回答
はい、まさにおっしゃる通りです。今日のモデルの多くはLLM、大規模言語モデルです。
たとえば、ChatGPTはOpenAIのモデルを使用していますし、MetaにはLLamaというAIインターフェイスがあります。
その他にも市場にはいくつかモデルがありますし、本当にオープンソースのモデルもいくつか存在しています。
LLMを世界の基本的な一般知識と考えることができます。インターネット上の豊富なデータや一般的なデータを含み、英語の理解、人間同士のやり取りの基本的な理解を提供します。
一方で、SLM、つまり専門的なモデルは少し異なります。AIが私たちの日常生活において中心的な技術になると考えるならば、特定のドメインに特化したモデルが必要になります。
これらの小規模なモデルはLLMと共存し、補完関係にあると考えています。たとえば、LLMが文脈を理解し、質問が何についてなのかを把握する一方で、SLMがその文脈をもとに正確な回答を提供する仕組みです。
たとえば、インターネットからデータを収集し文脈を理解するためにLLMを使用し、特定の数式の問題に対してはSLMを使用して解答を生成する、といった具合です。
SLMはLLMに比べて計算リソースやデータの消費が少なく、トレーニングにかかる時間も短縮されます。また、非常に特定のデータで訓練されるため、誤解や間違いが少ないのが特徴です。
Andreij Karpathyの有名な引用に「LLMはOSであり、SLMはその上に構築されるアプリのようなものだ」とありますが、まさにそのような未来が訪れると信じています。
Milanの進行
素晴らしいですね。Ramさん、共有いただきありがとうございます。
それでは、Gauravさんにお聞きします。同じ質問になりますが、Ionetの視点でLLMとSLMの将来をどのように捉えていますか?
Gauravの回答
Ramさんがほとんどのポイントをカバーされましたが、私も同じ見解を持っています。
LLMとSLMはお互いに補完的なツールです。
LLMは複雑で汎用的なタスクを得意とします。しかし、それゆえに非常にリソースを消費します。一方で、SLMは特定のタスクに焦点を当てているため、高い性能、エネルギー効率、そして適応性を持っています。
SLMはエッジデバイスや特定のタスクに最適です。
たとえば、以前私が勤務していたBooking Holdings Groupでは、LLMをカスタマーサポートの自動化に使用している例を見ました。航空会社や銀行はLLMを使用して、さまざまなトピックの顧客の質問に対応できます。
LLM搭載のチャットボットは文脈を理解し、シームレスな回答を提供します。たとえば、予約のキャンセルや近隣のホテルの提案といった複雑なリクエストにも対応できます。
一方、SLMの優位性が発揮されるのは、たとえばフィットネストラッカーのようなデバイスです。SLMはトレーニングデータをもとに、個人のワークアウトパターンを分析し、特化したアドバイスを提供します。
これらのモデルは少量のデータで十分に動作し、必要な計算リソースも少なく、プライバシー保護の点でも優れています。
将来的には、LLMとSLMを組み合わせたハイブリッドな活用が進むと予想しています。業界はそれぞれの利点を活かしながら、タスクに応じて最適なツールを選択するようになるでしょう。
Milanの進行
Gauravさん、ありがとうございます。本当に興味深い内容ですね。
IonetとOpenLedgerが、これらの技術の将来について共通のビジョンを持っていることがわかりました。
それでは次に、パートナーシップについて話を進めましょう。
パートナーシップは、特にWeb3のエコシステムにおいて、目標を達成するうえで非常に重要な役割を果たします。
Tausifさんに質問です。Ionetのミッションを進める上で、パートナーシップはどのような役割を果たしますか? また、コラボレーションの際に重視する点は何ですか?
Tausifの回答
もちろんです。パートナーシップは、この分野を前進させるうえで重要な要素です。
もし視点を少し広げて、IonetやOpenLedgerがどのように取り組んでいるかを見ると、私たちはAIの技術スタックを分散化することを目指しています。
Ionetは、分散型で世界中にアクセス可能な計算能力を提供することに焦点を当てています。一方で、OpenLedgerは、信頼できるデータプラットフォームの提供に注力しています。
エコシステム全体を見渡すと、他のプレイヤーがさまざまなレイヤーで実験しており、アプリケーションやビジネスモデルを模索して、消費者にとって意味のあるものを構築しています。
これらのパートナーシップは、単独で取り組むよりも、各チームの強みを活かしてより大きな成果を生み出すものです。たとえば、IonetとOpenLedgerのコラボレーションはその良い例です。
Ionetは計算能力に焦点を当てており、OpenLedgerはデータの検証と帰属の専門性を持っています。それぞれが異なるレイヤーで構築しながらも、組み合わせることで非常に効果的な結果を生み出しています。
Web2では、ChatGPTのような技術が人々の仕事や生産性の向上に統合されましたが、Web3でも同様に、分散型AIの成功には、エコシステム全体の協力が必要です。
このようなパートナーシップは、分散型AIを現実のものにするために不可欠です。
Milanの進行
ありがとうございます、Tausifさん。とてもよくわかりました。
それでは、Ramさんにお聞きします。OpenLedgerの視点から見た場合、パートナーシップの重要性や、パートナー選定の際に重視するポイントは何ですか?
Ramの回答
私もTausifさんの意見に全面的に同意します。ただし、1つ追加するならば、私は単なる「名ばかりのパートナーシップ」を信じません。形だけのパートナーシップでは、実りある結果は得られません。
私たちのパートナーシップは非常に具体的で、私たちの技術スタックに意味のある形で統合される必要があります。
たとえば、OpenLedgerではドメイン固有のモデルを構築するためにデータを収集していますが、それを細かく調整するためには計算能力が必要です。このニーズを満たすために、計算能力を提供する企業とのパートナーシップが不可欠です。
パートナー選定の際には、コスト効率、分散化、統合のしやすさを重視しています。
Ionetとのパートナーシップは自然な選択でした。彼らは分散型の計算能力を提供し、それが私たちのビジョンと完全に一致しています。また、コスト面でも非常に優れています。
さらに、私たちはエンドユーザーに利益をもたらす機能を向上させるためのパートナーとも連携しています。
Milanの進行
ありがとうございます、Ramさん。パートナーシップにおける視点が非常に明確ですね。
次はデータの帰属(アトリビューション)とコミュニティの関与について話を進めます。
Gauravさん、次の質問です。データの帰属がなぜ重要なのか、また、コミュニティがどのように参加できるのかを教えてください。
Gauravの回答
モデルを構築したことがある人には明らかですが、モデル作成プロセスの中で最大の労力が費やされるのは、データの収集、クリーニング、そしてモデルが利用可能な形に整える作業です。このプロセスには全体の70~80%が費やされます。
モデルの性能は、入力されるデータの質によって決まります。
中央集権型のプレイヤー(たとえばGoogleやAmazon)は、すでに多くのプロダクトを持っており、これらからデータを大量に収集しています。たとえばGoogleは、チャット、Gmail、そしてさまざまなサービスを通じてデータを収集しています。そして、これらを活用して強力なモデルを構築しています。
しかし、分散型AIエージェントを構築したい場合、私たちにはクリーンで偏りのないデータが必要です。そのため、データを提供してくれる人たちに動機を与える仕組みが必要です。
ここでデータの帰属が重要になります。誰がデータを提供したのか、そのデータがどのように使用されるのかを明確にすることで、データ提供者の権利を守ります。
さらに、多くの暗号プロジェクトはデータ提供者にトークンを報酬として与える仕組みを導入しています。これにより、信頼が生まれ、コミュニティがデータを共有する動機付けになります。
これがデータの帰属が重要である理由であり、これを活用して分散型エコシステムを構築するための基盤が形成されます。
Milanの進行
ありがとうございます、Gauravさん。非常にわかりやすい説明でした。
それでは、Tausifさん、ビジネスの視点から見て、データの帰属がなぜ重要なのか、またコミュニティがどのように利益を得ることができるのか、ぜひお聞かせください。
Tausifの回答
その通りですね。データの帰属は、AIの主流化、特に分散型AIを広める上で鍵となる要素だと思います。
AIに関わっていない人々が、AIをどのように受け止めているかを考えてみてください。多くの人は、AIが自分の仕事を奪ったり、自分の努力を利用してしまうのではないかと恐れています。
データ帰属の仕組みを導入することで、人々はAI開発の成功に自分も参加していると感じられるようになります。
たとえば、昨日OpenAIの動画モデルがHugging Face上でリークされました。その理由は、アーティストが自分たちの作品がモデルのトレーニングに無断で使用されたと主張したことによります。
このような事例は、透明性のある帰属システムの必要性を強く示しています。人々が自分の貢献が評価され、適切に補償されると感じることができれば、AIを受け入れ、さらに成長させることができるのです。
データの帰属は単なる公平性の問題にとどまらず、持続可能なエコシステムを作るための重要な要素です。
Milanの進行
素晴らしいポイントですね、Tausifさん。ありがとうございます。
Ramさん、それではOpenLedgerとしての視点をお聞かせください。データの帰属についてどのように考えており、どのようにコミュニティを巻き込んでいますか?
Ramの回答
GauravさんとTausifさんが述べられたように、データの帰属は特に分散型AIエコシステムにおいて不可欠です。
OpenLedgerでは、データの品質と帰属を保証するために、2つの主要な方法を採用しています。
技術的検証:
私たちは、Eigenvalidators(データ検証エンジン)を使用して、提供されたデータの品質を検証しています。これらのエンジンはデータを非公開で検証し、プライバシーを保護します。ネガティブインセンティブ:
提供されたデータが有用でない場合、報酬や帰属が行われません。これにより、ジャンクデータの提供が自然と抑制されます。
また、すべてのデータポイントをオンチェーンで記録することで透明性を確保しています。データ提供者は、自分のデータがどのように使用され、どのモデルに影響を与えたか、そしてどのように報酬を得られるのかを確認できます。
これにより、信頼できる効率的なシステムを構築し、コミュニティが価値のあるデータを提供する動機を与えています。
Milanの進行
Ramさん、ありがとうございます。透明性と信頼性について非常に説得力のある説明ですね。
次に、「3つのレイヤー」モデル、つまりデータ貢献、モデル構築、アプリについてお話を進めたいと思います。
Ramさん、これらのレイヤーがどのように連携し、エコシステム全体にどのような利益をもたらすのか教えていただけますか?
Ramの回答
もちろんです。この3つのレイヤー(データ貢献、モデル構築、アプリ)は、分散型AIの中核を成すものです。
データ貢献:
これは基礎となるレイヤーです。個人や企業がデータセットを提供します。OpenLedgerでは、すべてのデータをオンチェーンで追跡し、透明性を確保しています。提供者は、自分のデータがどのようにモデルに影響を与えたかを確認できます。モデル構築:
データが収集されると、それを使ってドメイン特化型のモデルを構築します。モデル構築者は、どのデータが推論精度を向上させるかを理解し、それを活用してアルゴリズムを微調整します。アプリ:
これは最終的にエンドユーザーが触れる製品です。たとえば、カスタマーサポートをAIで強化するアプリなどです。これらのアプリは、訓練済みモデルを使用してユーザーに価値を提供します。
これらのレイヤーを統合することで、完全に透明な分散型エコシステムを実現します。データ提供者、モデル構築者、アプリ開発者の全員が利益を享受し、ウィンウィンの関係を築くことができます。
Milanの進行
素晴らしい解説ですね、Ramさん。
それでは、Gauravさん、Ionetではこれらのレイヤーにどのようにアプローチしているのか教えていただけますか?
Gauravの回答
Ionetでは、少し異なるアプローチを取っています。データ貢献から始めるのではなく、計算インフラの構築から始めました。
以下が、私たちが構築している3つのレイヤーの概要です。
アプリ(最上位レイヤー):
最初の優先事項は、ユーザーが強力なAIモデルに無料でアクセスできるようにすることです。たとえば、既存のトレーニング済みモデルをGPUインフラにデプロイし、コミュニティがすぐに利用できるようにします。モデル構築(中間レイヤー):
第2フェーズでは、ユーザーが自分のデータセットをアップロードしてモデルを訓練・微調整できるプラットフォームを提供します。また、モデルのデプロイやスケーリングも可能にします。機械学習エンジニアが複雑なインフラを気にせず、モデル構築に専念できる環境を整備します。データ貢献(基礎レイヤー):
最終的には、OpenLedgerに似た分散型システムを構築します。これにより、個人や企業がデータセットを共有し、そのプロセスが完全に透明化されます。
この段階的なアプローチにより、コミュニティにすぐに価値を提供しつつ、長期的な成長の基盤を構築しています。
Milanの進行
Gauravさん、非常に興味深い戦略ですね。
それではAMAの最後に、コミュニティから寄せられた質問に答えていきたいと思います。
Milanの進行
AMAの最後に、コミュニティから寄せられた3つの質問に答えます。
それぞれの質問について、Gauravさん、Tausifさん、Ramさんにお答えいただきます。
質問 1: Tausifさんへの質問
「コラボレーションを魅力的だと感じるのはどのような点ですか?また、その成功を予感させる要素は何ですか?」
Tausifの回答
素晴らしい質問ですね。
私がコラボレーションに魅力を感じるのは、両者の強みが互いを補完し合う瞬間です。
たとえば、Ionetは計算インフラに特化していますが、OpenLedgerはデータ検証と帰属の専門性を持っています。この2つを組み合わせることで、分散型AIスタックの異なるレイヤーにおいて非常に強力なソリューションを提供できます。
また、私はパートナーがどのようなニッチ分野に取り組んでいるのか、その「キラーアプリ」をどのように構築しようとしているのかを理解しようとします。それが私たちの製品ロードマップやビジョンにどのように適合するかを検討するのです。
さらに、両チームが同じように情熱を持って取り組んでいる場合、そのコラボレーションは成功しやすいと感じます。強い目的意識と明確な目標を共有することで、ゼロから1へと飛躍する可能性が高まります。
IonetとOpenLedgerのパートナーシップは、まさにその好例です。それぞれが自分たちの専門分野に集中しつつ、互いに補完し合っています。これこそが、私が理想とする成功するコラボレーションの形です。
質問 2: Gauravさんへの質問
「Ionetのプラットフォームで、コミュニティメンバーがAIモデルを直接テストする機会はありますか?」
Gauravの回答
もちろんあります!
まず、数週間以内に、コミュニティが事前トレーニング済みのAIモデルに無料でアクセスできるプラットフォームをリリースします。
さらに、2024年1月には、Ionet Intelligenceの第2フェーズを開始します。このフェーズでは、ユーザーが自分のモデルをアップロードし、GPUでデプロイしてテストできるようになります。
特に高性能なモデルには、GPUクレジットやトークン報酬を提供し、開発をさらに促進します。
私たちの目標は、コミュニティが自由に実験し、価値を生み出せる環境を提供することです。今後の展開にぜひご期待ください!
質問 3: Ramさんへの質問
「OpenLedgerは、データ所有権と帰属についてどのように革命を起こそうとしているのですか?また、それがAIや分散型技術全体にどのような影響を与えると考えていますか?」
Ramの回答
非常に良い質問です。
OpenLedgerは、データ所有権を提供者に返すことを目指しています。
現在、私たちはすべてのデータポイントをオンチェーンで記録し、完全な透明性を確保しています。これにより、データ提供者は、自分のデータがどのように使用され、どのモデルに影響を与えたかを確認できます。また、それに応じた報酬も得られる仕組みを提供しています。
これにより、企業や個人がより質の高いデータを提供するインセンティブが生まれます。それはAIモデルの品質向上につながり、最終的にはエコシステム全体に利益をもたらします。
さらに、AIが私たちの日常生活の中で中心的な役割を果たすようになるにつれて、その基盤が中央集権的であると多くの問題が生じます。分散型モデルであれば、個人や企業がAIの成長に貢献しながら、その恩恵を公平に享受できます。
私たちは、YouTubeがコンテンツ制作者に収益のチャンスを提供したのと同様に、データ提供者が分散型AIの成功から収益を得られる未来を目指しています。これが、OpenLedgerがAIと分散型技術全体に与える最大の影響だと考えています。
クロージング
Gauravさん、Tausifさん、Ramさん、本日はお時間をいただきありがとうございました。
そして、AMAにご参加いただいた皆さんも、本当にありがとうございました。
このAMAに参加しているスクリーンショットを撮り、OpenLedgerのDiscordチャンネルに投稿してください。最初の50名の方には賞品が当たります!
分散型AIの未来は非常に明るいと信じています。IonetとOpenLedgerのパートナーシップが、この業界に与える影響を楽しみにしています。
Ramさん、最後にもう一言お願いします。
この場に参加できたことを光栄に思います。引き続き、皆さんとのコラボレーションを楽しみにしています。
素晴らしいですね。ありがとうございました。皆さん、良い一日をお過ごしください!
英語原文
Gaurav, can you hear me okay?
Yeah, I can hear you.
Fantastic. Is that Ram speaking?
All right, brilliant. We have here Gaurav, we have Tarseev. I think Tarseev is just joining in.
Gaurav, do you want to just do a quick mic check, make sure that everyone can hear you loud and clear?
I think we're just going to give Gaurav 30 more seconds, but super excited to be here, guys.
Welcome, welcome, welcome. We are thrilled to have this conversation today. We're at OpenLedger. It's a roundtable AMA discussion.
We're just going to give it maybe a couple more minutes and then we'll get started.
Tarseev there, Gaurav, you'll just need to make Tarseev also a speaker. Tarseev, if you can request to speak, we'll get you added on.
Tidy up a bit of these things and then we can get started.
Gaurav, can you hear us okay? Just checking in from your side.
We can't seem to hear you right now.
Okay, guys, just bear with us about 30 more seconds. Gaurav is just figuring out.
Hello, can you hear me now?
Yes, I can hear you. Loud and clear.
Welcome, welcome, Gaurav. Can you hear us okay, Gaurav, just checking in?
Yes, I can hear you.
Perfect. Amazing, amazing.
Well, thank you all for joining the Ionet and OpenLedger roundtable AMA. My name is Milan. I will be your host today.
I'm also the VP of Marketing at Ionet. Super, super thrilled to be here. It's going to be a fantastic AMA. There's a lot to unpack here.
And so, you know, we're just going to kick it off with a brief round of introductions.
Today, we have Ionation on the call, but we also have a lot of people from OpenLedger.
And with that being said, Gaurav, you just want to introduce what Ionet is for those people in the audience who are from OpenLedger who may not be 100% familiar with us? We can just give them a couple of sentences on what Ionet is.
Hi, guys. We're basically, we started our journey as a deep end. And in the first six months of our, we can say, birth, we became one of the biggest deep ends in the market for the GPUs.
And then now we basically have started our journey to become an end-to-end AI player, which is creating a whole ecosystem around not just giving the GPUs currently, but now we give a platform to build models, serve models, basically a whole ecosystem around the AI play.
That's where we're headed. So we started with the deep end, and now we're creating a platform where people can do anything or everything to do with AI going forward.
Brilliant. Thank you so much for sharing that, Gaurav.
And Ram, over to you. For those of the audience who may not be 100% familiar in terms of what OpenLedger is doing, do you want to give a few sentences on what you guys are all about?
Yeah. First of all, thanks for having me here. And I'm glad to be among you guys. And good to meet you again, Gaurav.
So yeah, OpenLedger is a data blockchain for AI. We provide decentralized trust infrastructure for specialized models to be built on top of us.
And the three major stakeholders in our platform, one is data nets, where people contribute unique data sets. Think of these to be domain-specific data sets.
And then these data sets are trained to build specialized models on top of us. Basically, they fine-tune models of these data sets.
And then these models are then inferred by agents or apps or any kind of AI services that would need inference of models.
So that's what OpenLedger is. We are building this platform which orchestrates all of this together.
Thank you so much for sharing that, Ram.
And for those who are unfamiliar, Ram, what is your role at OpenLedger?
I'm one of the core contributors of OpenLedger.
I've been in the industry from 2017, majorly in building a blockchain R&D firm. We've worked with pretty much some of the major blockchains like Polygon, Hedon Hashgraph, Aptos, and many more.
We've also spent a good amount of time working with a lot of Web2 projects, bringing them to Web3.
Some of them are Viacom, Walmart's India subsidiary Flipkart, and many more as well.
So that's sort of our background. OpenLedger is something that we've been working on for the last year or so.
We've raised about 8 million, Polychain led the round. And we have Borderless Capital and some more interesting investors who are part of our cap table. And we're excited about what we're building.
Fantastic. Thank you so much for that, Ram.
And for those who are unfamiliar with Gaurav, Tausif, Gaurav is our Chief Technology Officer, doing all of the cool tech things that you guys are seeing.
And Tausifheads up all of our BD and partnerships. So I know you guys see a tremendous amount of partnerships that we're hosting on our X account and so much more.
And that is all thanks to Tausif. So just by way of introduction, that is the IOT team.
Now before we begin, it is worth me mentioning one thing, which is super important for our community members.
So for those of you who are perhaps a little bit new to either Ionet or OpenLedger, there is a prize to be won from this particular AMA.
All you need to do is take a screenshot of you being in the AMA and post it in the OpenLedger community and Discord channel.
I'm sure there's a lot of community moderators and astronauts from the IO team with the links, but there are prizes to be won there.
So if you are listening in, why not win something in the process as well, right?
So just by way, just as an FYI, there are some cool prizes there.
But thank you all for joining us today. We have over 100 people listening in, and that number is going to keep on increasing.
Now, with that being said, guys, we're going to go into our very first question.
So, guys, this question is about really about envisioning the future of LLMs and SLMs.
And what the community has been asking is really about explaining how individual companies, whether it's Ionet, whether it's OpenLedger, how they're viewing the future of LLMs.
And for those of you who don't know, LLMs are basically large language models and SLMs, which are small language models.
And where we really see these technologies heading.
So over to you, Ram, I'm going to kick it off by just presenting this question to you first.
What are your thoughts on that?
[続きます...]
Ram's Response:
Yeah, so as you had rightly put, most of the models today are large language models (LLMs). Any apps, like ChatGPT, run OpenAI's model, and Meta has its own AI interfaces that use LLaMA as a model.
There are a few others as well in the market, and a couple of truly open-source models too. I would consider large language models as your basic general knowledge of the world. They have a vast collection of data, including the Internet's generalized knowledge, giving an understanding of language and basic human interaction.
Small language models (SLMs), or specialized models, are different. If AI becomes as central to our daily lives as we predict—akin to electricity being ubiquitous—then domain-specific models will play a crucial role.
These smaller models will work hand-in-hand with LLMs. For instance, apps today and tomorrow could use an LLM for general context and SLMs for accurate, domain-specific tasks.
Let me give you an example. A model might use an LLM to scrape the internet and understand the general context of a query. Then, for a math-related task, it could pass that context to a domain-specific SLM to provide a precise and accurate response.
Domain-specific SLMs are much more efficient in terms of computational resources, data consumption, and training time. They also tend to hallucinate less, as they work with very specific data tailored to specialized tasks.
Andrej Karpathy has a famous quote likening LLMs to operating systems and SLMs to applications built on top of them. That's where we see the industry evolving. LLMs provide a broad base, and SLMs add targeted functionality.
Milan's Transition:
Fantastic. Thank you so much for that, Ram. Super insightful.
Gaurav's Response:
I think Ram has predominantly covered most of the aspects, and I share a similar view.
LLMs and SLMs are complementary tools. LLMs excel in handling complex, general-purpose tasks. However, they are resource-intensive because of their vast scope and generality.
SLMs, on the other hand, focus on specific tasks. They are highly performant, energy-efficient, and adaptable. These qualities make them perfect for edge devices or specialized tasks.
For example, let's say I previously worked at Booking Holdings Group. Over there, I saw companies adopting LLMs for customer support automation. Airlines or banks could use an LLM to answer queries across multiple topics. LLM-powered chatbots can understand context and provide seamless responses, like handling cancellations or suggesting nearby hotels.
SLMs, however, excel in focused tasks, such as fitness trackers. An SLM could analyze workout patterns and suggest tailored activities. Because it processes smaller, more relevant datasets, it consumes less power and offers better data privacy.
I foresee a hybrid future where LLMs and SLMs coexist. Industries will leverage both, choosing the right tool depending on the task's scope and requirements.
Milan's Transition:
Thank you so much for that, Gaurav. Really interesting context. It's great to see that both Ionet and OpenLedger are aligned on how LLMs and SLMs will shape the future.
Transition to Partnerships:
Taking a step back, let's talk about partnerships. Collaboration plays a huge role in advancing our missions, especially in the Web3 ecosystem.
Tausif, we’ve got a question here: How do partnerships help drive Ionet's mission? And what qualities do you look for in potential collaborators?
Tausif's Response:
Absolutely. Partnerships are essential to moving this space forward.
If you take a step back, Ionet and OpenLedger are working toward decentralizing the AI tech stack. Ionet focuses on distributed, globally accessible compute power, while OpenLedger builds trust-based, permissionless data platforms.
In the broader ecosystem, other players are experimenting with different layers—be it applications or business models—to bring decentralized AI to the mainstream.
To succeed, we need collaborations where the sum of the parts is greater than the whole. For example, the Ionet-OpenLedger partnership exemplifies how compute and data complement each other. Together, we can push the boundaries of what's possible.
From Ionet's side, we focus on the foundational compute layer. By partnering with innovators in other parts of the stack, we enable an entire ecosystem to flourish. Partnerships like these make decentralized AI a reality faster and more effectively.
Milan's Transition:
Thanks, Tausif. Ram, from OpenLedger’s perspective, how do you view partnerships? What do you prioritize when forming collaborations?
Ram's Response:
I completely agree with Tausif. However, I want to add that I don’t believe in "label partnerships," where companies collaborate for show without tangible results.
Our partnerships focus on integrating into our tech stack meaningfully. For example, OpenLedger collects data to train domain-specific models. Naturally, we need compute power to fine-tune these models.
When evaluating partners, we prioritize cost efficiency, decentralization, and ease of integration. Ionet was a natural fit. Their decentralized compute offering is cost-effective and aligns with our vision of democratizing AI.
Beyond compute, we collaborate with partners who improve our platform’s functionality and benefit the end-user experience.
Milan's Transition:
Thanks, Ram. That’s a great perspective on partnerships.
Now, let's dive into data attribution and community involvement.
Milan's Next Question:
The community has been asking about the importance of data attribution and how they can contribute. Gaurav, why is data attribution crucial, and how can the community get involved?
[続きます...]
Gaurav's Response:
People who have built models will find this obvious, but for those who haven't, it's worth understanding that the majority of effort in creating a model—about 70–80%—goes into sourcing, cleaning, and preparing the data.
The performance of a model is only as good as the data fed into it.
Centralized players like Google and Amazon already have products like Alexa and Gmail to collect massive amounts of data. They use this to train their models and create valuable services.
In the decentralized space, we don’t have such built-in systems for gathering data. This is where data attribution becomes critical.
We need to motivate individuals and organizations to provide clean, unbiased data. Attribution helps by ensuring that contributors are recognized for their work.
To encourage contributions, many crypto projects have started incentivizing data providers with tokens. This not only creates trust but also motivates the community to share valuable datasets.
That’s why attribution is essential, and rewarding contributors with recognition or incentives will help us build a robust decentralized ecosystem.
Milan's Transition:
Thank you, Gaurav. That’s a very clear explanation.
Tausif, from a broader business perspective, why is data attribution essential, and how can the community benefit from it?
Tausif's Response:
Absolutely. Data attribution is a key factor in driving mainstream adoption of AI, especially decentralized AI.
Let’s step back and consider how most people perceive AI today. Many fear that AI could replace their jobs or exploit their hard work without recognition.
By implementing a proper attribution system, we make people feel included in the AI development process.
For instance, just yesterday, OpenAI’s video model was leaked on Hugging Face by artists who claimed their work was used to train the model without consent.
This highlights the need for transparent attribution. If people see that their contributions are valued and compensated, they’re more likely to embrace AI and contribute to its growth.
Data attribution is not just about fairness—it’s about creating a sustainable ecosystem where contributors and users both benefit.
Milan's Transition:
Great points, Tausif. Ram, what’s OpenLedger’s take on data attribution? How do you ensure data quality and incentivize contributions?
Ram's Response:
As Gaurav and Tausifmentioned, data attribution is essential, especially in the decentralized AI ecosystem.
At OpenLedger, we ensure quality and attribution through two primary methods:
Technical Validation:
We use validation engines to assess the quality of incoming data. These engines are run by Eigenvalidators, who validate datasets without compromising privacy.Negative Incentives:
If someone contributes low-quality data, they won’t receive rewards or attribution. This discourages junk contributions and ensures that only high-quality data flows into the system.
Moreover, we record every data point on-chain, ensuring transparency. Contributors can see how their data is used, which models it influenced, and how they’re rewarded.
This creates a trustless, efficient system where contributors are motivated to provide valuable data.
Milan's Transition:
Thanks, Ram. You’ve touched on some really interesting points about transparency and trust.
Let’s shift gears slightly. Ram, earlier you mentioned the "three layers" of decentralized AI—data contribution, model building, and apps.
Could you expand on how these layers interact and benefit the broader ecosystem?
Ram's Response:
Sure. These three layers—data contribution, model building, and apps—are the backbone of decentralized AI.
Data Contribution:
This is the foundational layer where individuals or businesses provide datasets. On OpenLedger, every dataset is tracked on-chain, ensuring transparency and provenance. Contributors can see how their data influences models and how much revenue they earn.Model Building:
Once data is collected, it’s used to train domain-specific models. By understanding how data impacts inference accuracy, model builders can fine-tune their algorithms.Apps:
These are the end-user products that leverage the trained models. For example, a business could use a specialized model to power its AI-driven customer support.
By integrating these three layers, we enable a fully transparent and decentralized ecosystem. Data providers, model builders, and app developers all benefit, creating a win-win scenario for everyone involved.
Milan's Transition:
That’s a great breakdown, Ram.
Gaurav, how does Ionet’s approach to these layers differ from OpenLedger’s?
Gaurav's Response:
At Ionet, we’ve taken a slightly different approach. Instead of starting with data contribution, we began with compute infrastructure.
Here’s how we’re building out the three layers:
Apps (Top Layer):
Our first priority is enabling end-users to access powerful AI models for free. For example, we’re deploying pre-trained models on our GPU infrastructure and making them publicly available. This gives immediate value to the community.Model Building (Middle Layer):
In Phase 2 of our platform, users will be able to upload their datasets and train or fine-tune models. We’ll also provide tools to deploy and scale these models. For example, ML engineers won’t have to worry about setting up pipelines or scaling GPUs—we’ll handle it for them.Data Contribution (Bottom Layer):
Eventually, we’ll implement a decentralized system for data attribution and contribution, similar to OpenLedger’s approach. This will allow individuals and businesses to share datasets while maintaining full transparency.
We believe this phased approach—starting with apps, then models, and finally data—will create the most immediate impact while setting the stage for long-term growth.
Milan's Transition:
Thanks, Gaurav. That’s a fascinating strategy.
We’re nearing the end of the AMA, but we have time for a few quick community questions.
Community Question for Tausif:
What makes a collaboration exciting for you, and what do you look for in a successful partnership?
Tausif's Response:
Great question!
For me, an exciting collaboration happens when both parties complement each other’s strengths.
For example, Ionet focuses on compute, while OpenLedger specializes in data validation and attribution. Together, we’re tackling different layers of the decentralized AI stack, creating a more complete solution.
I also look for partners who are mission-aligned and passionate about what they’re building. When both teams are equally invested, the collaboration is more likely to succeed.
Community Question for Gaurav:
Will the community get opportunities to test AI models on Ionet’s platform?
Gaurav's Response:
Absolutely!
In the next few weeks, we’ll release a platform where users can access pre-trained AI models for free.
If you have your own models, Phase 2 of Ion Intelligence (launching in January) will allow you to deploy and test them on our GPUs.
We’ll even provide incentives for high-performing models, including GPU credits and token rewards. Stay tuned!
Community Question for Ram:
How is OpenLedger changing perceptions of data ownership and attribution?
Ram's Response:
OpenLedger is redefining data ownership by giving control back to the contributors.
We record every data point on-chain, ensuring transparency. Contributors know how their data is used and are rewarded accordingly.
This approach encourages businesses and individuals to share high-quality data, ultimately improving AI models and benefiting the broader ecosystem.
By decentralizing AI, we’re building a future where everyone—not just big tech—can participate and benefit.
Milan's Closing:
Thank you, Gaurav, Tausif, and Ram, for an incredibly insightful discussion.
And thank you to everyone who joined today’s AMA. Don’t forget to post your screenshots in the OpenLedger Discord for a chance to win prizes!
We’re excited about the future of decentralized AI and look forward to collaborating further.
Have a great day, everyone!